Gibbs sampler for Bayesian prediction of triple seasonal autoregressive processes
COMMUNICATIONS IN STATISTICS-THEORY AND METHODS(2024)
摘要
Researchers have extended autoregressive (AR) time-series models to adequately fit and model time-series with triple seasonality. These AR extensions can be referred to as triple seasonal AR (TSAR) models. For these TSAR time-series models, only Bayesian estimation and identification have been introduced. Therefore, in this article, we aim to extend the existing work for presenting the Bayesian prediction for TSAR models using the Gibbs sampler algorithm. In this Bayesian prediction, we first assume the TSAR errors are identically normally distributed, and we employ normal-gamma and g priors for the TSAR parameters. Based on the normally distributed TSAR errors and the specified TSAR parameters' priors, we are able to derive the conditional predictive and posterior densities. Particularly, we show the conditional posterior densities of TSAR coefficients and variance are the multivariate normal and inverse-gamma, respectively. In addition, for the future TSAR observations, we show the conditional predictive density is the multivariate normal. Using these derived full conditional distributions, we propose the Gibbs sampler to efficiently approximate the joint predictive and posterior densities and to easily carry out multiple-step ahead predictions. We validate the accuracy of forecasting for the proposed Gibbs sampler by conducting a simulation study. Moreover, we apply the proposed Gibbs sampler to hourly electricity-load time-series datasets in some European countries, along with a comparison with the well-known long-short term memory (LSTM) recurrent neural model.
更多查看译文
关键词
Triple seasonality,TSAR models,predictive analysis,Gibbs sampler,LSTM model,hourly electricity-load
AI 理解论文
溯源树
样例
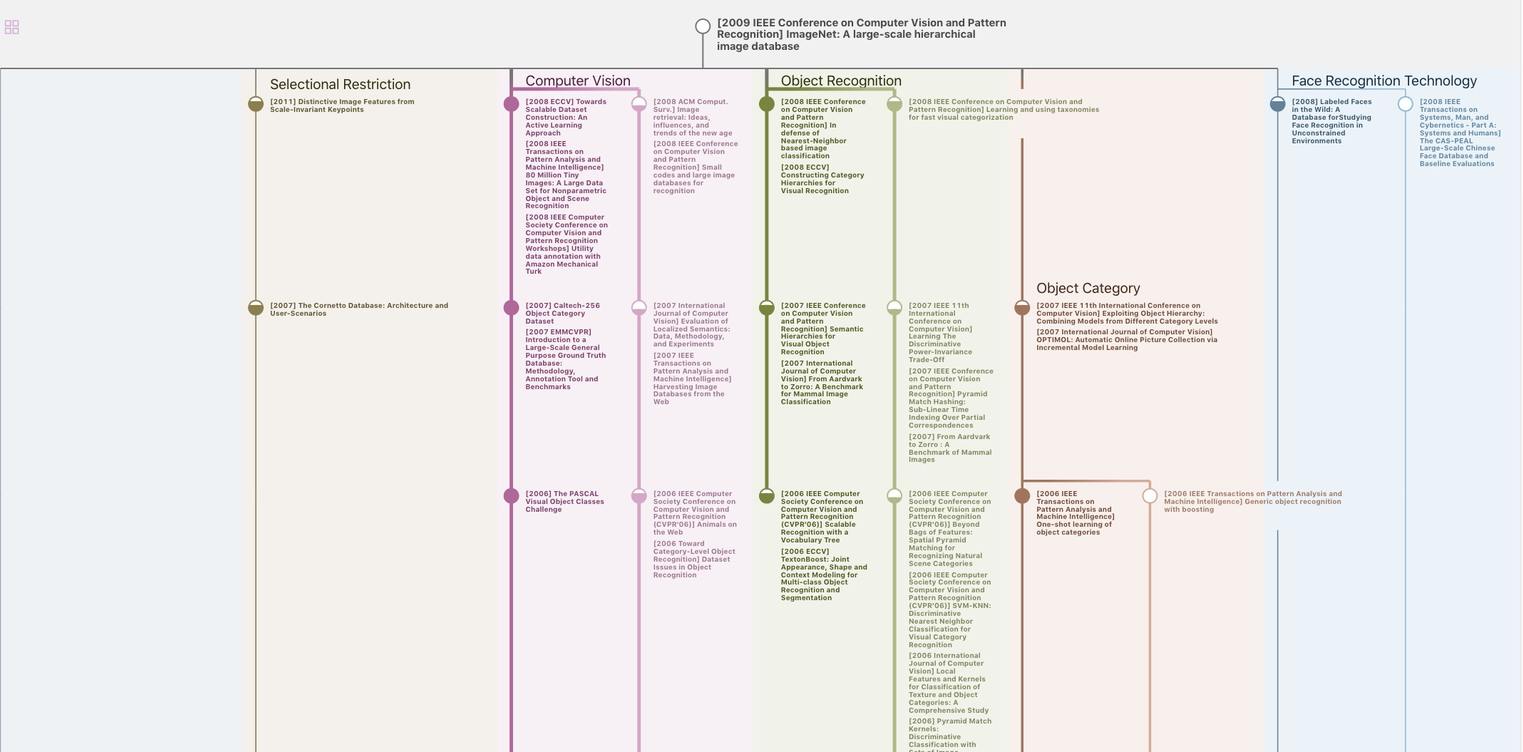
生成溯源树,研究论文发展脉络
Chat Paper
正在生成论文摘要