A deep learning model for translating CT to ventilation imaging: analysis of accuracy and impact on functional avoidance radiotherapy planning
Japanese Journal of Radiology(2024)
摘要
Radiotherapy planning incorporating functional lung images has the potential to reduce pulmonary toxicity. Free-breathing 4DCT-derived ventilation image (CTVI) may help quantify lung function. This study introduces a novel deep-learning model directly translating planning CT images into CTVI. We investigated the accuracy of generated images and the impact on functional avoidance planning. Paired planning CT and 4DCT scans from 48 patients with NSCLC were randomized to training (n = 41) and testing (n = 7) data sets. The ventilation maps were generated from 4DCT using a Jacobian-based algorithm to provide ground truth labels (CTVI4DCT). A 3D U-Net-based model was trained to map CT to synthetic CTVI (CTVISyn) and validated using fivefold cross-validation. The highest-performing model was applied to the testing set. Spearman's correlation (rs) and Dice similarity coefficient (DSC) determined voxel-wise and functional-wise concordance between CTVI4DCT and CTVISyn. Three plans were designed per patient in the testing set: one clinical plan without CTVI and two functional avoidance plans combined with CTVI4DCT or CTVISyn, aimed at sparing high-functional lungs defined as the top 50
更多查看译文
关键词
Deep learning,4DCT,Synthetic imaging,Ventilation imaging,Radiotherapy,Lung cancer
AI 理解论文
溯源树
样例
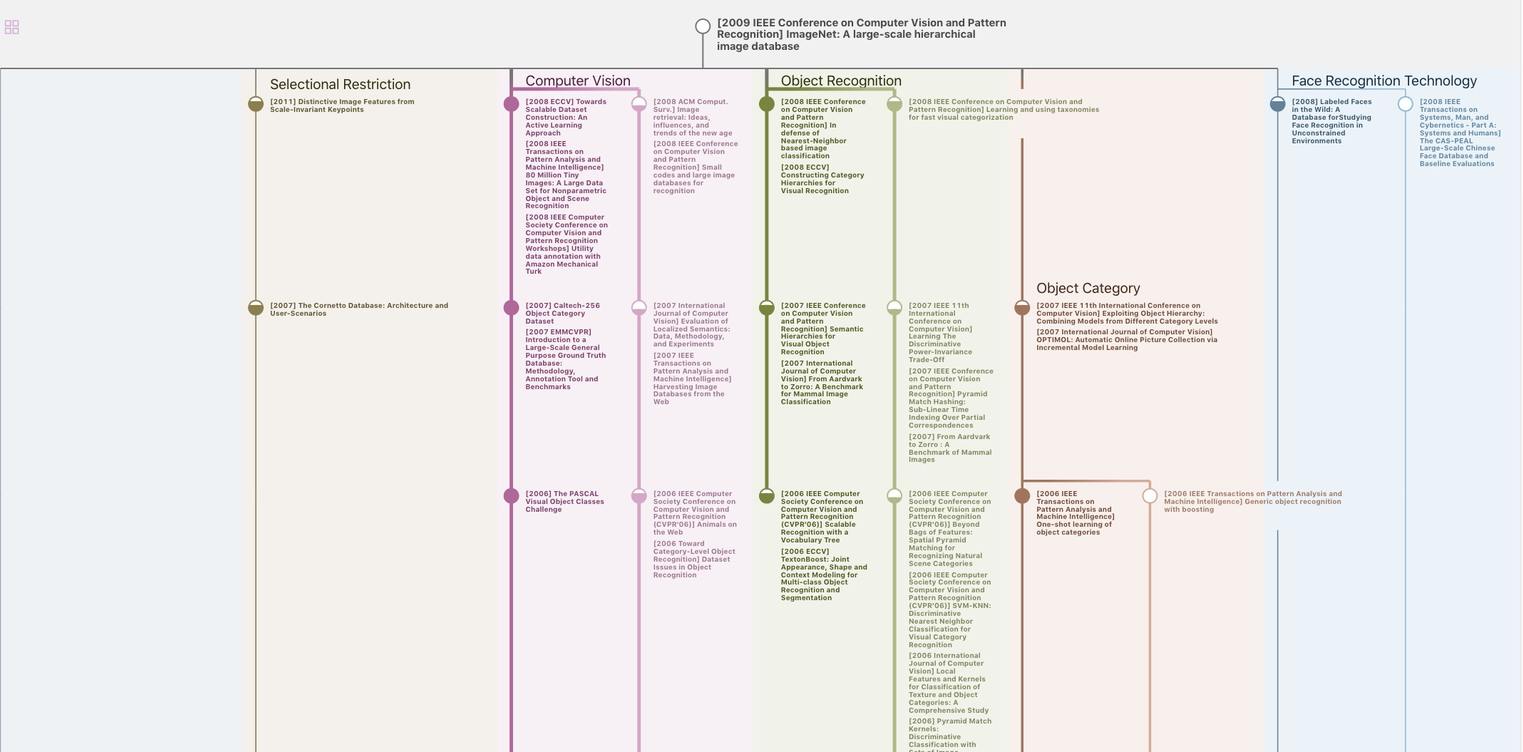
生成溯源树,研究论文发展脉络
Chat Paper
正在生成论文摘要