Cross-domain Low-dose CT Image Denoising with Semantic Preservation and Noise Alignment
IEEE Transactions on Multimedia(2024)
摘要
Deep learning (DL)-based Low-dose CT (LDCT) image denoising methods may face domain shift problem, where data from different domains (i.e., hospitals) may have similar anatomical regions but exhibit different intrinsic noise characteristics. Therefore, we propose a plug-and-play model called Lowand High-frequency Alignment (LHFA) to address this issue by leveraging semantic features and aligning noise distributions of different CT datasets, while maintaining diagnostic image quality and suppressing noise. Specifically, the LHFA model consists of a Low-frequency Alignment (LFA) module that preserves semantic features (i.e., low-frequency components) with fewer perturbations from both domains for reconstruction. Notably, a Highfrequency Alignment (HFA) module is proposed to quantify the discrepancy between noise representations (i.e., high-frequency components) in a latent space mapped by an auto-encoder. Experimental results demonstrate that the LHFA model effectively alleviates the domain shift problem and significantly improves the performance of DL-based methods on cross-domain LDCT image denoising task, outperforming other domain adaptationbased methods.
更多查看译文
关键词
Image denoising,deep learning,domain adaptation,low-dose CT image
AI 理解论文
溯源树
样例
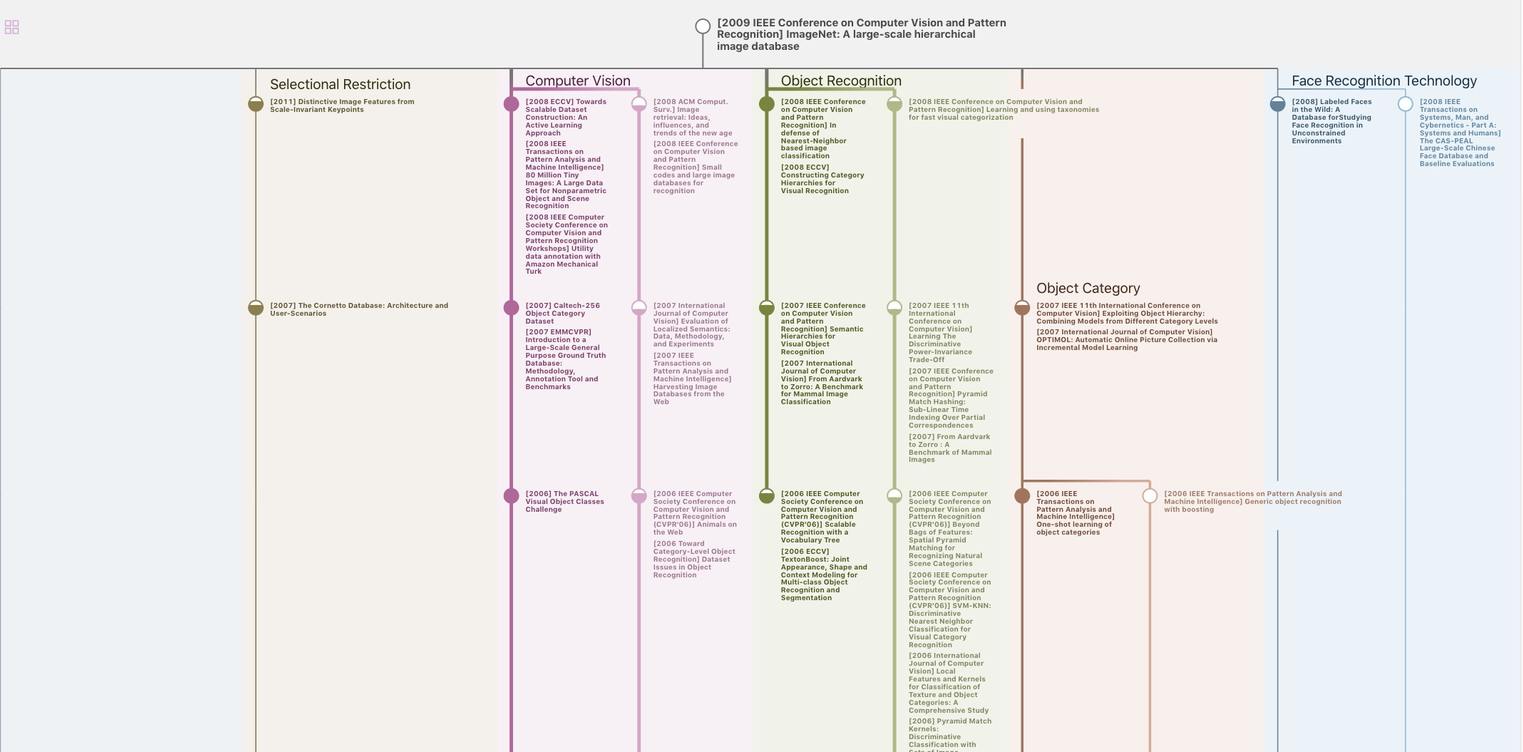
生成溯源树,研究论文发展脉络
Chat Paper
正在生成论文摘要