Centralized and Federated Learning for COVID-19 Detection With Chest X-Ray Images: Implementations and Analysis
IEEE TRANSACTIONS ON EMERGING TOPICS IN COMPUTATIONAL INTELLIGENCE(2024)
摘要
In the health domain, due to privacy issues, many important datasets are isolated, which nonetheless need to be analyzed collaboratively for conclusions to be drawn efficiently. To maintain data privacy, federated learning (FL) trains a communal model from scattered datasets without centralized data integration. In this paper, we compare and analyze the performance of traditional deep learning (DL) and FL techniques using the chest X-Ray (CXR) image dataset for COVID-19 detection. We first implemented DL techniques VGG-16, ResNet50, and Inceptionv3, where ResNet50 is found to be best on the classification task with 98% accuracy. We then proposed FL implementations - federated averaging and federated learning using ResNet50 for training local and global models. The proposed FL converges faster and outperforms the base FL for both independent and identically distributed (IID) and non-IID datasets. While the FL handles bigger data efficiently, compared to DL, it compromised 3.56% in accuracy to preserve privacy. Our results provide a platform for the further investigation of FL in COVID-19 detection.
更多查看译文
关键词
COVID-19,Residual neural networks,Data models,Training,X-rays,Servers,Privacy,Chest X-ray,deep learning,federated learning,heterogeneity,privacy-preserving
AI 理解论文
溯源树
样例
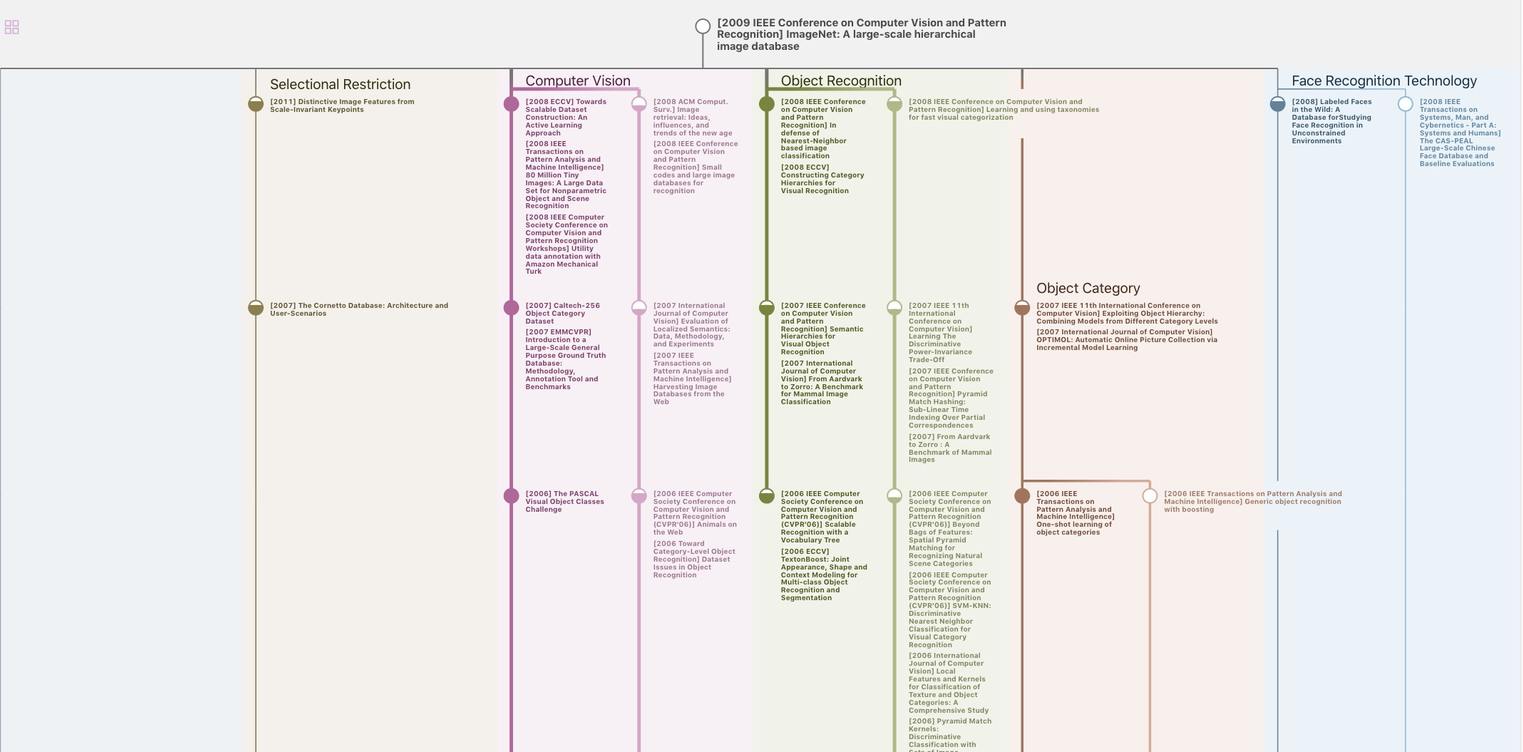
生成溯源树,研究论文发展脉络
Chat Paper
正在生成论文摘要