OCI-SSL: Open Class-Imbalanced Semi-Supervised Learning With Contrastive Learning
IEEE Transactions on Emerging Topics in Computational Intelligence(2024)
摘要
Semi-supervised learning (SSL) is a powerful technique that leverages unlabeled data to improve model performance. Conventional SSL algorithms generally make the assumption that the unlabeled data are derived from approximately balanced known classes. However, in real-world scenarios, the unlabeled data may come from imbalanced known classes and out-of-distribution (OOD) unknown classes, which significantly impacts the performance of SSL algorithms. In this study, a more realistic framework for open class-imbalanced semi-supervised learning (OCI-SSL) is presented to address the challenges posed by imbalanced class distribution and OOD novel classes. To alleviate the adverse effects caused by the OOD data, an improved one-vs-all classifier that incorporates the strategies of hard-negative sampling and Bernoulli sampling is proposed to identify OOD samples. Then an auxiliary balancing classifier is designed to improve both the supervised loss of labeled data and the consistency regularization loss of unlabeled data in the presence of class imbalance by introducing a mask of rebalancing class distribution. Moreover, a weight-parameterized semi-supervised contrastive learning method is developed to enhance feature learning for all in-distribution data. Extensive experiments demonstrate that our method outperforms state-of-the-art methods and achieves an average accuracy improvement of 11.7% and AUROC improvement of 50.3% on CIFAR-10.
更多查看译文
关键词
Class-imbalanced learning,contrastive learning,open-set,rebalance,semi-supervised learning (SSL)
AI 理解论文
溯源树
样例
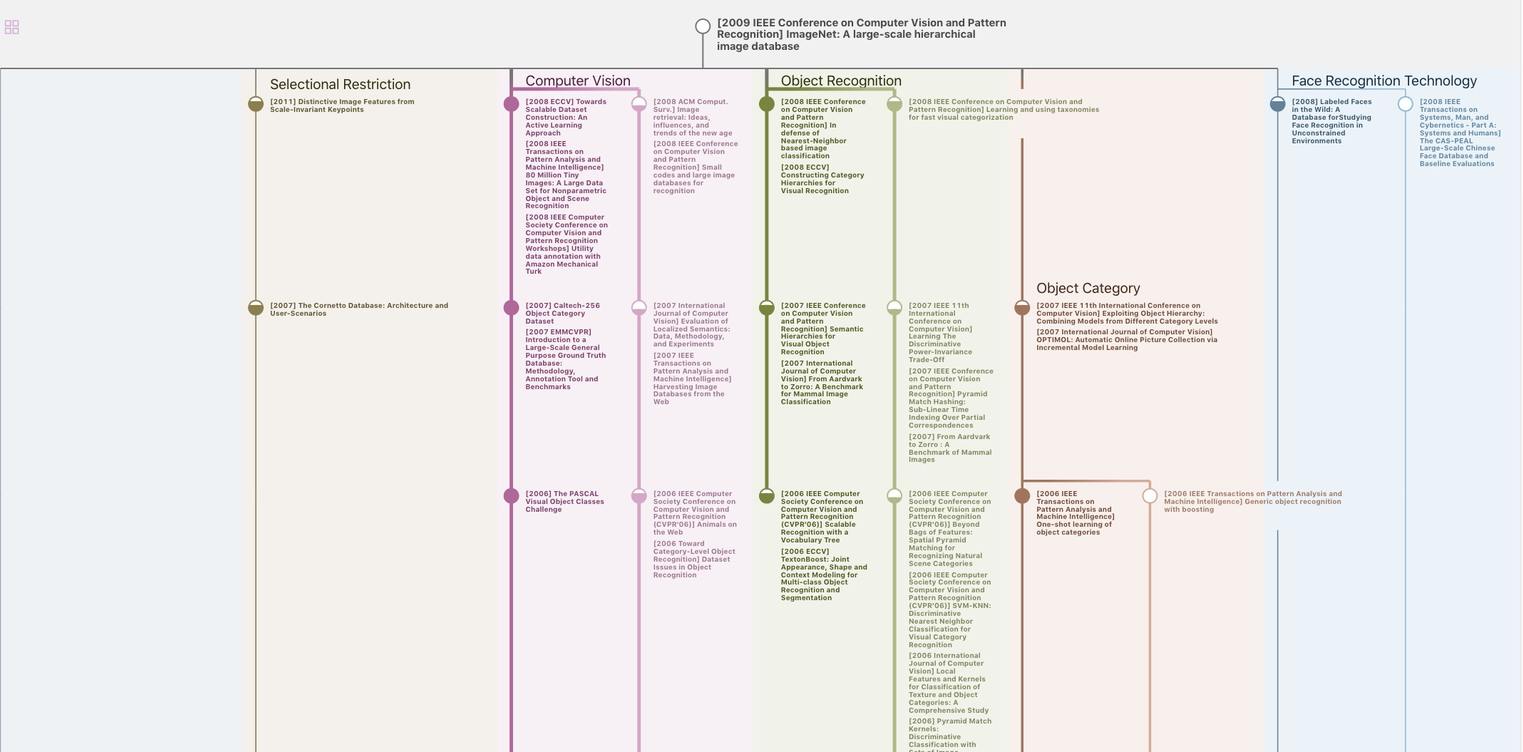
生成溯源树,研究论文发展脉络
Chat Paper
正在生成论文摘要