Application of Randomness for Security and Privacy in Multi-Party Computation
IEEE Transactions on Dependable and Secure Computing(2024)
摘要
A secure Multi-Party Computation (MPC) is one of the distributed computational methods, where it computes a function over the inputs given by more than one party jointly and keeps those inputs private from the parties involved in the process. Randomization in secret sharing leading to MPC is a requirement for privacy enhancements; however, most of the available MPC models use the trust assumptions of sharing and combining values. Thus, randomization in secret sharing and MPC modules is neglected. As a result, the available MPC models are prone to information leakage problems, where the models can reveal the partial values of the sharing secrets. In this paper, we propose the first model of utilizing a random function generator as an MPC primitive. More specifically, we analyze our previous development of the Symmetric Random Function Generator (SRFG) for information-theoretic security, where the system is considered to have unconditional security if it is secure against adversaries with unlimited computing resources and time. Further, we apply SRFG to eradicate the problem of information leakage in the general MPC model. Through a set of experiments, we show that SRFG is a function generator that can generate the combined functions (combination of logic GATEs) with
$n/2$
-private to
$n$
-private norms. As the main goal of MPC is privacy preservation of the inputs, we analyze the applicability of SRFG properties in secret sharing and MPC and observe that SRFG is eligible to be a cryptographic primitive in MPC developments. We also measure the performance of our proposed SRFG-based MPC framework with the other randomness generation-based MPC frameworks and analyze the comparative attributes with the state-of-the-art models. We observe that our posed SRFG-based MPC is
$\approx$
30% better in terms of throughput and also shows 100% privacy attainment.
更多查看译文
关键词
Cryptography,Privacy,Security,Information,Randomness,Function,Computation,Multi-party
AI 理解论文
溯源树
样例
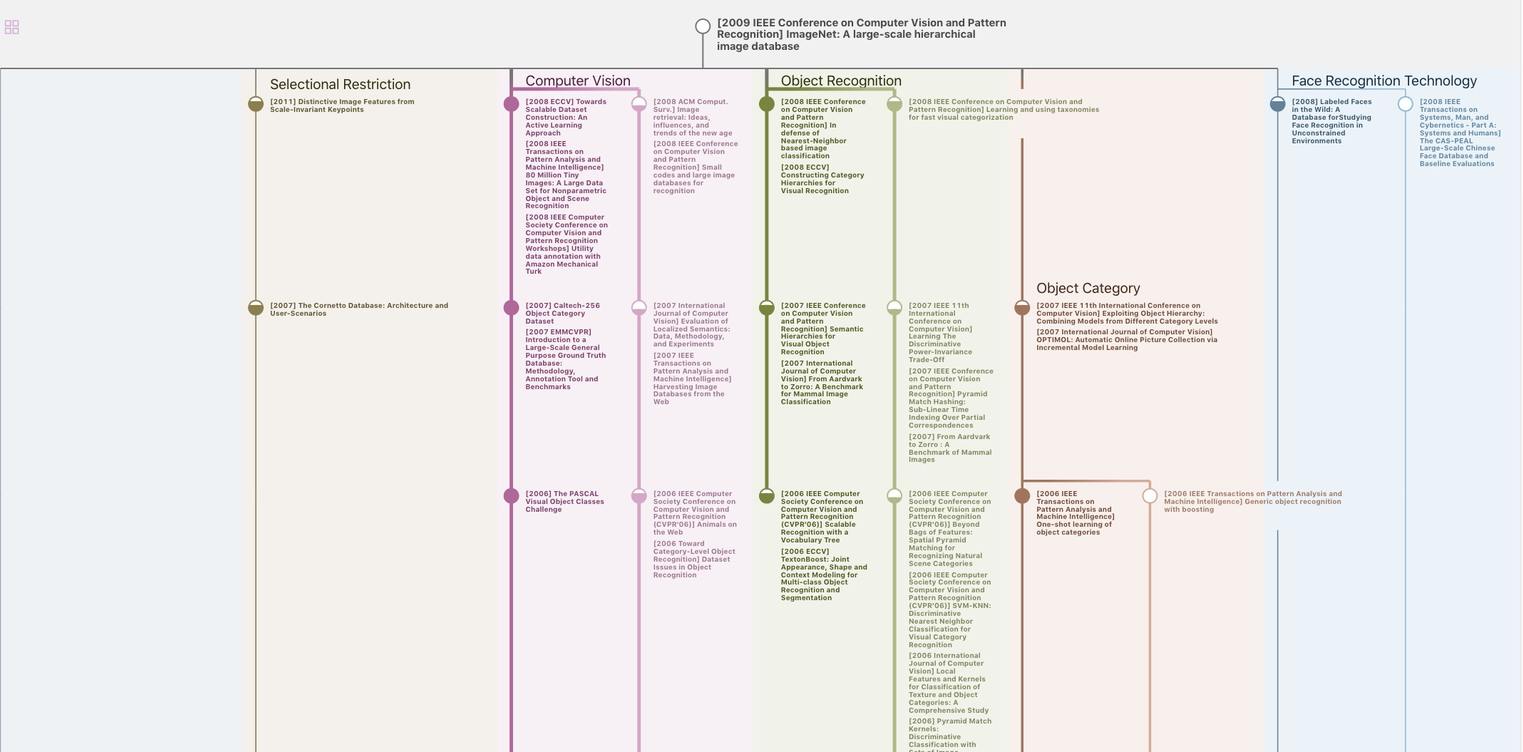
生成溯源树,研究论文发展脉络
Chat Paper
正在生成论文摘要