Universal Transfer Framework for Urban Spatio-Temporal Knowledge Based on Radial Basis Function
IEEE Transactions on Artificial Intelligence(2024)
摘要
The accurate and rapid transfer of complex urban spatio-temporal data is crucial for urban computing tasks such as urban planning and public transportation deployment for smart-city applications. Existing works consider auxiliary data or propose end-to-end models to process complex spatio-temporal information into more complex deep features. However, the latter is incapable of decoupling spatio-temporal knowledge, which means these end-to-end models lack modularity and substitutability. A general modular framework that can automatically capture simple representations of complex spatio-temporal information is required. In this paper, we thus propose a universal framework for the transfer of spatio-temporal knowledge based on a radial basis function (RBF). We termed this approach STRBF-TF. The proposed STRBF-TF generates simple RBF representations of spatio-temporal flow distribution with an RBF transfer block and also leverages a channel attention mechanism. Moreover, we propose two RBF kernel initializers suitable for the source and the target domains, respectively. The framework retains important spatio-temporal knowledge in simple representations for the reconfiguration of spatio-temporal feature distribution for fast and accurate transfer. We conducted cross-domain learning experiments on a large real-world telecom dataset. The results demonstrate the efficiency and accuracy of the proposed approach, as well as its suitability for real-world applications.
更多查看译文
关键词
Artificial intelligence in smart cities,Knowledge transfer,Transfer learning,Machine learning
AI 理解论文
溯源树
样例
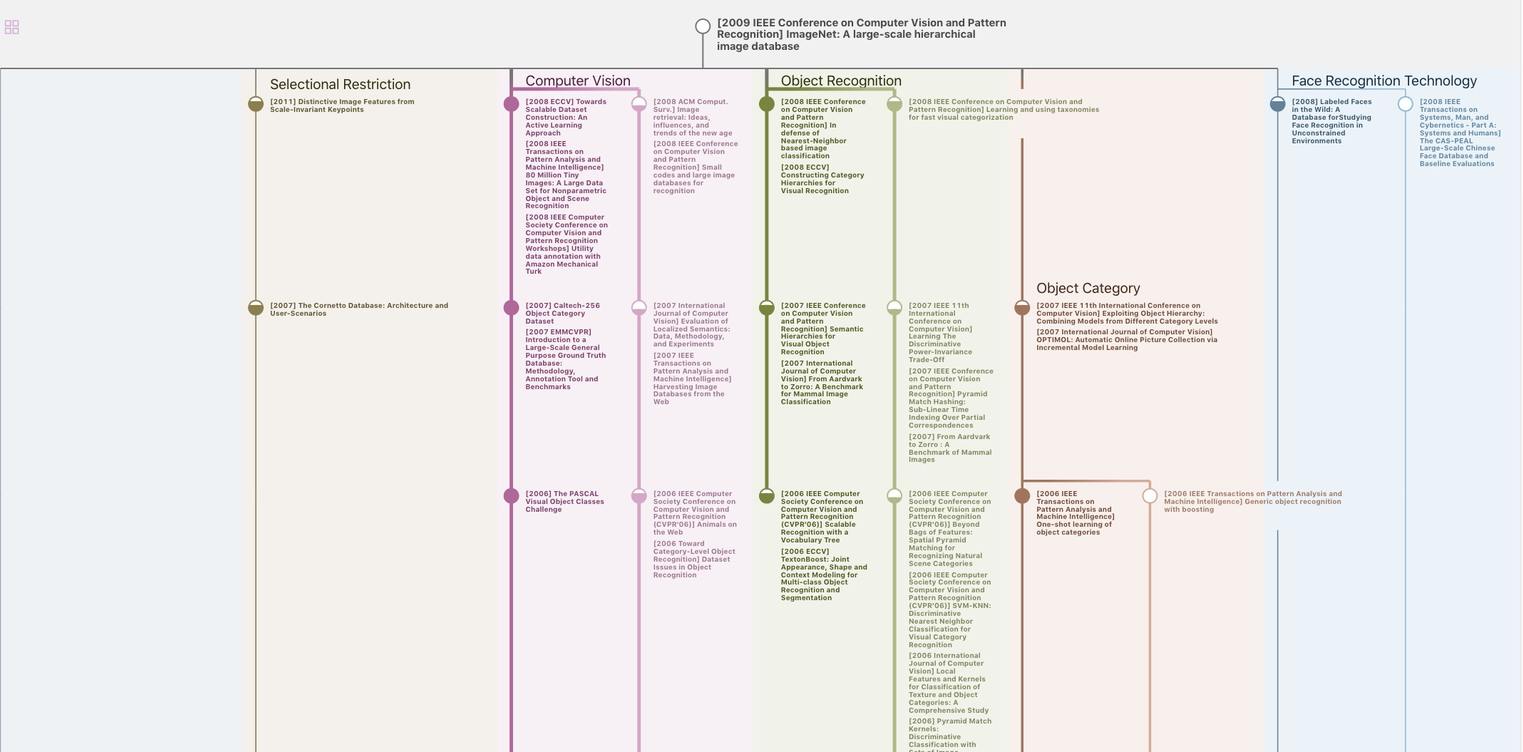
生成溯源树,研究论文发展脉络
Chat Paper
正在生成论文摘要