SQIX: QMIX Algorithm Activated by General Softmax Operator for Cooperative Multiagent Reinforcement Learning
IEEE Transactions on Systems, Man, and Cybernetics: Systems(2024)
摘要
Multiagent cooperative systems can be used to conceptualize many real-world problems. Reinforcement learning is a particularly effective tool. The issue of bias in
$Q$
-function value estimation in single-agent reinforcement learning has garnered a lot of interest and substantial study. Indeed, this challenge endures in multiagent reinforcement learning, primarily owing to the inclusion of maximization operations. The crux of the matter lies in the inability to seamlessly extrapolate single-agent reinforcement learning algorithms to their multiagent counterparts. In this article, we introduce a more encompassing and straightforward principle: the notion of appropriate value correction. We suggest replacing the maximization operation with a monotonically nondecreasing function to obtain more accurate value estimates. We theoretically demonstrate that this operation effectively reduces the potential overestimation bias in the QMIX algorithm. Ultimately, our methodology, dubbed the SMIX algorithm—a fusion of the QMIX algorithm empowered by the Softmax operator, attains state-of-the-art outcomes across diverse multiagent cooperative tasks. This success extends to challenging domains such as StarCraft II, marking it as one of the most formidable games to date.
更多查看译文
关键词
Cooperative multiagent system,multiagent deep reinforcement learning,softmax operator,StarCraft,value estimation
AI 理解论文
溯源树
样例
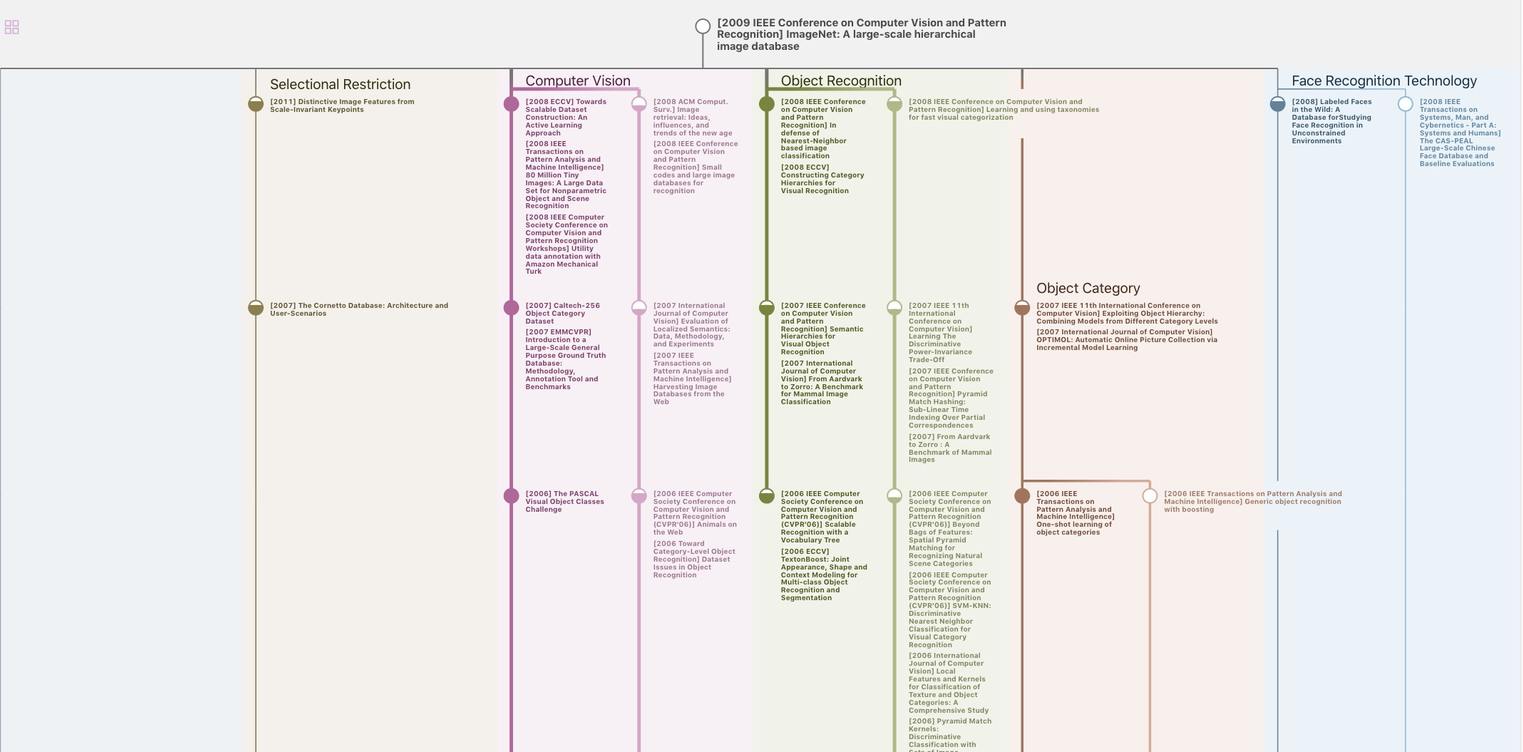
生成溯源树,研究论文发展脉络
Chat Paper
正在生成论文摘要