BAM: Box Abstraction Monitors for Real-time OoD Detection in Object Detection
arxiv(2024)
摘要
Out-of-distribution (OoD) detection techniques for deep neural networks
(DNNs) become crucial thanks to their filtering of abnormal inputs, especially
when DNNs are used in safety-critical applications and interact with an open
and dynamic environment. Nevertheless, integrating OoD detection into
state-of-the-art (SOTA) object detection DNNs poses significant challenges,
partly due to the complexity introduced by the SOTA OoD construction methods,
which require the modification of DNN architecture and the introduction of
complex loss functions. This paper proposes a simple, yet surprisingly
effective, method that requires neither retraining nor architectural change in
object detection DNN, called Box Abstraction-based Monitors (BAM). The novelty
of BAM stems from using a finite union of convex box abstractions to capture
the learned features of objects for in-distribution (ID) data, and an important
observation that features from OoD data are more likely to fall outside of
these boxes. The union of convex regions within the feature space allows the
formation of non-convex and interpretable decision boundaries, overcoming the
limitations of VOS-like detectors without sacrificing real-time performance.
Experiments integrating BAM into Faster R-CNN-based object detection DNNs
demonstrate a considerably improved performance against SOTA OoD detection
techniques.
更多查看译文
AI 理解论文
溯源树
样例
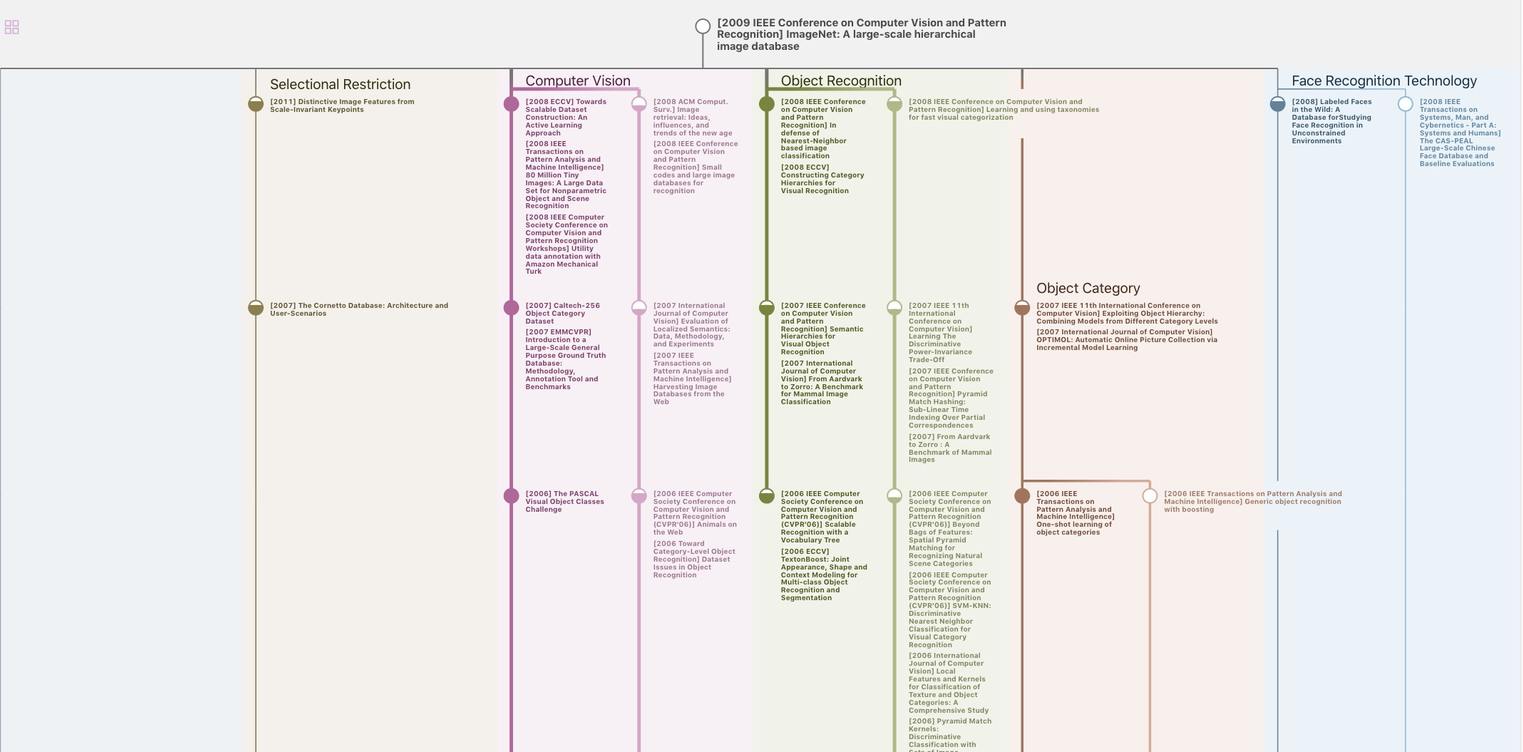
生成溯源树,研究论文发展脉络
Chat Paper
正在生成论文摘要