Common Sense Enhanced Knowledge-based Recommendation with Large Language Model
arxiv(2024)
摘要
Knowledge-based recommendation models effectively alleviate the data sparsity
issue leveraging the side information in the knowledge graph, and have achieved
considerable performance. Nevertheless, the knowledge graphs used in previous
work, namely metadata-based knowledge graphs, are usually constructed based on
the attributes of items and co-occurring relations (e.g., also buy), in which
the former provides limited information and the latter relies on sufficient
interaction data and still suffers from cold start issue. Common sense, as a
form of knowledge with generality and universality, can be used as a supplement
to the metadata-based knowledge graph and provides a new perspective for
modeling users' preferences. Recently, benefiting from the emergent world
knowledge of the large language model, efficient acquisition of common sense
has become possible. In this paper, we propose a novel knowledge-based
recommendation framework incorporating common sense, CSRec, which can be
flexibly coupled to existing knowledge-based methods. Considering the challenge
of the knowledge gap between the common sense-based knowledge graph and
metadata-based knowledge graph, we propose a knowledge fusion approach based on
mutual information maximization theory. Experimental results on public datasets
demonstrate that our approach significantly improves the performance of
existing knowledge-based recommendation models.
更多查看译文
AI 理解论文
溯源树
样例
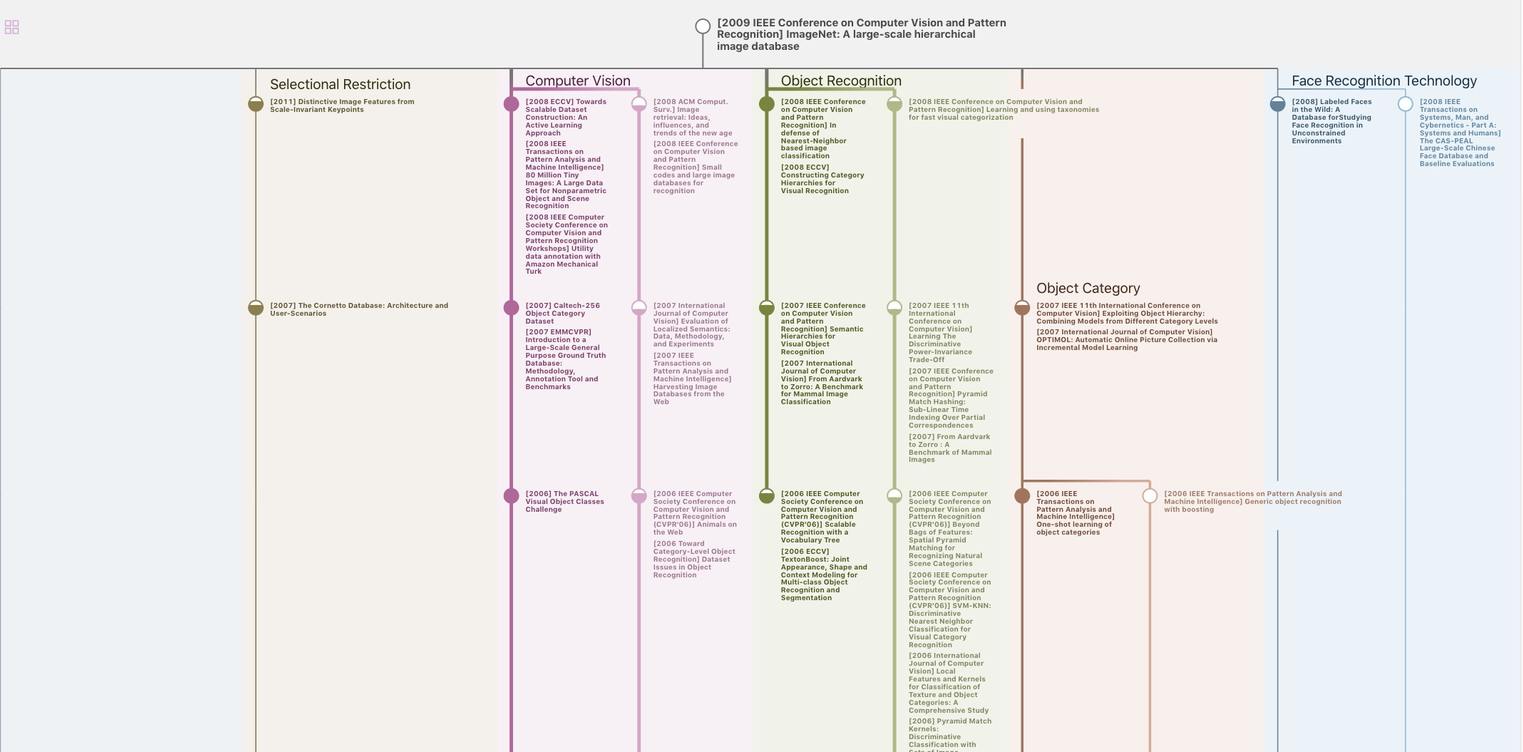
生成溯源树,研究论文发展脉络
Chat Paper
正在生成论文摘要