Physical data-driven modeling of deformation mechanism constraints on earth-rock dams based on deep feature knowledge distillation and finite element method
Engineering Structures(2024)
摘要
Constructing an interpretable model for the long-term deformation Structural Health Monitoring (SHM) of earth-rock dams is of great significance for improving the safety state evaluation and monitoring effect. In this paper, a physics-data-driven model for the deformation SHM of earth-rock dams is proposed based on deep mechanism knowledge distillation. Firstly, the deterministic model is established based on the Finite Element Model (FEM) and outputs the hydraulic load component curve and aging component curve. Then a regression prediction model (HTSGAN) between influencing factors and deformation measurements at multiple measurement points is established based on the Graph Convolutional Network (GCN) and attention mechanism. Finally, the Teacher-Hydraulic-Time-Seepage Graph Attention Networks (T-HTSGAN) model is established based on the feature-based multi-teacher knowledge distillation using the knowledge of hydraulic loading physics and soil-rock creep physics of the FEM for mechanism constraints. The model effectively solves the problems of poor model interpretability and lack of physics knowledge constraints in previous earth-rock dam SHM models. The research results are applied to a project of a 185.5-meter-high concrete-faced rockfill dam, and the predictive performance of the model is more effective and stable through the comparison of six baseline models. The comparative analysis of the component curves proves the effectiveness of the proposed knowledge distillation method for mechanism constraints and improves the interpretability of the neural network model. Therefore, the model is more suitable for engineering applications.
更多查看译文
关键词
Dam safety monitoring,Deep learning,Graph convolutional network,Knowledge distillation,Attention mechanism
AI 理解论文
溯源树
样例
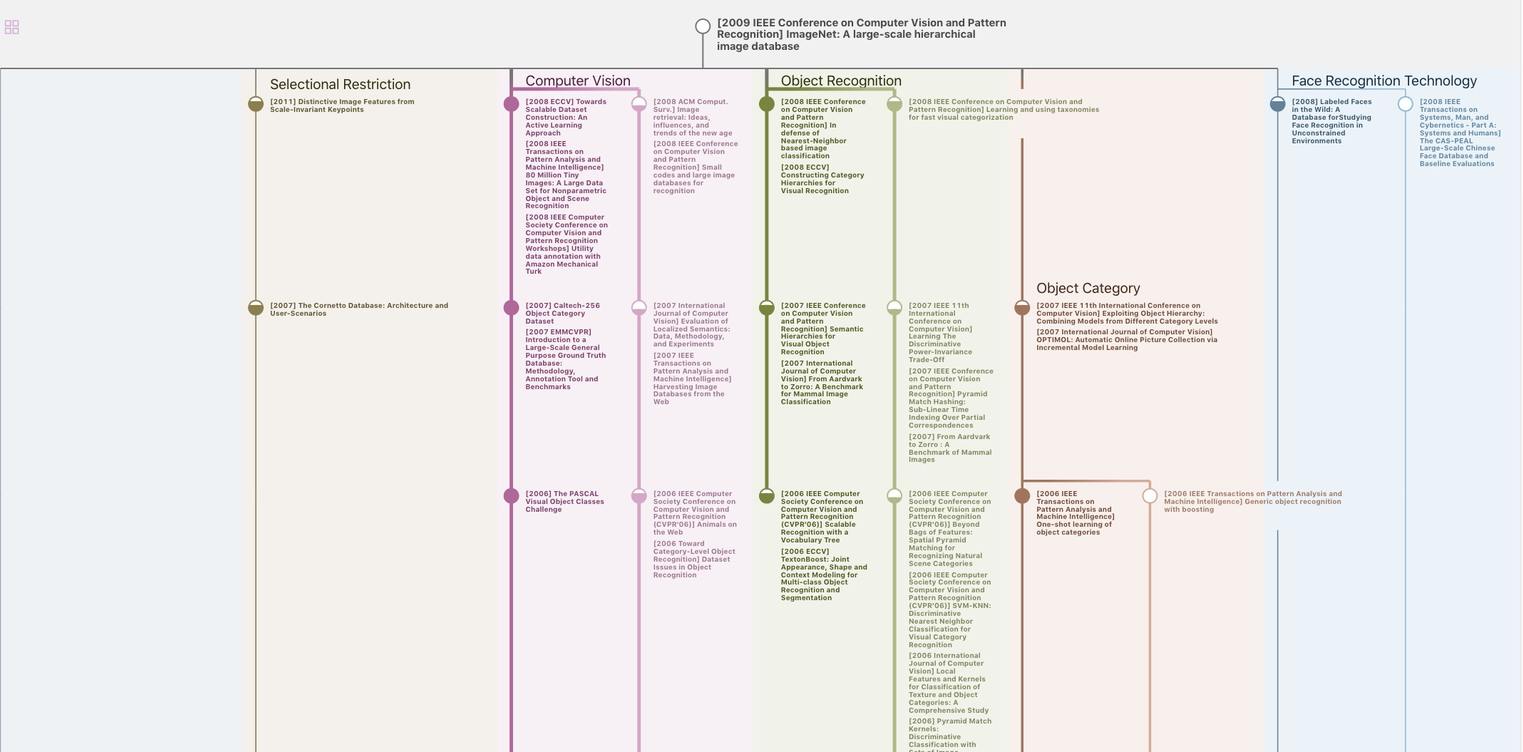
生成溯源树,研究论文发展脉络
Chat Paper
正在生成论文摘要