Advanced Learning Technique Based on Feature Differences of Moving Intervals for Detecting DC Series Arc Failures
Machines(2024)
摘要
DC microgrids are vital for integrating renewable energy sources into the grid, but they face the threat of DC arc faults, which can lead to malfunctions and fire hazards. Therefore, ensuring the secure and efficient operation of DC systems necessitates a comprehensive understanding of the characteristics of DC arc faults and the implementation of a reliable arc fault detection technique. Existing arc-fault detection methods often rely on time–frequency domain features and machine learning algorithms. In this study, we propose an advanced detection technique that utilizes a novel approach based on feature differences between moving intervals and advanced learning techniques (ALTs). The proposed method employs a unique approach by utilizing a time signal derived from power supply-side signals as a reference input. To operationalize the proposed method, a meticulous feature extraction process is employed on each dataset. Notably, the difference between features within distinct moving intervals is calculated, forming a set of differentials that encapsulate critical information about the evolving arc-fault conditions. These differentials are then channeled as inputs for advanced learning techniques, enhancing the model’s ability to discern intricate patterns indicative of DC arc faults. The results demonstrate the effectiveness and consistency of our approach across various scenarios, validating its potential to improve fault detection in DC systems.
更多查看译文
关键词
moving intervals,differential features,advanced learning techniques
AI 理解论文
溯源树
样例
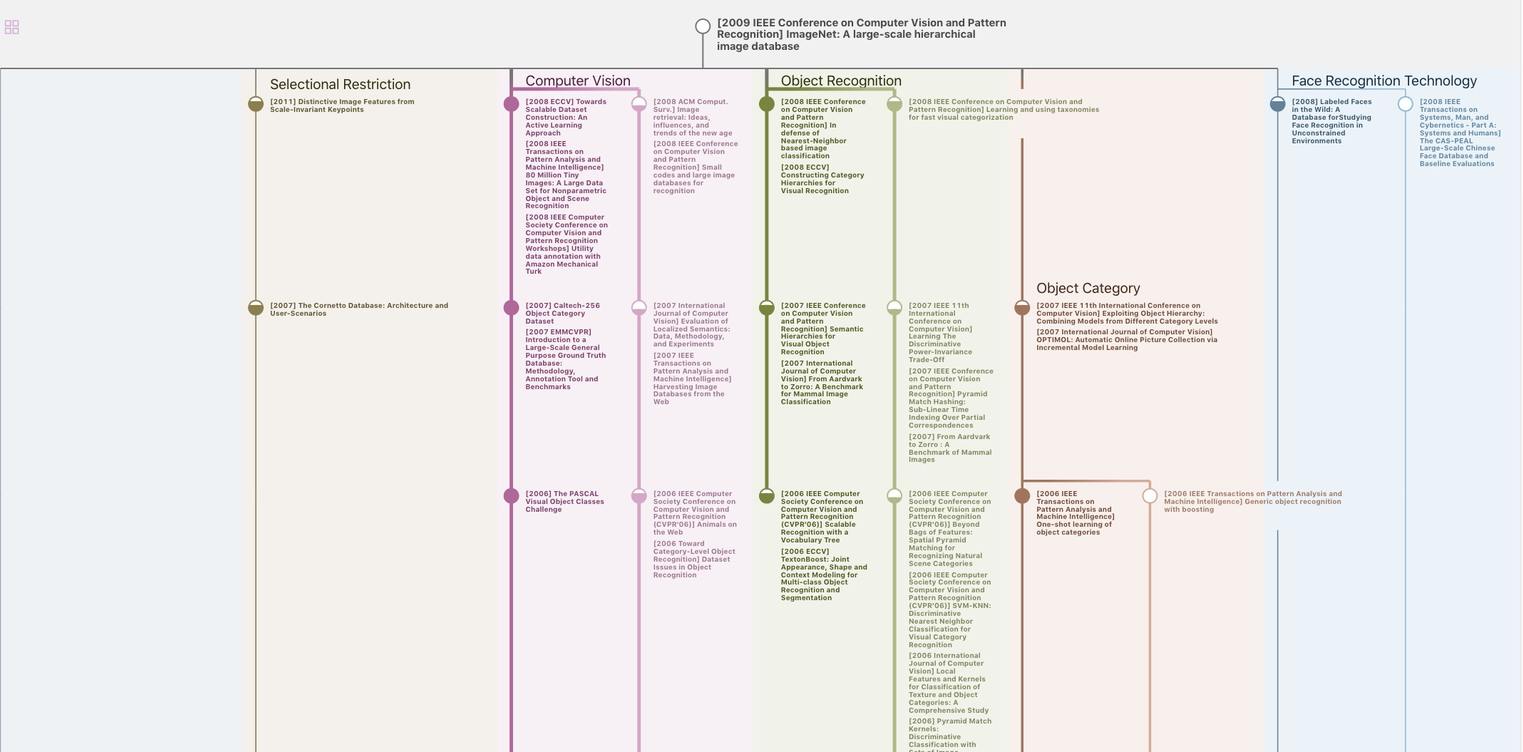
生成溯源树,研究论文发展脉络
Chat Paper
正在生成论文摘要