High-Throughput Ensemble-Learning-Driven Band Gap Prediction of Double Perovskites Solar Cells Absorber
MACHINE LEARNING AND KNOWLEDGE EXTRACTION(2024)
摘要
Perovskite materials have attracted much attention in recent years due to their high performance, especially in the field of photovoltaics. However, the dark side of these materials is their poor stability, which poses a huge challenge to their practical applications. Double perovskite compounds, on the other hand, can show more stability as a result of their specific structure. One of the key properties of both perovskite and double perovskite is their tunable band gap, which can be determined using different techniques. Density functional theory (DFT), for instance, offers the potential to intelligently direct experimental investigation activities and predict various properties, including band gap. In reality, however, it is still difficult to anticipate the energy band gap from first principles, and accurate results often require more expensive methods such as hybrid functional or GW methods. In this paper, we present our development of high-throughput supervised ensemble learning-based methods: random forest, XGBoost, and Light GBM using a database of 1306 double perovskites materials to predict the energy band gap. Based on elemental properties, characteristics have been vectorized from chemical compositions. Our findings demonstrate the efficiency of ensemble learning methods and imply that scientists would benefit from recently employed methods in materials informatics.
更多查看译文
关键词
double perovskite,ensemble learning,band gap,Bayesian optimization,materials informatics
AI 理解论文
溯源树
样例
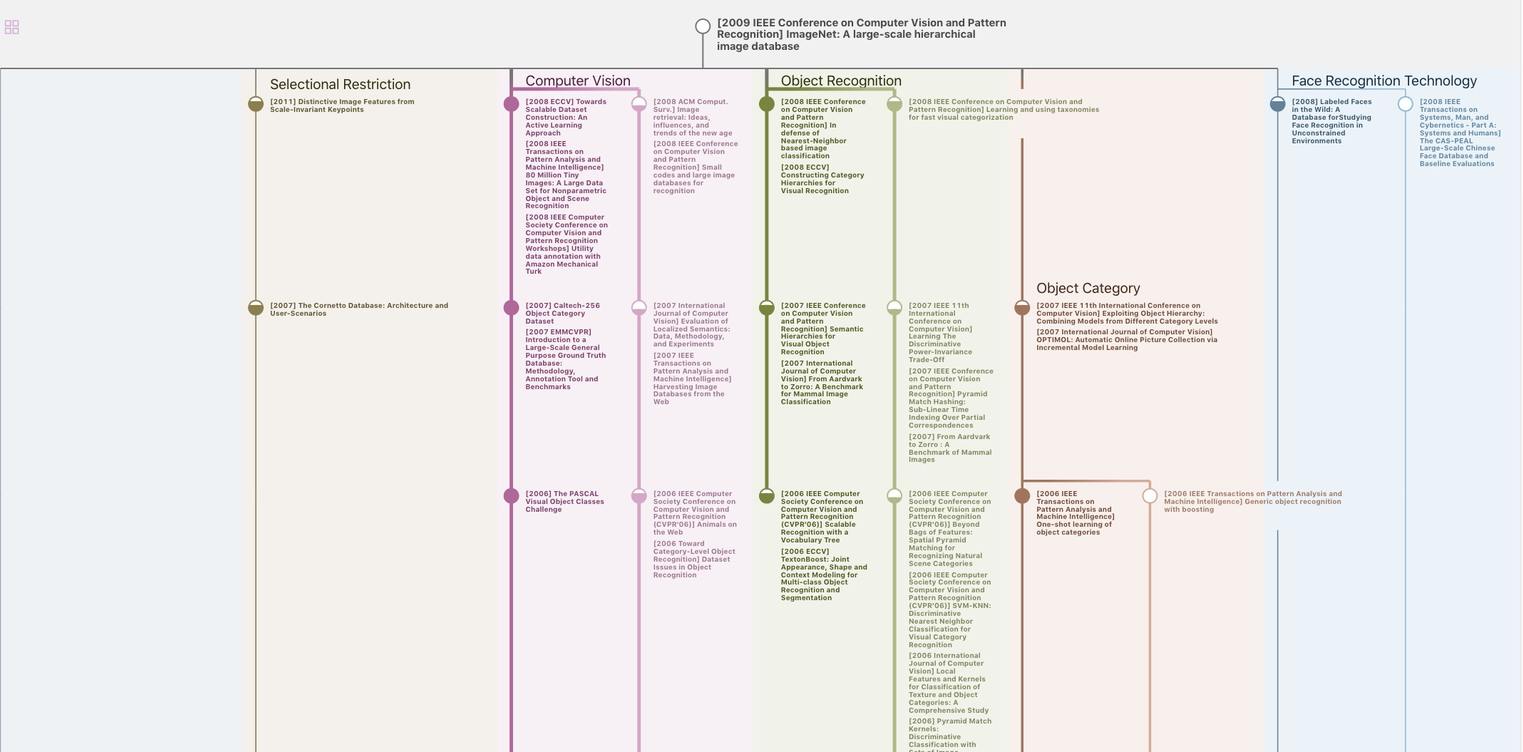
生成溯源树,研究论文发展脉络
Chat Paper
正在生成论文摘要