Vertical Metabolome Transfer from Mother to Child: An Explainable Machine Learning Method for Detecting Metabolomic Heritability
Metabolites(2024)
摘要
Vertical transmission of metabolic constituents from mother to child contributes to the manifestation of disease phenotypes in early life. This study probes the vertical transmission of metabolites from mothers to offspring by utilizing machine learning techniques to differentiate between true mother–child dyads and randomly paired non-dyads. Employing random forests (RF), light gradient boosting machine (LGBM), and logistic regression (Elasticnet) models, we analyzed metabolite concentration discrepancies in mother–child pairs, with maternal plasma sampled at 24 weeks of gestation and children’s plasma at 6 months. The propensity of vertical transfer was quantified, reflecting the likelihood of accurate mother–child matching. Our findings were substantiated against an external test set and further verified through statistical tests, while the models were explained using permutation importance and SHapley Additive exPlanations (SHAP). The best model was achieved using RF, while xenobiotics were shown to be highly relevant in transfer. The study reaffirms the transmission of certain metabolites, such as perfluorooctanoic acid (PFOA), but also reveals additional insights into the maternal influence on the child’s metabolome. We also discuss the multifaceted nature of vertical transfer. These machine learning-driven insights complement conventional epidemiological findings and offer a novel perspective on using machine learning as a methodology for understanding metabolic interactions.
更多查看译文
关键词
PFOS,PFOA,childhood,pregnancy,propensity
AI 理解论文
溯源树
样例
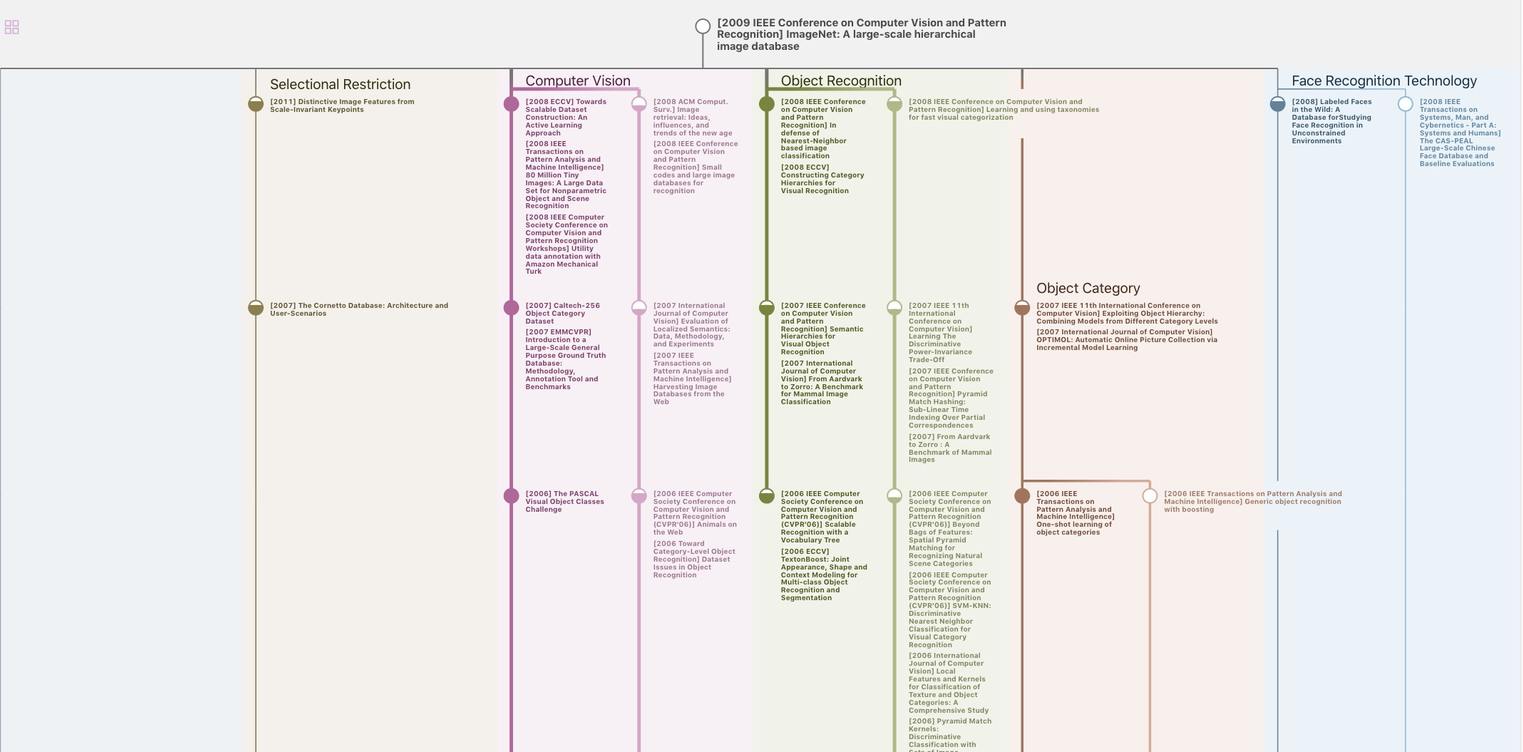
生成溯源树,研究论文发展脉络
Chat Paper
正在生成论文摘要