Keep the Human in the Loop: Arguments for Human Assistance in the Synthesis of Simulation Data for Robot Training
MULTIMODAL TECHNOLOGIES AND INTERACTION(2024)
摘要
Robot training often takes place in simulated environments, particularly with reinforcement learning. Therefore, multiple training environments are generated using domain randomization to ensure transferability to real-world applications and compensate for unknown real-world states. We propose improving domain randomization by involving human application experts in various stages of the training process. Experts can provide valuable judgments on simulation realism, identify missing properties, and verify robot execution. Our human-in-the-loop workflow describes how they can enhance the process in five stages: validating and improving real-world scans, correcting virtual representations, specifying application-specific object properties, verifying and influencing simulation environment generation, and verifying robot training. We outline examples and highlight research opportunities. Furthermore, we present a case study in which we implemented different prototypes, demonstrating the potential of human experts in the given stages. Our early insights indicate that human input can benefit robot training at different stages.
更多查看译文
关键词
robot training,human-in-the-loop,simulation,machine learning
AI 理解论文
溯源树
样例
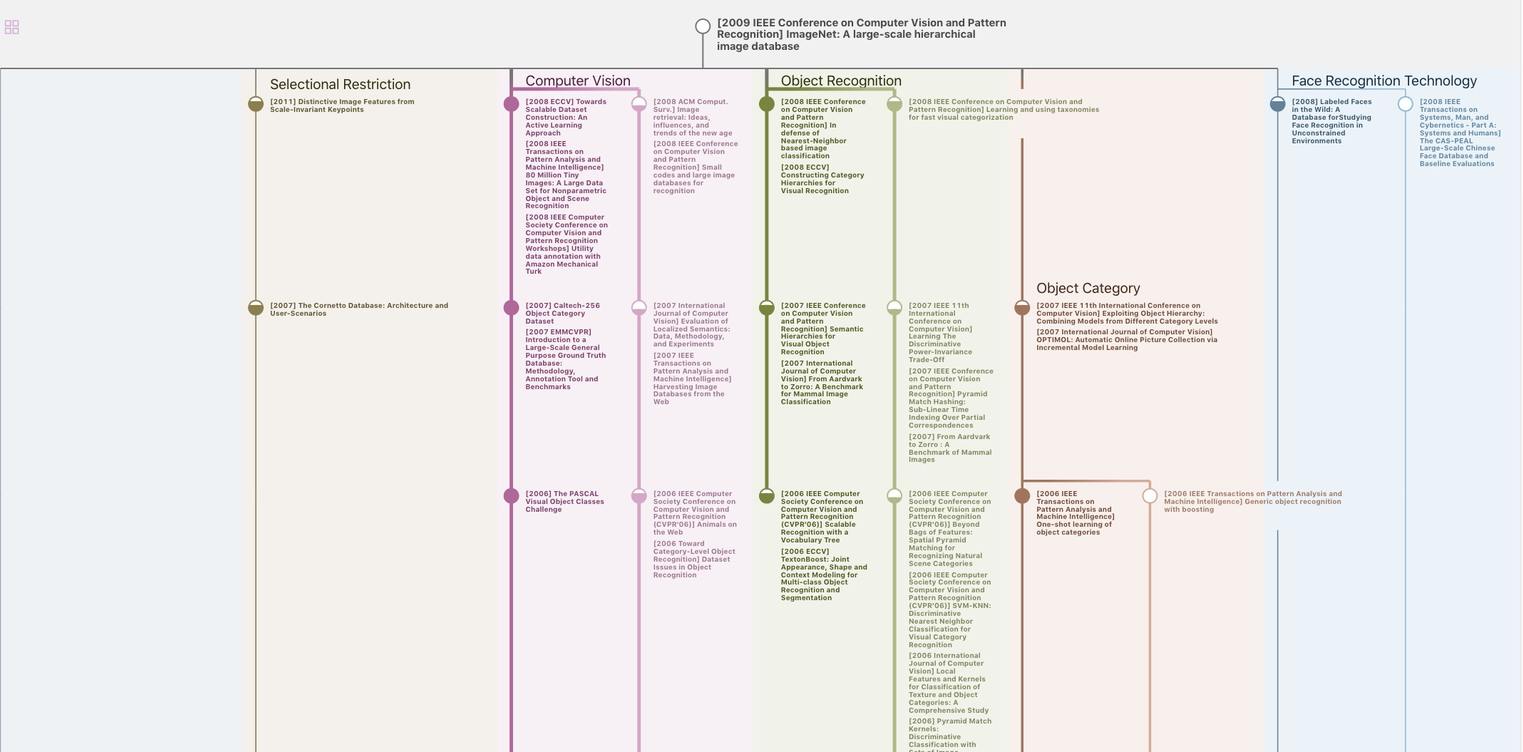
生成溯源树,研究论文发展脉络
Chat Paper
正在生成论文摘要