Knowledge Graph Recommendation based on Structural Semantics and Collaborative Semantics.
International Conference on Parallel and Distributed Systems(2023)
摘要
In recent years, the combination of knowledge graph (KG) and recommendation algorithms has achieved significant success. KG contains rich information to improve users and items representations as side information. Existing KG-aware recommendation methods have shown effectiveness in some cases. However, we contend these methods mainly focus on extracting structural semantic information from KG, while not fully exploring the collaborative semantic information (semantically similar information that may not be directly reflected in the graph structure). Considering the heterogeneity of KG, we design a novel method under the contrastive learning paradigm, named Knowledge Graph Recommendation based on Structural Semantics and Collaborative Semantics (KGSC). In this paper, we focus on exploring the integration of collaborative semantic information and studying the complementary relationship between structural semantics and collaborative semantics. Specifically, we assume that users (items) with similar representations exist in the same latent space. By mapping all entities representations into a collaborative semantic space, we are able to capture the underlying "commonality" features among entities. Then, we include them into contrastive pairs, to better mine users (items) features and relationships. Extensive experiments on three real-world public datasets show that our KGSC consistently outperforms state-of-the-art techniques.
更多查看译文
AI 理解论文
溯源树
样例
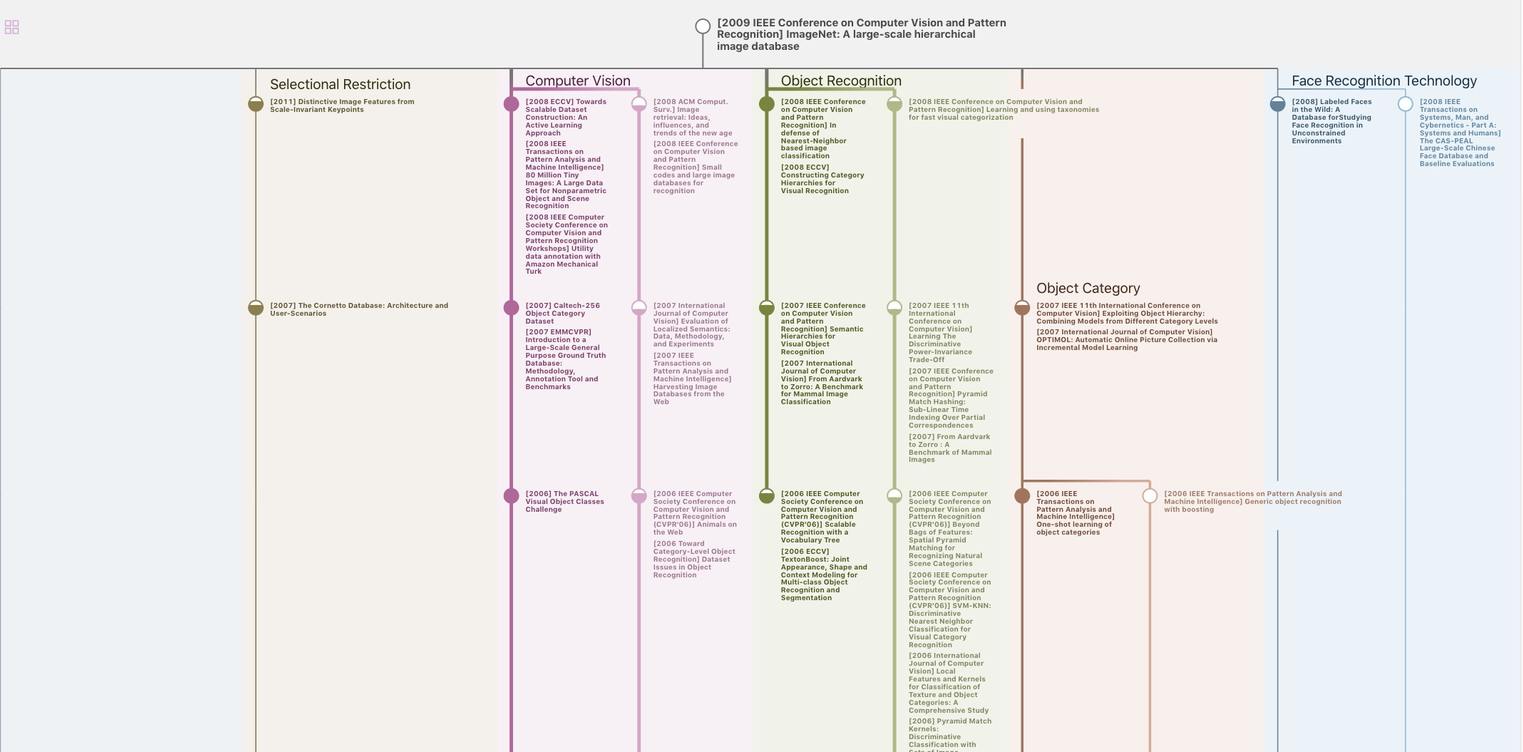
生成溯源树,研究论文发展脉络
Chat Paper
正在生成论文摘要