A two-stage framework for pixel-level pavement surface crack detection
Engineering Applications of Artificial Intelligence(2024)
摘要
Surface crack is one of the most common distresses of pavement structure, impacting its serviceability and sustainability. Over the past decade, many efforts have been devoted to developing computer vision-based models (e.g., image processing- or deep learning-based) for the automatic detection of pavement surface crack. However, there is a great gap between the public image data taken by the phone or other portable devices and the real-world image data acquired by the linear array charge-coupled device (CCD) camera, which usually is high resolution and contains limited crack pixels per image. To improve the pavement surface crack detection efficiency and accuracy in engineering practice, we propose a novel two-stage framework for automatic pavement surface detection at the pixel level. In stage I, the images concluding pixel cracks are selected using a convolutional neural network (CNN)-based classification network. In stage II, the selected images are processed with our proposed separation-combination strategy and the second version of crack transformer (CTv2) for pavement surface crack detection at the pixel level. Comprehensive experimental investigation and comparison have been conducted on training performance and visualization results, validating the superiority of the developed framework. It paves the way for the large-scale application of automatic pavement crack detection in an efficient manner.
更多查看译文
关键词
Crack detection,Transformer,Pixel-level,Semantic segmentation,Encoder-decoder
AI 理解论文
溯源树
样例
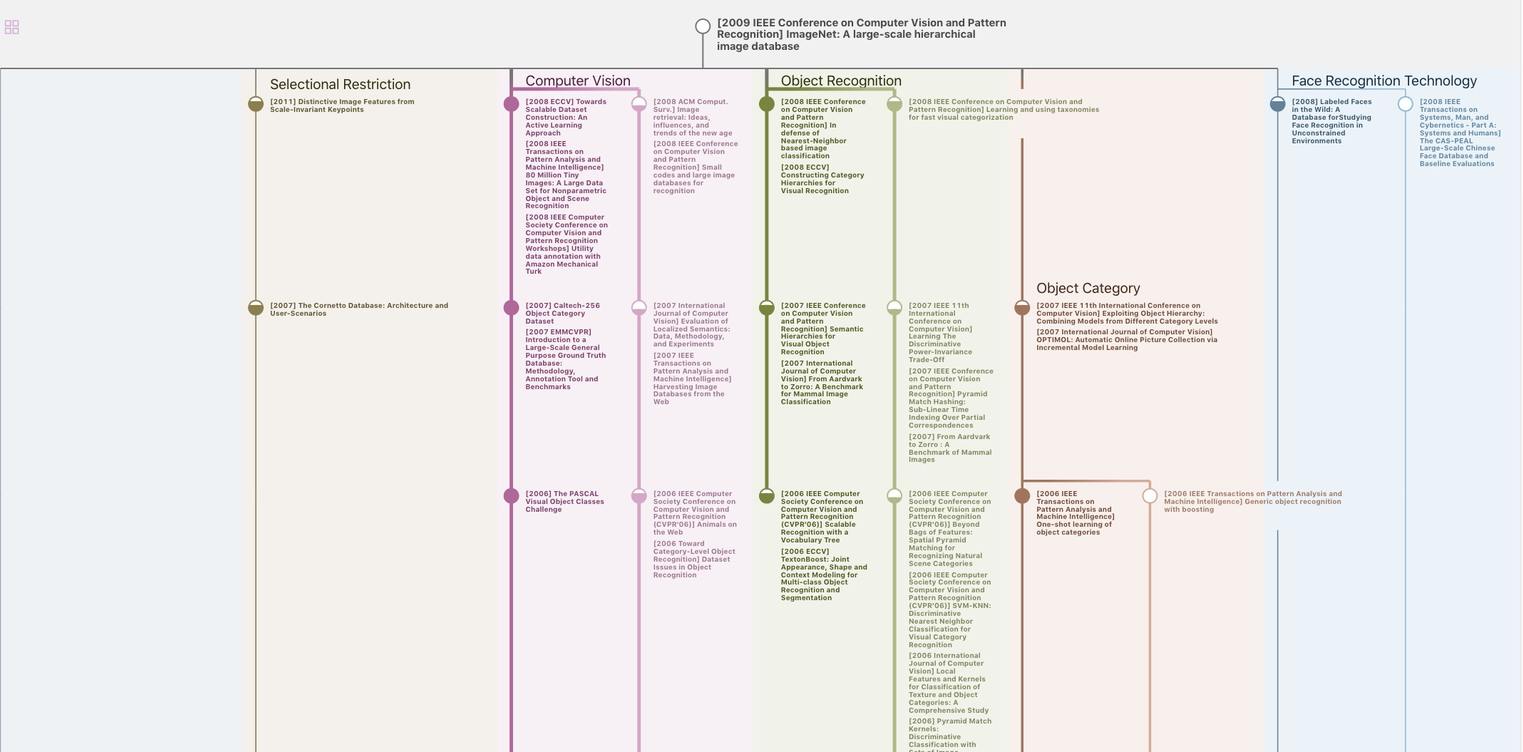
生成溯源树,研究论文发展脉络
Chat Paper
正在生成论文摘要