Optimized deep learning models for effluent prediction in wastewater treatment processes
ENVIRONMENTAL SCIENCE-WATER RESEARCH & TECHNOLOGY(2024)
摘要
Monitoring wastewater holds significant importance in minimizing pollution discharge and enhancing resource utilization. This study aims to develop a soft sensor for the prediction and optimization of critical wastewater treatment indicators. To address the nonlinear characteristics of the wastewater process, this work introduces a novel model incorporating the convolutional neural network (CNN) and gated recursive unit (GRU). Considering the high-dimensional data in practical production processes, feature selection based on Lasso regression is employed to identify key features for the training model. In addition, the proposed CNN-GRU model is enhanced using parameters determined by the Bayesian optimization algorithm in this work. The model is trained using urban wastewater data to predict effluent chemical oxygen demand (COD) and suspended solids (SS). For COD prediction, the test set achieves a coefficient of determination (R2) of 0.97, and it attains an R2 of 0.90 for SS prediction, demonstrating superior generalization ability. Furthermore, comparative analysis with random forest, long short-term memory, CNN, and GRU models reveals the improved prediction performance of the CNN-GRU model after Bayesian optimization. Aiming at prediction of water quality in wastewater treatment systems, an optimized deep learning model with high prediction performance is proposed.
更多查看译文
AI 理解论文
溯源树
样例
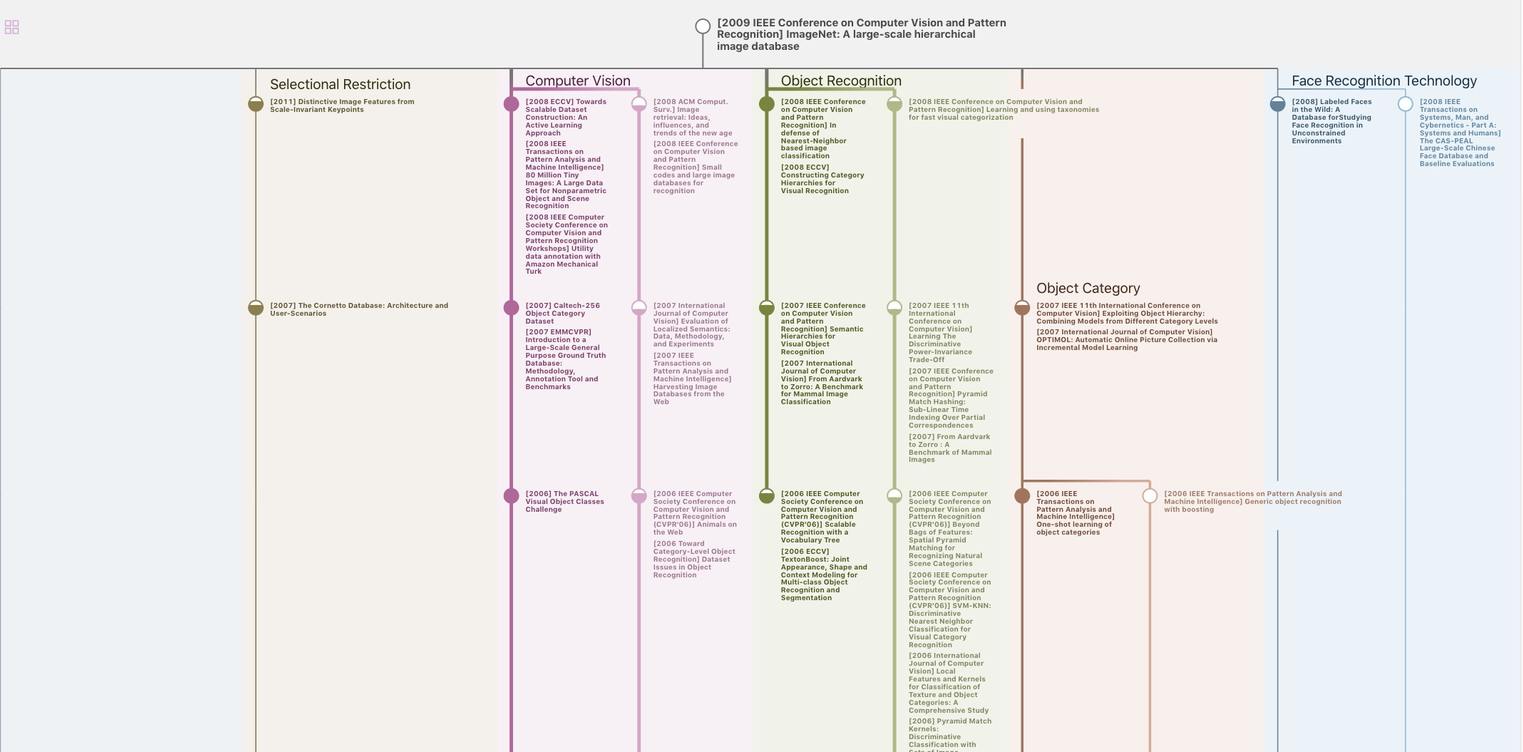
生成溯源树,研究论文发展脉络
Chat Paper
正在生成论文摘要