An Analysis of Federated Learning Performance in Mobile Networks
2023 IEEE Virtual Conference on Communications (VCC)(2023)
摘要
The increase in personal data collection for service customization threatens users' privacy. In federated learning, privacy can be preserved by distributing the learning process, where only neural networks' weights are shared between clients and servers. This work evaluates the impact of different networks parameters on the performance of federated learning models in a mobile-oriented scenario. Particularly, we consider the performance of convolutional neural networks for image classification. The experiments use the Flower framework and the CIFAR-10 dataset for image classification. Typical parameters in mobile networks, such as latency, connectivity, data volume, and client availability, are evaluated. Our results show that, in addition to the increase in total training time, a higher number of disconnected users in a training round can negatively impact the model performance. These results indicate the need for client-server orchestration to perform dynamic adaptation of network conditions.
更多查看译文
关键词
Federated learning,wireless mobile networks
AI 理解论文
溯源树
样例
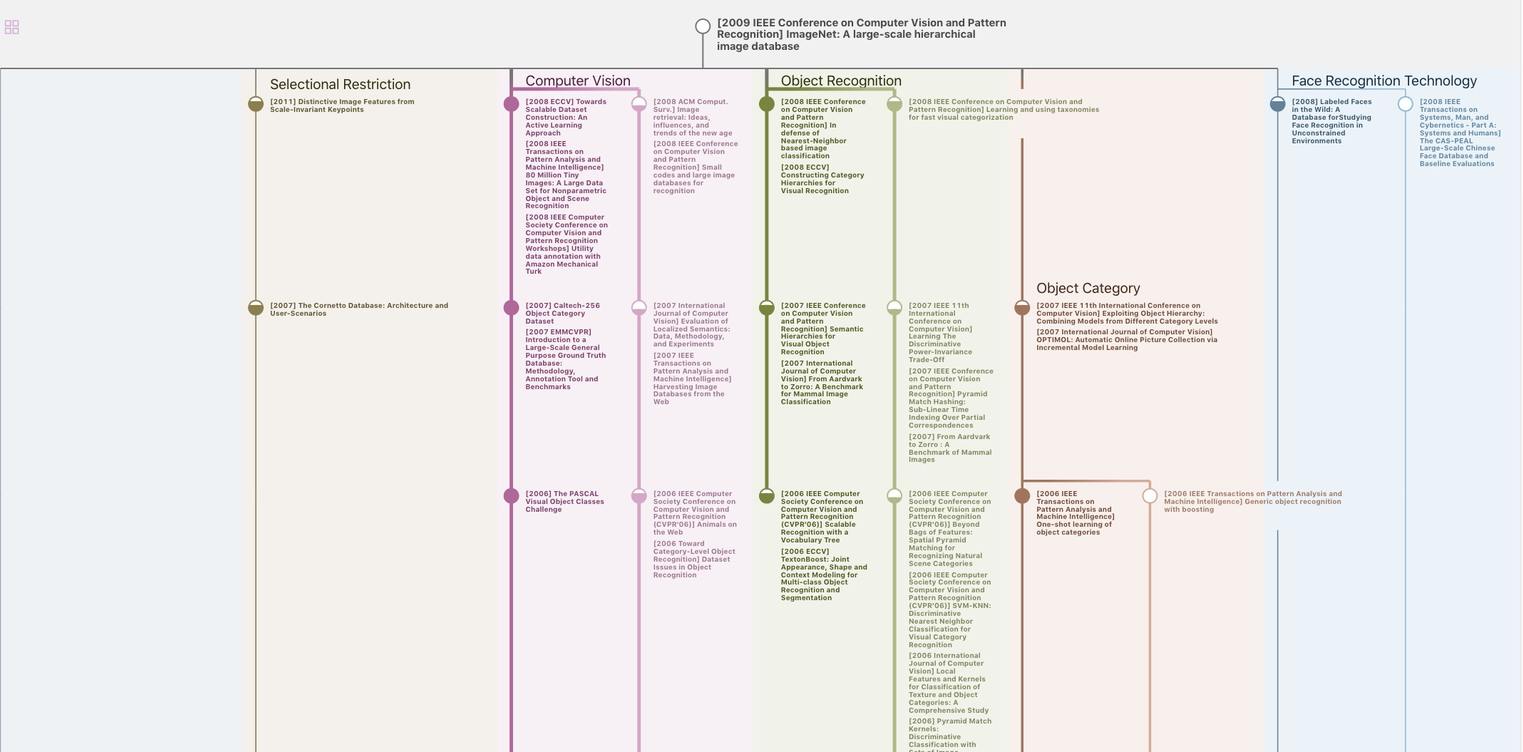
生成溯源树,研究论文发展脉络
Chat Paper
正在生成论文摘要