Path Loss Prediction for Vehicular-to-Infrastructure Communication Using Machine Learning Techniques.
VCC(2023)
摘要
Vehicular communications are becoming increasingly important due to the need for safer, more efficient, and sustainable transportation. They require the development of accurate radio channel models for vehicular environments. This study compares vehicle-to-infrastructure path loss predictions obtained using four machine learning models: artificial neural network, support vector regression, random forest, and gradient tree boosting. The model design employs predictors from the profile environment between the transmitter and receiver positions. A methodology to select the best predictor subset is applied by examining their contribution to performance and interpretability. We propose a generalization test considering unknown streets (scenarios), and the results demonstrate that the gradient tree boosting model significantly improves the path loss prediction compared to the log-distance path loss model.
更多查看译文
关键词
Path loss,Vehicular-to-infrastructure,Machine learning,Predictor selection technique
AI 理解论文
溯源树
样例
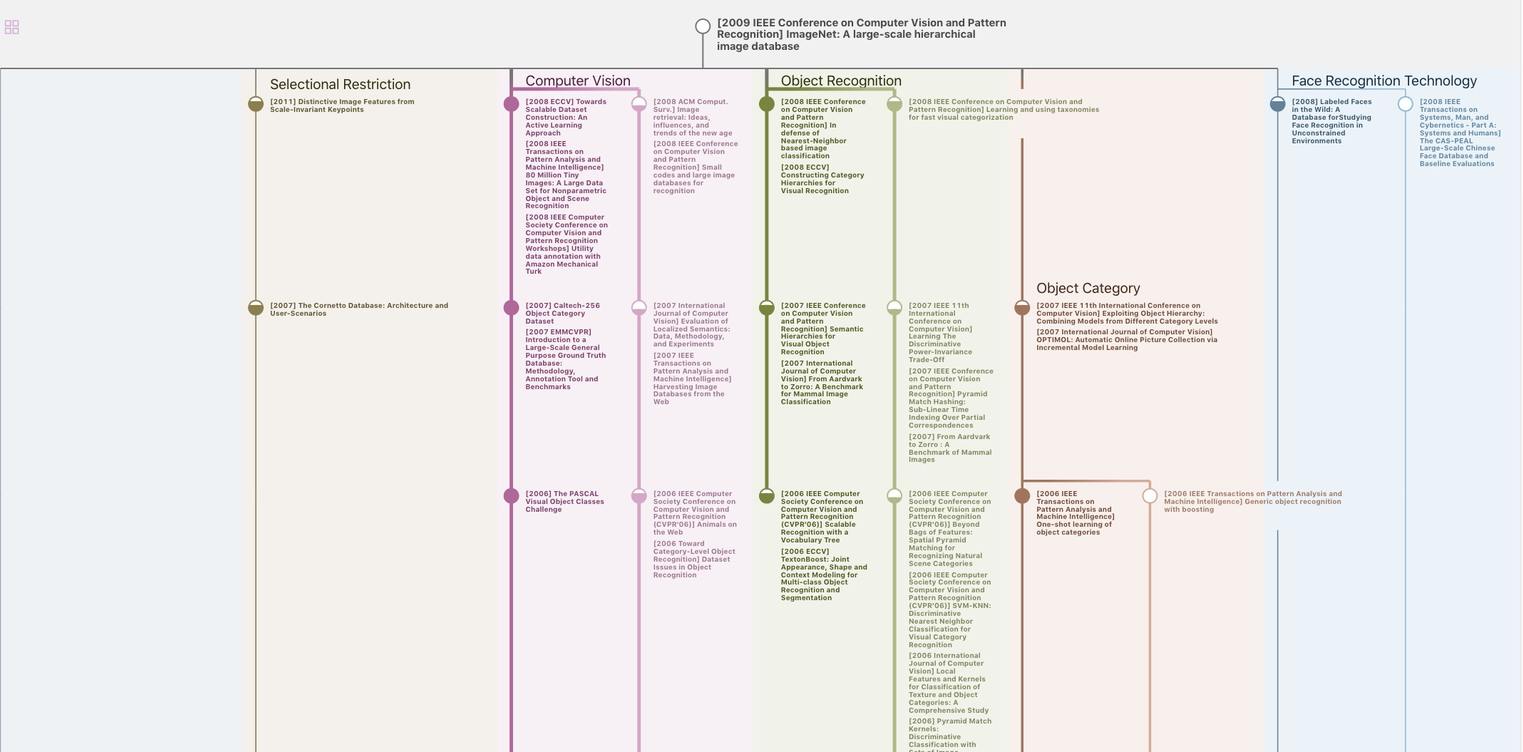
生成溯源树,研究论文发展脉络
Chat Paper
正在生成论文摘要