An Adaptive Teacher-Student Framework for Real-time Video Inference in Multi-User Heterogeneous MEC Networks.
International Conference on Parallel and Distributed Systems(2023)
摘要
Teacher-student learning has emerged as a promising framework for real-time video inference on mobile devices in multi-access edge computing (MEC) networks, where heavyweight teacher models are deployed on edge servers, and lightweight student models distilled from teacher models are deployed on mobile devices. To deal with data drift and maintain the inference accuracy, the student model has to be updated periodically with the help of the teacher model through a training process. Different training configurations, such as training epochs and frozen layers, lead to different accuracy improvements with different resource requirements. However, in multi-user heterogeneous MEC networks, due to resource heterogeneity and limited computing resources of edge servers, it is quite challenging to update all the student models simultaneously to achieve high inference accuracy. To address this problem, in this paper, we propose an adaptive teacher-student framework in multi-user heterogeneous MEC networks. The key idea is to adaptively make optimal updating decisions (i.e., offloading decision and configuration selection decision) for each user, where the available resources of edge servers and network conditions are taken into account. First, we model the teacher-student collaborative video inference problem as an optimization problem with the aim of maximizing the average inference accuracy. Then, we propose an evolutionary deep reinforcement learning algorithm, CEM-MASAC, to solve this problem. Finally, trace-driven simulations employing real-world bandwidth traces demonstrate the superiority of our algorithm compared to the baseline methods.
更多查看译文
关键词
video inference,multi-access edge computing,deep reinforcement learning,teacher-student learning,model updating
AI 理解论文
溯源树
样例
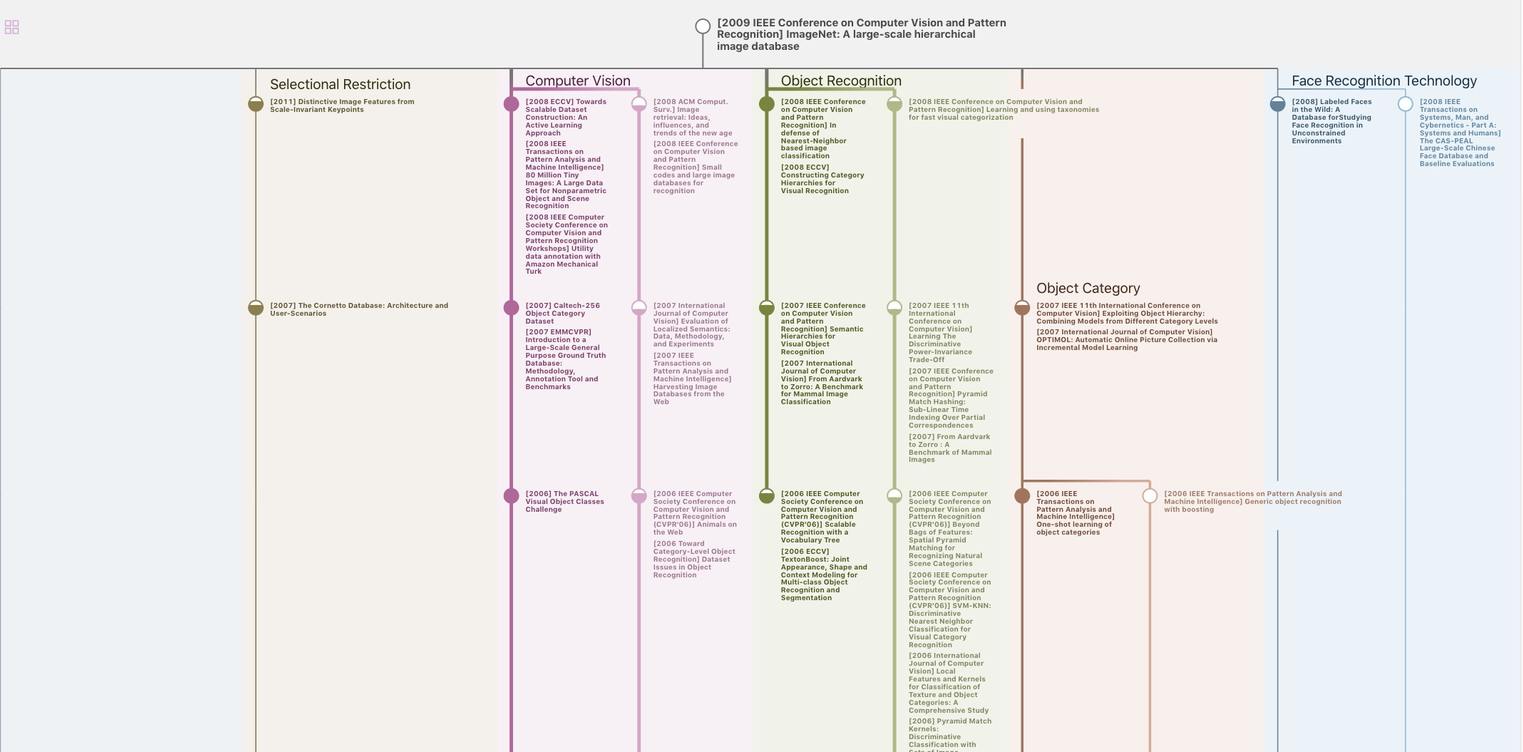
生成溯源树,研究论文发展脉络
Chat Paper
正在生成论文摘要