Self-supervised Multi-task Distillation for Few-shot Classification.
International Conference on Parallel and Distributed Systems(2023)
摘要
Few-shot classification has gained significant attention owing to the effectiveness in classifying unseen classes with a few annotated images. Although previous works achieve encouraging classification performance, they heavily rely on one-hot labels during the meta-learning process, which may result in the supervision collapse and limited generalization. To address these challenges, the few-shot classification based on a self-supervised multi-task distillation (SMD) is proposed for mitigating the nuisance arising from one-hot labels. Specifically, SMD formulates multiple auxiliary tasks to enhance the cross entropy classification in a multi-task learning manner, including the self-supervised classification task and the self-distilled classification task. These auxiliary tasks do not rely on one-hot labels in meta-learning, which can effectively enhance generalization performance of the model. Finally, extensive experiment results on two benchmark datasets, i.e., CIFAR-FS and FC-100, demonstrate the superiority and effectiveness of SMD.
更多查看译文
关键词
few-shot classification,self distillation,self supervision,multi-task learning
AI 理解论文
溯源树
样例
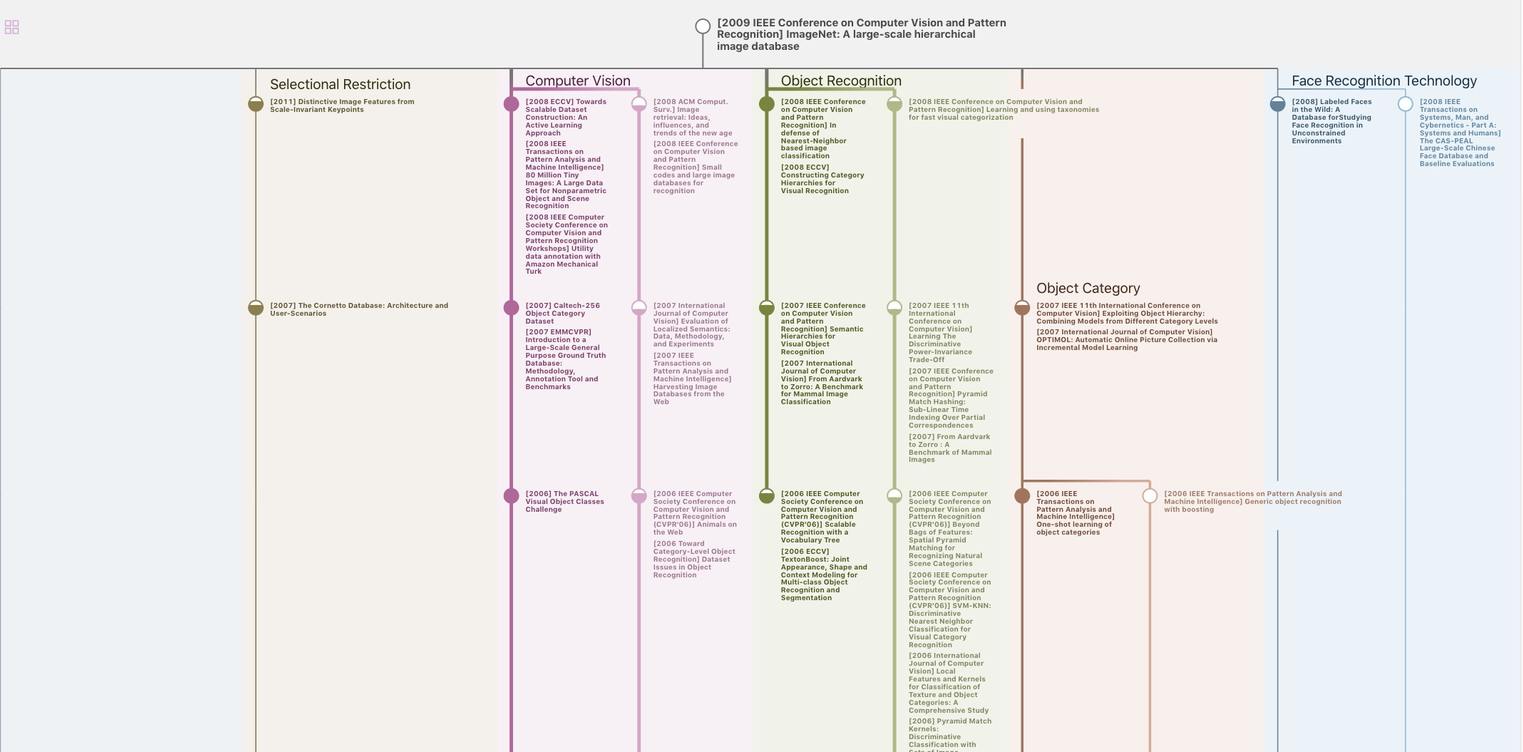
生成溯源树,研究论文发展脉络
Chat Paper
正在生成论文摘要