#Graph embedding orthogonal decomposition: a synchronous feature selection technique based on collaborative particle swarm optimization
Pattern Recognition(2024)
摘要
In unsupervised feature selection, the clustering label matrix has the ability to distinguish between projection clusters. However, the latent geometric structure of the clustering labels is often ignored. In addition, the optimal sub-feature selection performance of feature selection techniques relies greatly on the choice of balanced parameters, and the selection range of most technical parameters is limited and fixed. To solve the above-mentioned problems, this paper proposes a synchronous feature selection technique based on graph-embedded cluster label orthogonal decomposition and collaborative particle swarm optimization (GOD-cPSO). First, GOD-cPSO extends the feature selection framework of clustering label orthogonal decomposition by graph embedding to retain the latent geometric structure of clustering labels, thus maintaining the correlation between clustered sample labels. Then, the l2,1-2-norm with strong global convergence is extended to the graph embedding clustering label orthogonal decomposition framework. By imposing this non-convex constraint, GOD-cPSO can achieve low-dimensional sparse and low-redundant sub-features. In addition, the local structure preserving of low-dimensional manifolds is integrated into the graph-embedded clustering label orthogonal decomposition framework to obtain good cluster separation and effectively maintain the latent local structure of the data. Finally, to ensure the adaptive parameter selection over a large range, GOD-cPSO synchronously guides the graph-embedding clustering labeling orthogonal decomposition framework for feature selection through collaborative particle swarm optimization. GOD-cPSO has synchronous parameter optimization and feature selection and picks parameters in a larger range. Comprehensive numerical experiments are performed on nine datasets to test the validity of the GOD-cPSO. The experimental results demonstrate that the sub-features selected by the GOD-cPSO have stronger discriminative power and are superior to other techniques in the clustering assignments.
更多查看译文
关键词
Clustering label orthogonal decomposition,Graph-embedded,Local structure preserving,Particle swarm optimization
AI 理解论文
溯源树
样例
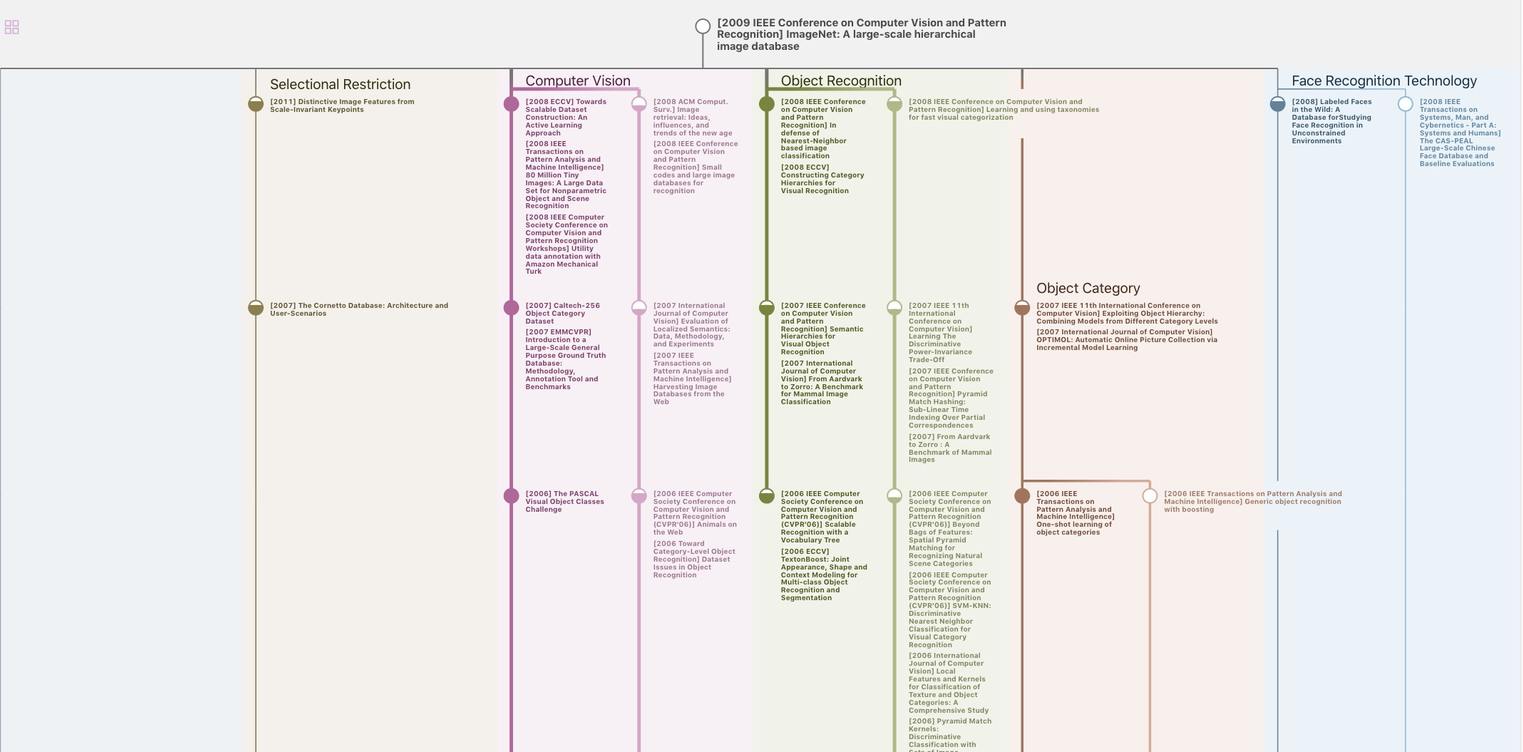
生成溯源树,研究论文发展脉络
Chat Paper
正在生成论文摘要