Digital twin enhanced quality prediction method of powder compaction process
Robotics and Computer-Integrated Manufacturing(2024)
摘要
During the powder compaction process, process parameters are required for product quality prediction. However, the inadequacy of compaction data leads to difficulties in constructing models for quality prediction. Meanwhile, the existing data generation methods can only generate required data partially, and fail to generate data for extreme operating conditions and difficult-to-measure quality parameters. To address this issue, a digital twin (DT) enhanced quality prediction method for powder compaction process is presented in this paper. First, a DT model of the powder compaction process with multiple dimensions is constructed and validated. Then, to solve the data inadequacy problem, data of process parameters are generated through an orthogonal experimental design, and are imported into the DT model to generate quality parameters, so as to obtain the virtual data. Finally, the quality prediction for the powder compaction process is achieved by the generative adversarial network-deep neural network (GAN-DNN) method. The effectiveness of the generated virtual data and the GAN-DNN method is verified through experimental comparison. On top of point-to-point validation, a quality prediction system applied in a powder compaction line is developed and implemented to demonstrate the end-to-end practicability of the proposed method.
更多查看译文
关键词
Digital twin,Data generation,Quality prediction,Powder compaction process
AI 理解论文
溯源树
样例
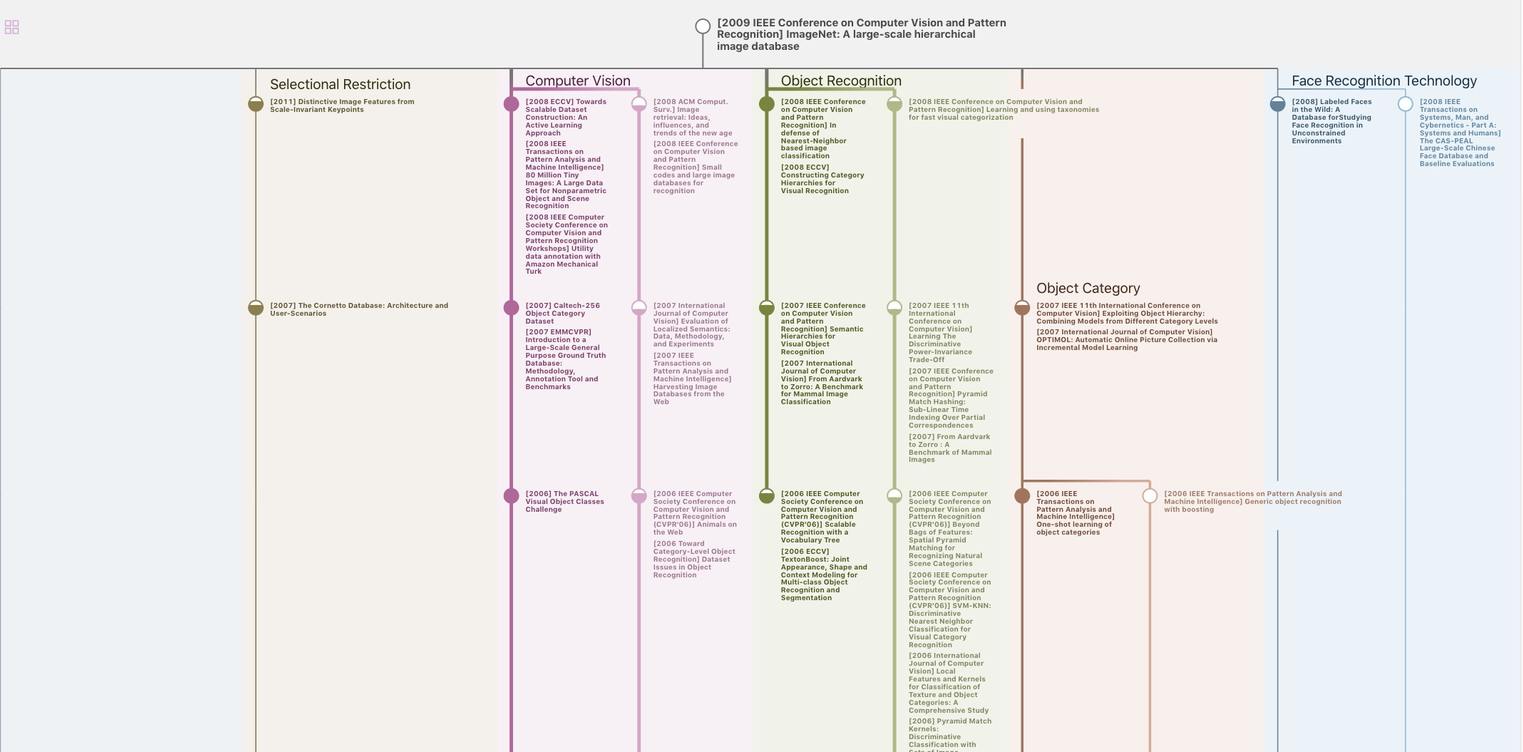
生成溯源树,研究论文发展脉络
Chat Paper
正在生成论文摘要