Model-agnostic explanations for survival prediction models.
Statistics in medicine(2024)
摘要
Advanced machine learning methods capable of capturing complex and nonlinear relationships can be used in biomedical research to accurately predict time-to-event outcomes. However, these methods have been criticized as "black boxes" that are not interpretable and thus are difficult to trust in making important clinical decisions. Explainable machine learning proposes the use of model-agnostic explainers that can be applied to predictions from any complex model. These explainers describe how a patient's characteristics are contributing to their prediction, and thus provide insight into how the model is arriving at that prediction. The specific application of these explainers to survival prediction models can be used to obtain explanations for (i) survival predictions at particular follow-up times, and (ii) a patient's overall predicted survival curve. Here, we present a model-agnostic approach for obtaining these explanations from any survival prediction model. We extend the local interpretable model-agnostic explainer framework for classification outcomes to survival prediction models. Using simulated data, we assess the performance of the proposed approaches under various settings. We illustrate application of the new methodology using prostate cancer data.
更多查看译文
关键词
explainer,LIME,predictive modeling,random survival forest
AI 理解论文
溯源树
样例
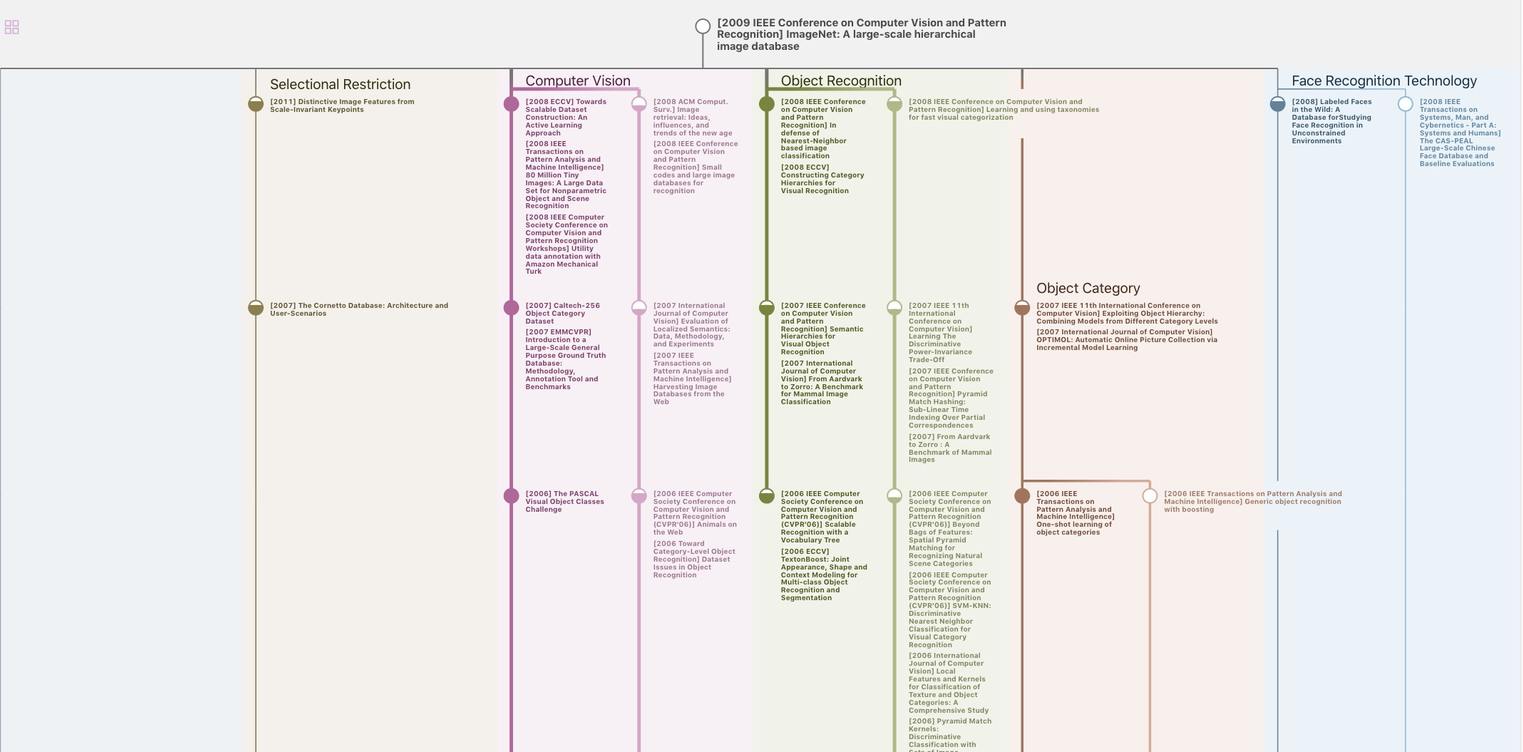
生成溯源树,研究论文发展脉络
Chat Paper
正在生成论文摘要