Model-free reinforcement learning for motion planning of autonomous agents with complex tasks in partially observable environments
Autonomous Agents and Multi-Agent Systems(2024)
摘要
Motion planning of autonomous agents in partially known environments with incomplete information is a challenging problem, particularly for complex tasks. This paper proposes a model-free reinforcement learning approach to address this problem. We formulate motion planning as a probabilistic-labeled partially observable Markov decision process (PL-POMDP) problem and use linear temporal logic (LTL) to express the complex task. The LTL formula is then converted to a limit-deterministic generalized Büchi automaton (LDGBA). The problem is redefined as finding an optimal policy on the product of PL-POMDP with LDGBA based on model-checking techniques to satisfy the complex task. We implement deep Q learning with long short-term memory (LSTM) to process the observation history and task recognition. Our contributions include the proposed method, the utilization of LTL and LDGBA, and the LSTM-enhanced deep Q learning. We demonstrate the applicability of the proposed method by conducting simulations in various environments, including grid worlds, a virtual office, and a multi-agent warehouse. The simulation results demonstrate that our proposed method effectively addresses environment, action, and observation uncertainties. This indicates its potential for real-world applications, including the control of unmanned aerial vehicles.
更多查看译文
关键词
Motion planning,Partially observable environments,Complex tasks,Linear temporal logic,Reinforcement learning,Recurrent neural networks
AI 理解论文
溯源树
样例
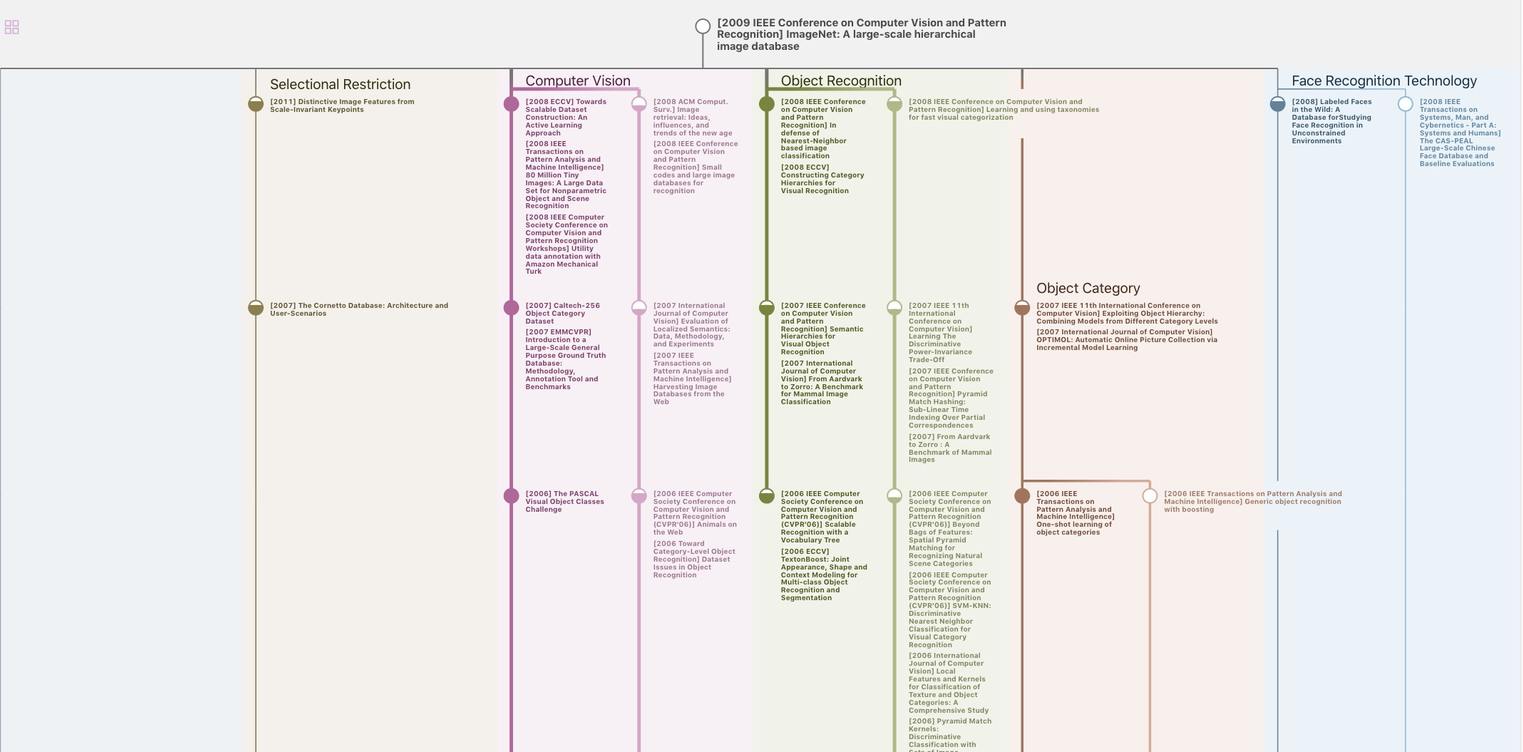
生成溯源树,研究论文发展脉络
Chat Paper
正在生成论文摘要