Impulse Noise Suppression by Deep Learning-Based Receivers in OFDM Systems
Wireless Personal Communications(2024)
摘要
Orthogonal Frequency Division Multiplexing (OFDM) systems are prone to signal corruption caused by strong and frequent impulses, which can be further exacerbated by multipath fading. Recent evolutions highlight the efficacy of a deep neural network (DNN) receiver in intrinsically estimating channel state information and recovering data explicitly, even without presuming the signal-to-noise ratio (SNR) level. However, the conventional DNN-based receiver, trained on data generated from computer simulations with WINNER II channel model and additive white Gaussian noise (AWGN), is susceptible to substantial performance degradation when subject to impulse noise. To address this challenge, this paper proposes fine-tuning the DNN model using impulse noise-laced data samples during subsequent training. The proposed method aims to enhance representation learning and improve the robustness of the receiver against impulse noise. The efficacy of the DNN-based receiver is assessed by comparing its bit error rate (BER) performance to that of a compressive sensing-based receiver, enabled by the consensus alternating direction method of multipliers (ADMM). Remarkably, the proposed DNN-based receiver achieves BER performance comparable to the clipping-featured receiver, which requires knowledge of the SNR value, an assumption relaxed by our enhanced DNN approach. Furthermore, extensive simulations demonstrate the promising robustness of the deep learning-based approach against impulse noise model mismatches between training and testing scenarios.
更多查看译文
关键词
Neural network,ADMM,Impulse noise,OFDM,Compressive sensing
AI 理解论文
溯源树
样例
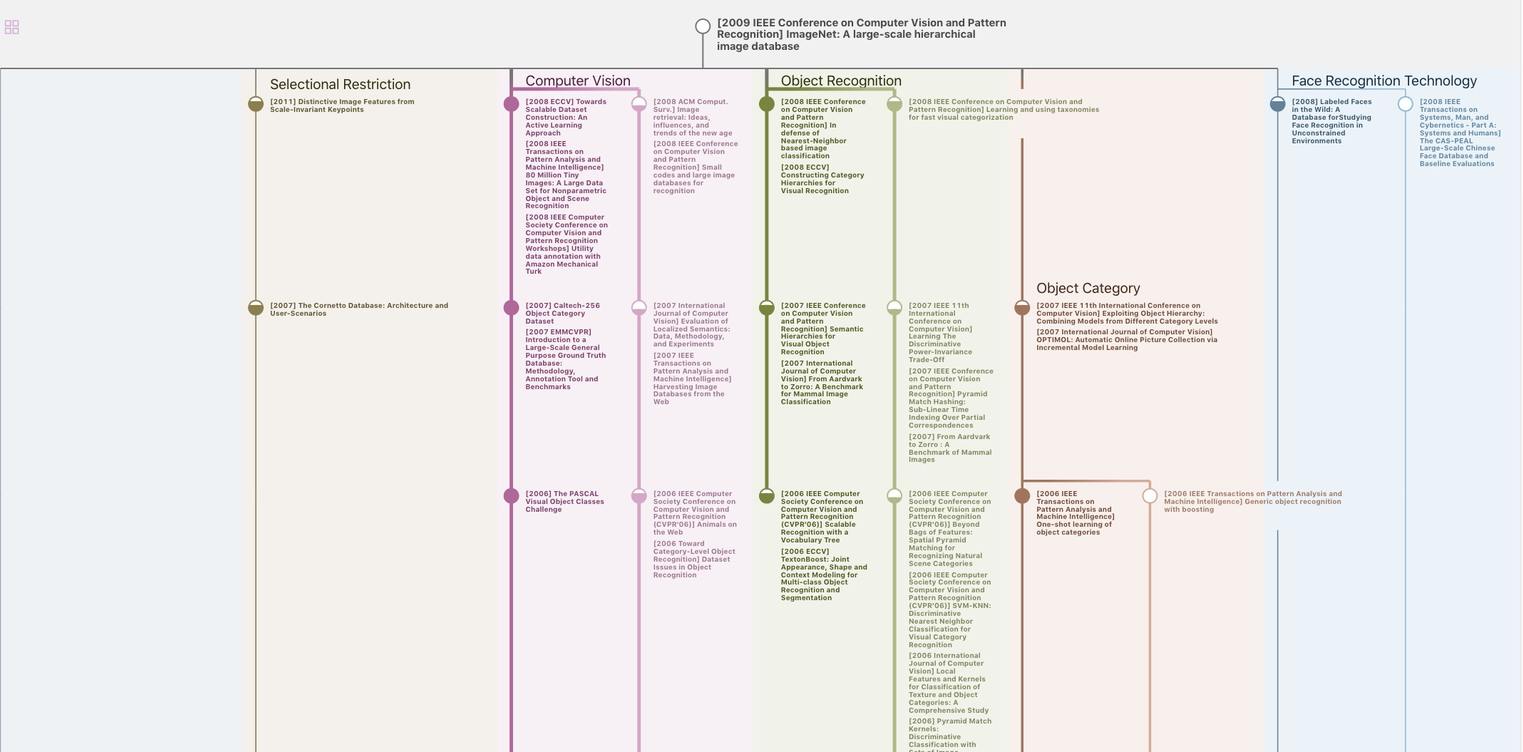
生成溯源树,研究论文发展脉络
Chat Paper
正在生成论文摘要