When Sparse Graph Representation Learning Falls into Domain Shift: Data Augmentation for Cross-Domain Graph Meta-Learning (Student Abstract)
AAAI 2024(2024)
摘要
Cross-domain Graph Meta-learning (CGML) has shown its promise, where meta-knowledge is extracted from few-shot graph data in multiple relevant but distinct domains. However, several recent efforts assume target data available, which commonly does not established in practice. In this paper, we devise a novel Cross-domain Data Augmentation for Graph Meta-Learning (CDA-GML), which incorporates the superiorities of CGML and Data Augmentation, has addressed intractable shortcomings of label sparsity, domain shift, and the absence of target data simultaneously. Specifically, our method simulates instance-level and task-level domain shift to alleviate the cross-domain generalization issue in conventional graph meta-learning. Experiments show that our method outperforms the existing state-of-the-art methods.
更多查看译文
关键词
Graph Neural Networks,Few-shot Learning,Domain Generalization
AI 理解论文
溯源树
样例
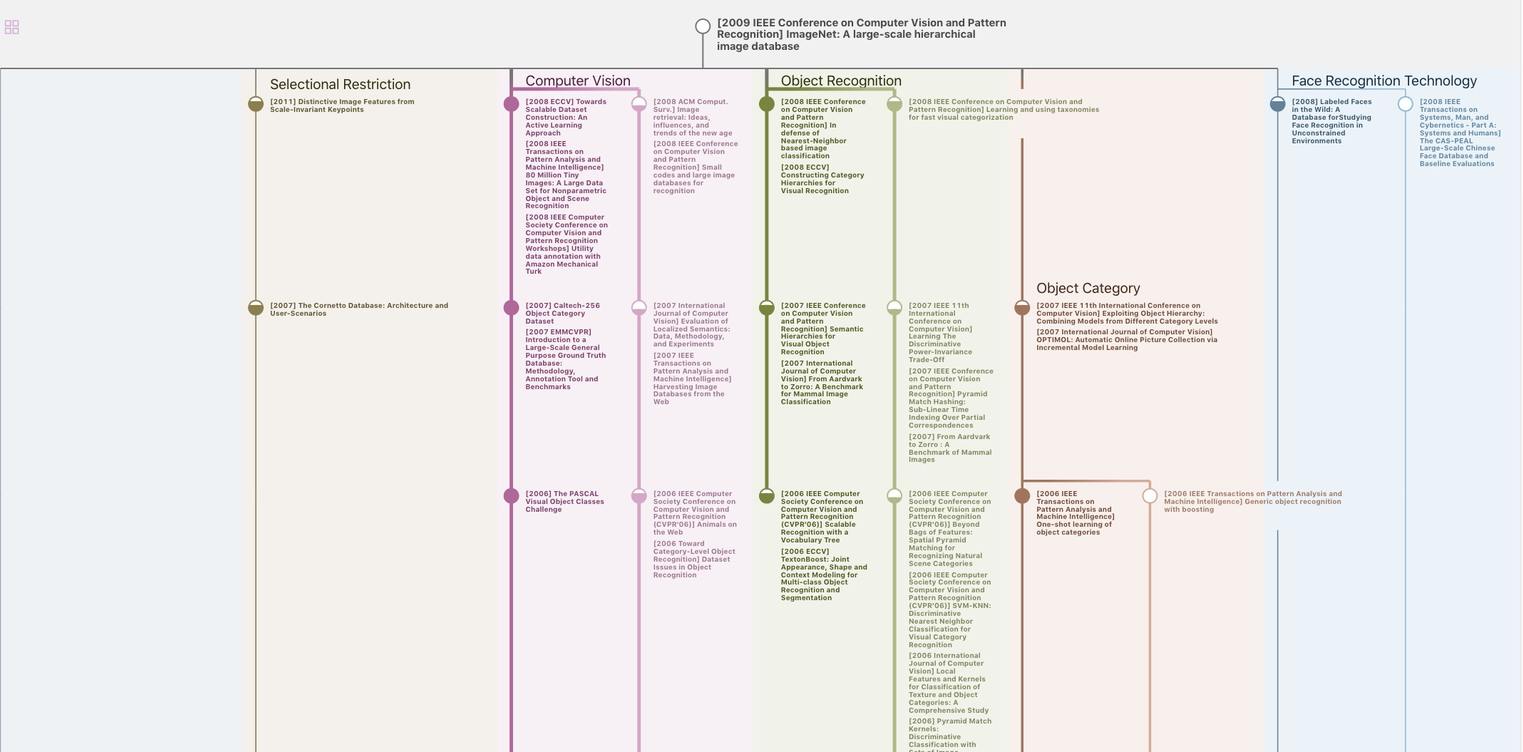
生成溯源树,研究论文发展脉络
Chat Paper
正在生成论文摘要