Efficient low-overlapping point cloud registration based on two-stage network learning
REMOTE SENSING LETTERS(2024)
摘要
This letter introduces an efficient registration method for large-scale point clouds with low overlap, which adopts a two-stage transformation learning process. In the first stage, we integrate a point-voxel encoding structure with an attention mechanism to predict rotation and point-wise importance weight. In the second stage, we employ a PointNet-based structure to estimate translation using optimized data. By adopting this two-stage procedure, our model can effectively learn rotation and translation separately, thereby increasing flexibility. We conducted extensive experiments on two large-scale indoor datasets (3Dmatch and S3DIS) and an outdoor dataset (KITTI odometry). The results demonstrate that our method outperforms traditional and deep learning-based approaches, achieving state-of-the-art performance in registration. Furthermore, our method exhibits superior generalization capabilities, highlighting its effectiveness in various registration scenarios.
更多查看译文
关键词
Fast 3D registration,low overlapping scenario,large scale point clouds
AI 理解论文
溯源树
样例
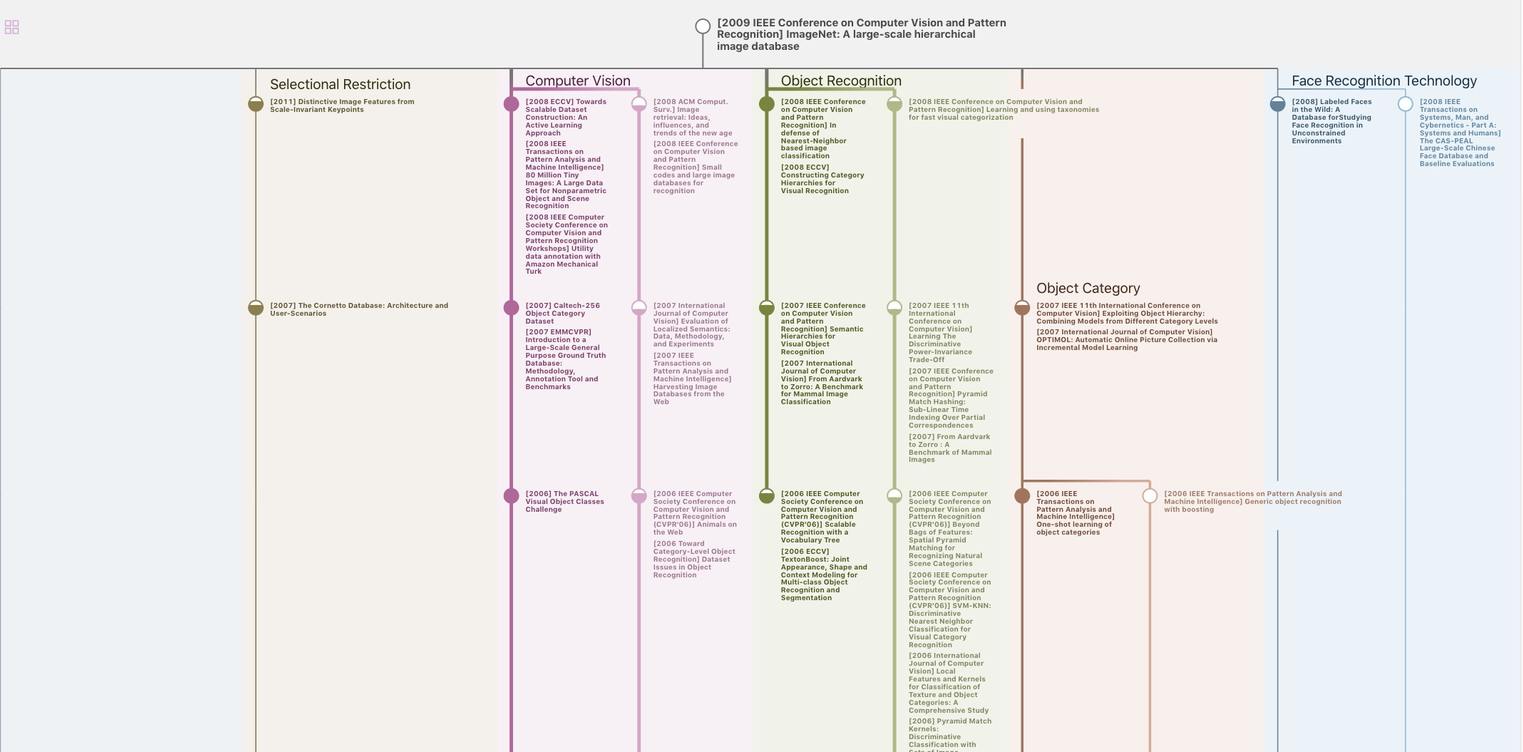
生成溯源树,研究论文发展脉络
Chat Paper
正在生成论文摘要