Towards Evidential and Class Separable Open Set Object Detection
AAAI 2024(2024)
摘要
Detecting in open-world scenarios poses a formidable challenge for models intended for real-world deployment. The advanced closed set object detectors achieve impressive performance under the closed set setting, but often produce overconfident misprediction on unknown objects due to the lack of supervision. In this paper, we propose a novel Evidential Object Detector (EOD) to formulate the Open Set Object Detection (OSOD) problem from the perspective of Evidential Deep Learning (EDL) theory, which quantifies classification uncertainty by placing the Dirichlet Prior over the categorical distribution parameters. The task-specific customized evidential framework, equipped with meticulously designed model architecture and loss function, effectively bridges the gap between EDL theory and detection tasks. Moreover, we utilize contrastive learning as an implicit means of evidential regularization and to encourage the class separation in the latent space. Alongside, we innovatively model the background uncertainty to further improve the unknown discovery ability. Extensive experiments on benchmark datasets demonstrate the outperformance of the proposed method over existing ones.
更多查看译文
关键词
CV: Object Detection & Categorization,CV: Adversarial Attacks & Robustness
AI 理解论文
溯源树
样例
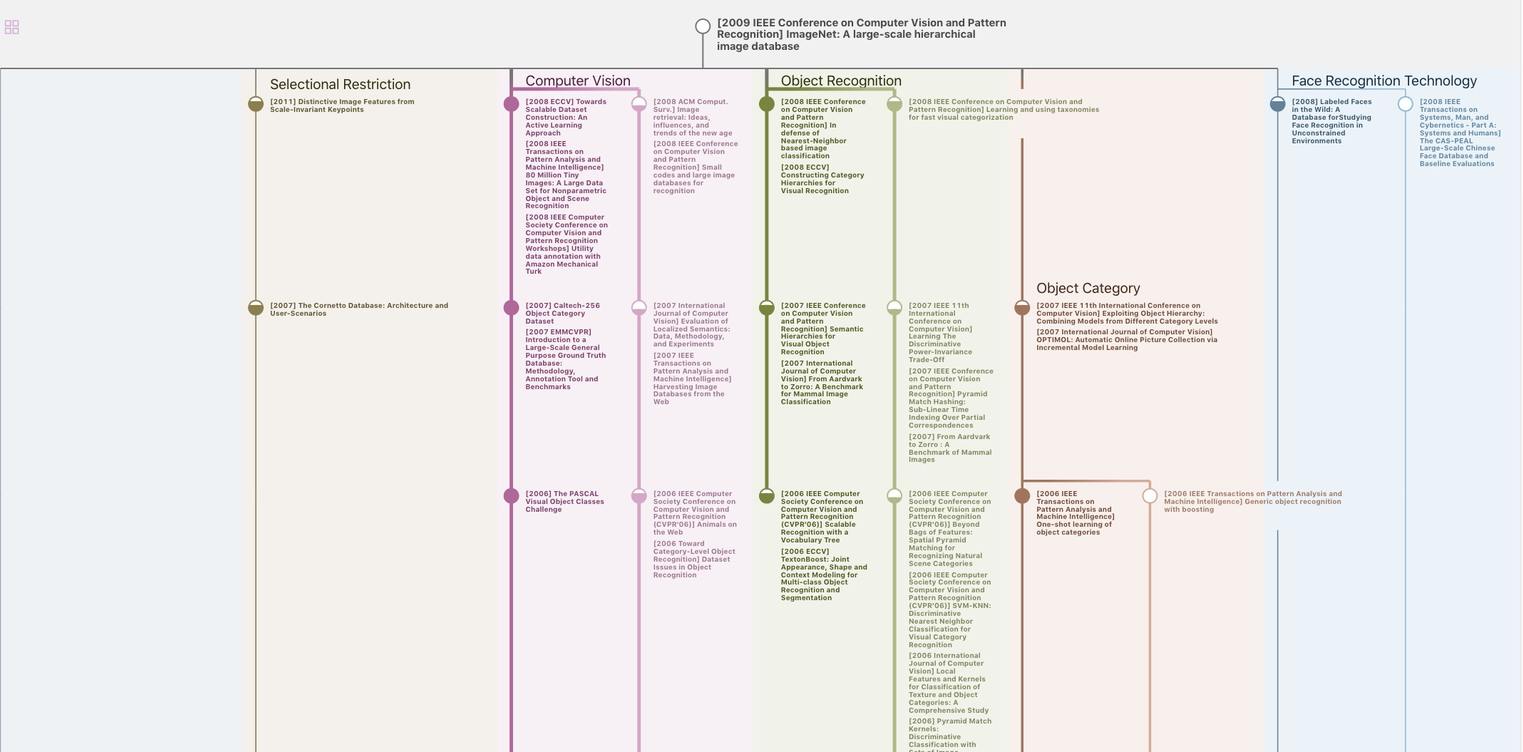
生成溯源树,研究论文发展脉络
Chat Paper
正在生成论文摘要