LAFA: Multimodal Knowledge Graph Completion with Link Aware Fusion and Aggregation
AAAI 2024(2024)
摘要
Recently, an enormous amount of research has emerged on multimodal knowledge graph completion (MKGC), which seeks to extract knowledge from multimodal data and predict the most plausible missing facts to complete a given multimodal knowledge graph (MKG). However, existing MKGC approaches largely ignore that visual information may introduce noise and lead to uncertainty when adding them to the traditional KG embeddings due to the contribution of each associated image to entity is different in diverse link scenarios. Moreover, treating each triple independently when learning entity embeddings leads to local structural and the whole graph information missing. To address these challenges, we propose a novel link aware fusion and aggregation based multimodal knowledge graph completion model named LAFA, which is composed of link aware fusion module and link aware aggregation module. The link aware fusion module alleviates noise of irrelevant visual information by calculating the importance between an entity and its associated images in different link scenarios, and fuses the visual and structural embeddings according to the importance through our proposed modality embedding fusion mechanism. The link aware aggregation module assigns neighbor structural information to a given central entity by calculating the importance between the entity and its neighbors, and aggregating the fused embeddings through linear combination according to the importance. Extensive experiments on standard datasets validate that LAFA can obtain state-of-the-art performance.
更多查看译文
关键词
DMKM: Mining of Visual, Multimedia & Multimodal Data,NLP: Language Grounding & Multi-modal NLP
AI 理解论文
溯源树
样例
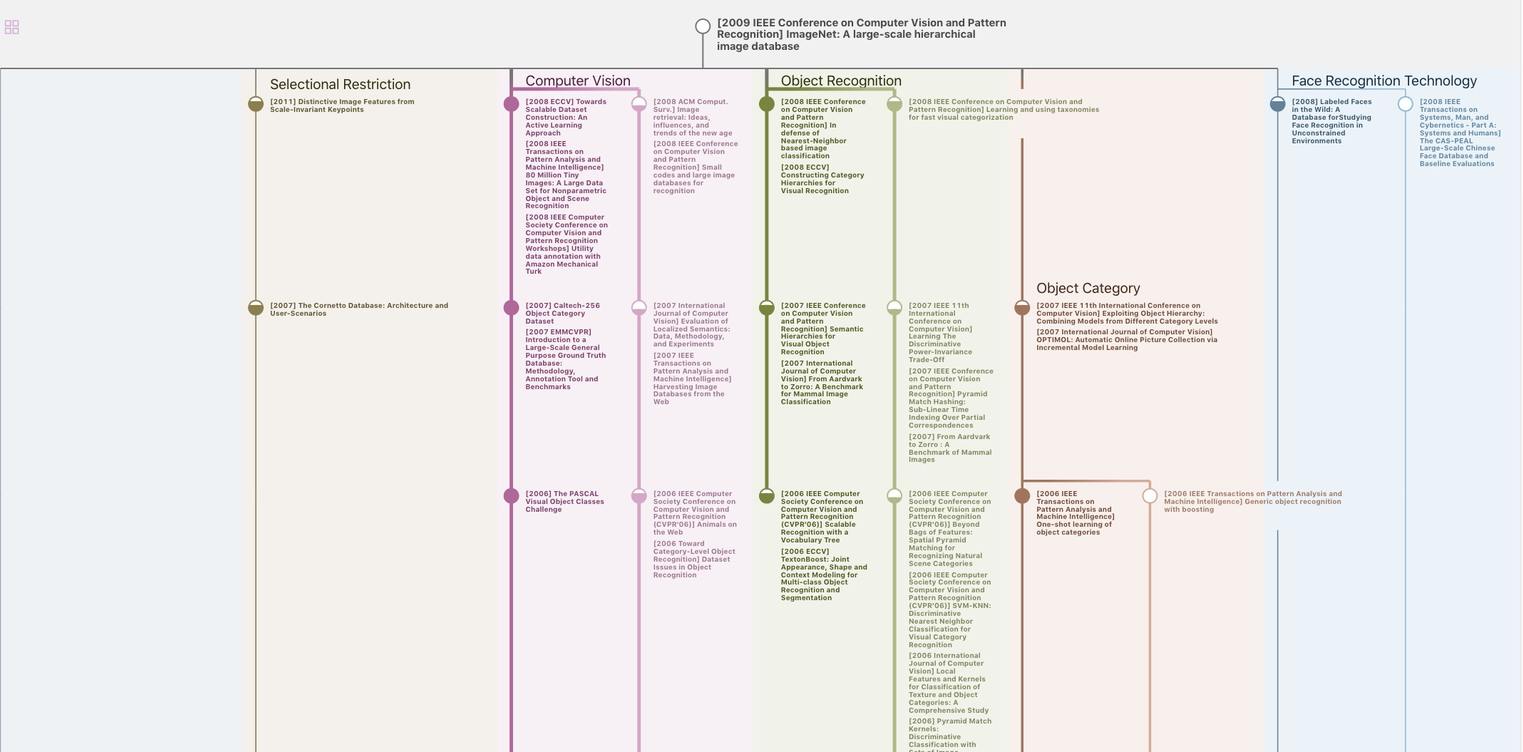
生成溯源树,研究论文发展脉络
Chat Paper
正在生成论文摘要