Cycle Self-Refinement for Multi-Source Domain Adaptation
AAAI 2024(2024)
摘要
Multi-source domain adaptation (MSDA) aims to transfer knowledge from multiple source domains to the unlabeled target domain. In this paper, we propose a cycle self-refinement domain adaptation method, which progressively attempts to learn the dominant transferable knowledge in each source domain in a cycle manner. Specifically, several source-specific networks and a domain-ensemble network are adopted in the proposed method. The source-specific networks are adopted to provide the dominant transferable knowledge in each source domain for instance-level ensemble on predictions of the samples in target domain. Then these samples with high-confidence ensemble predictions are adopted to refine the domain-ensemble network. Meanwhile, to guide each source-specific network to learn more dominant transferable knowledge, we force the features of the target domain from the domain-ensemble network and the features of each source domain from the corresponding source-specific network to be aligned with their predictions from the corresponding networks. Thus the adaptation ability of source-specific networks and the domain-ensemble network can be improved progressively. Extensive experiments on Office-31, Office-Home and DomainNet show that the proposed method outperforms the state-of-the-art methods for most tasks.
更多查看译文
关键词
ML: Transfer, Domain Adaptation, Multi-Task Learning,CV: Representation Learning for Vision
AI 理解论文
溯源树
样例
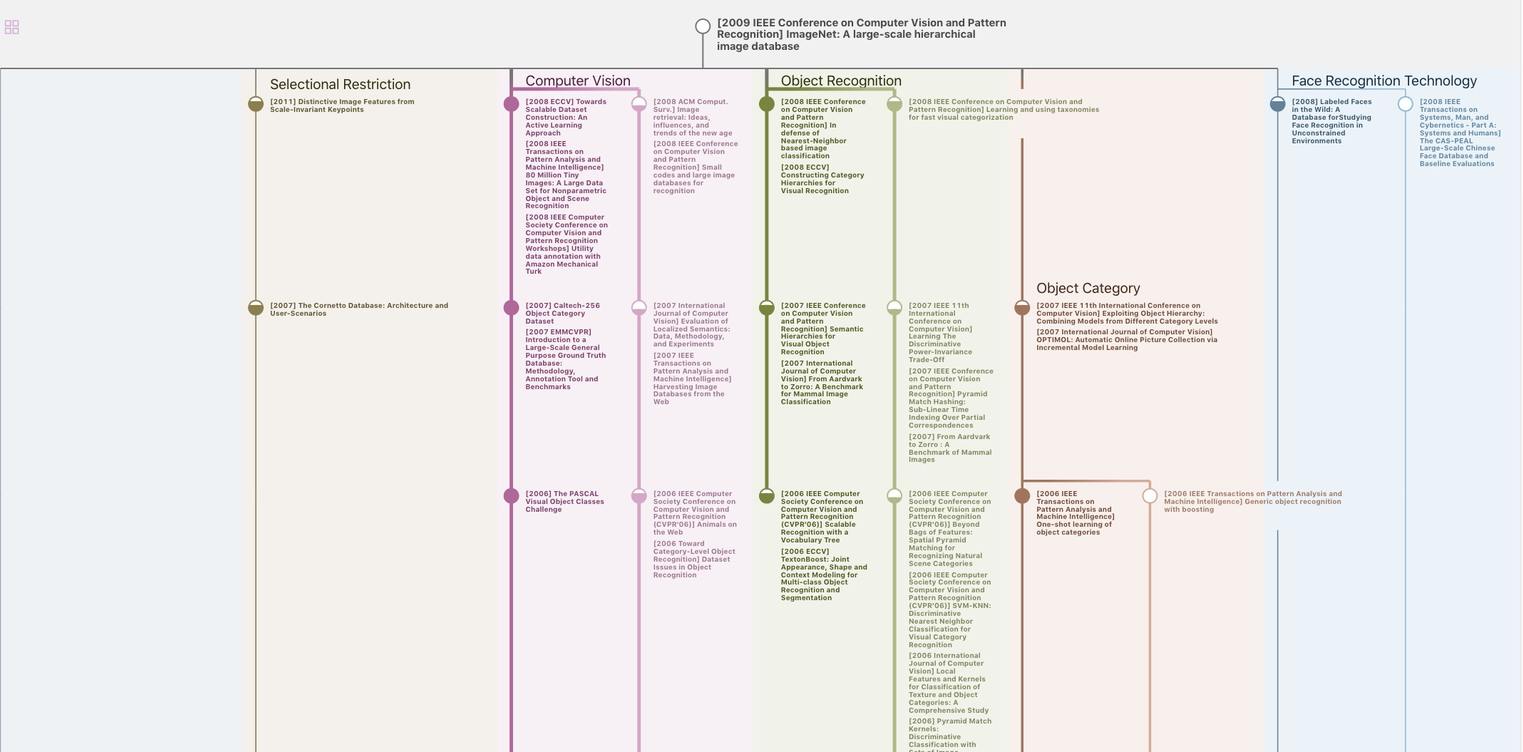
生成溯源树,研究论文发展脉络
Chat Paper
正在生成论文摘要