Towards Multi-Mode Outlier Robust Tensor Ring Decomposition
AAAI 2024(2024)
摘要
Conventional Outlier Robust Tensor Decomposition (ORTD) approaches generally represent sparse outlier corruption within a specific mode. However, such an assumption, which may hold for matrices, proves inadequate when applied to high-order tensors. In the tensor domain, the outliers are prone to be corrupted in multiple modes simultaneously. Addressing this limitation, this study proposes a novel ORTD approach by recovering low-rank tensors contaminated by outliers spanning multiple modes. In particular, we conceptualize outliers within high-order tensors as latent tensor group sparsity by decomposing the corrupted tensor into a sum of multiple latent components, where each latent component is exclusive to outliers within a particular direction. Thus, it can effectively mitigate the outlier corruptions prevalent in high-order tensors across multiple modes. To theoretically guarantee recovery performance, we rigorously analyze a non-asymptotic upper bound of the estimation error for the proposed ORTD approach. In the optimization process, we develop an efficient alternate direction method of multipliers (ADMM) algorithm. Empirical validation of the approach's efficacy is undertaken through comprehensive experimentation.
更多查看译文
关键词
ML: Matrix & Tensor Methods,ML: Multimodal Learning
AI 理解论文
溯源树
样例
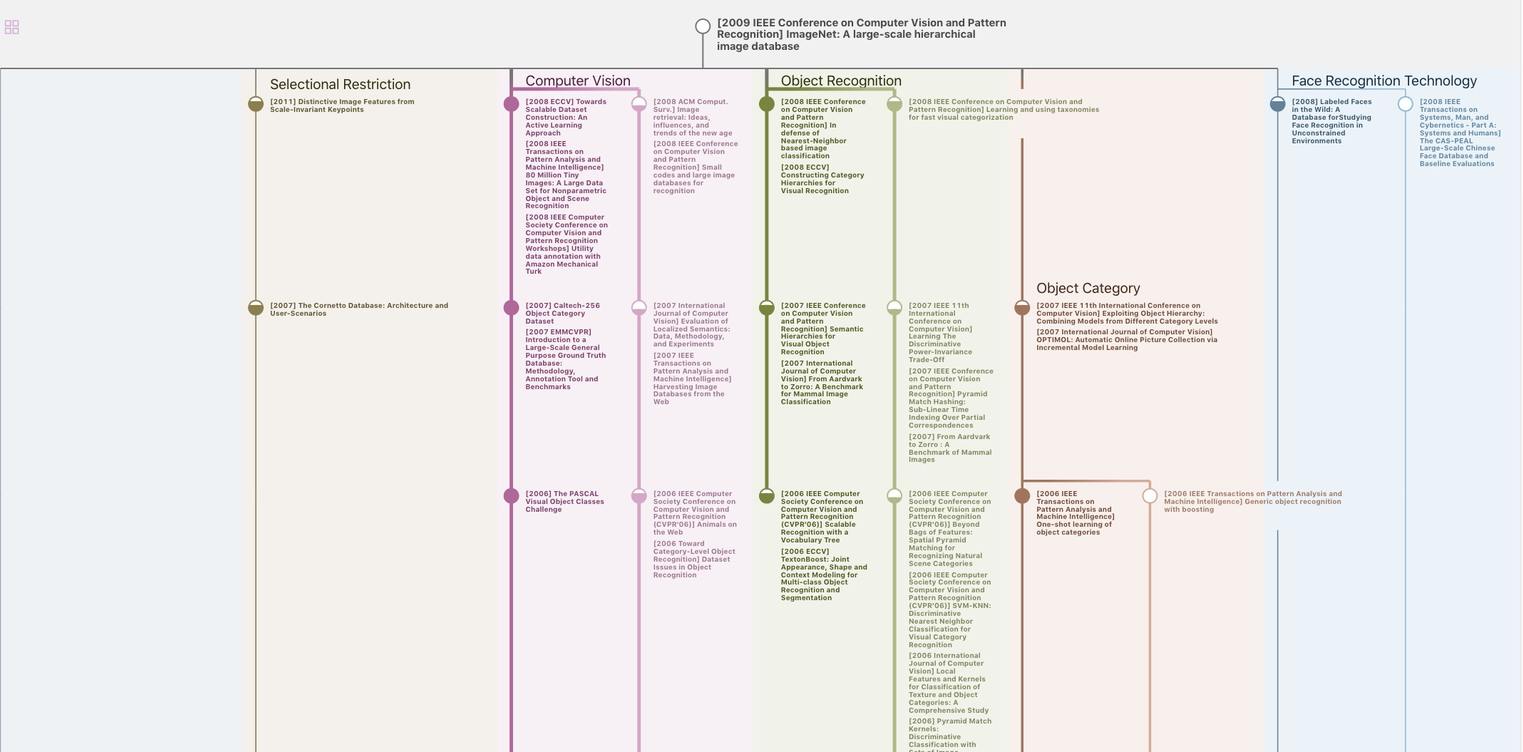
生成溯源树,研究论文发展脉络
Chat Paper
正在生成论文摘要