Towards Continual Knowledge Graph Embedding via Incremental Distillation
AAAI 2024(2024)
摘要
Traditional knowledge graph embedding (KGE) methods typically require preserving the entire knowledge graph (KG) with significant training costs when new knowledge emerges. To address this issue, the continual knowledge graph embedding (CKGE) task has been proposed to train the KGE model by learning emerging knowledge efficiently while simultaneously preserving decent old knowledge. However, the explicit graph structure in KGs, which is critical for the above goal, has been heavily ignored by existing CKGE methods. On the one hand, existing methods usually learn new triples in a random order, destroying the inner structure of new KGs. On the other hand, old triples are preserved with equal priority, failing to alleviate catastrophic forgetting effectively. In this paper, we propose a competitive method for CKGE based on incremental distillation (IncDE), which considers the full use of the explicit graph structure in KGs. First, to optimize the learning order, we introduce a hierarchical strategy, ranking new triples for layer-by-layer learning. By employing the inter- and intra-hierarchical orders together, new triples are grouped into layers based on the graph structure features. Secondly, to preserve the old knowledge effectively, we devise a novel incremental distillation mechanism, which facilitates the seamless transfer of entity representations from the previous layer to the next one, promoting old knowledge preservation. Finally, we adopt a two-stage training paradigm to avoid the over-corruption of old knowledge influenced by under-trained new knowledge. Experimental results demonstrate the superiority of IncDE over state-of-the-art baselines. Notably, the incremental distillation mechanism contributes to improvements of 0.2%-6.5% in the mean reciprocal rank (MRR) score. More exploratory experiments validate the effectiveness of IncDE in proficiently learning new knowledge while preserving old knowledge across all time steps.
更多查看译文
关键词
DMKM: Linked Open Data, Knowledge Graphs & KB Completio
AI 理解论文
溯源树
样例
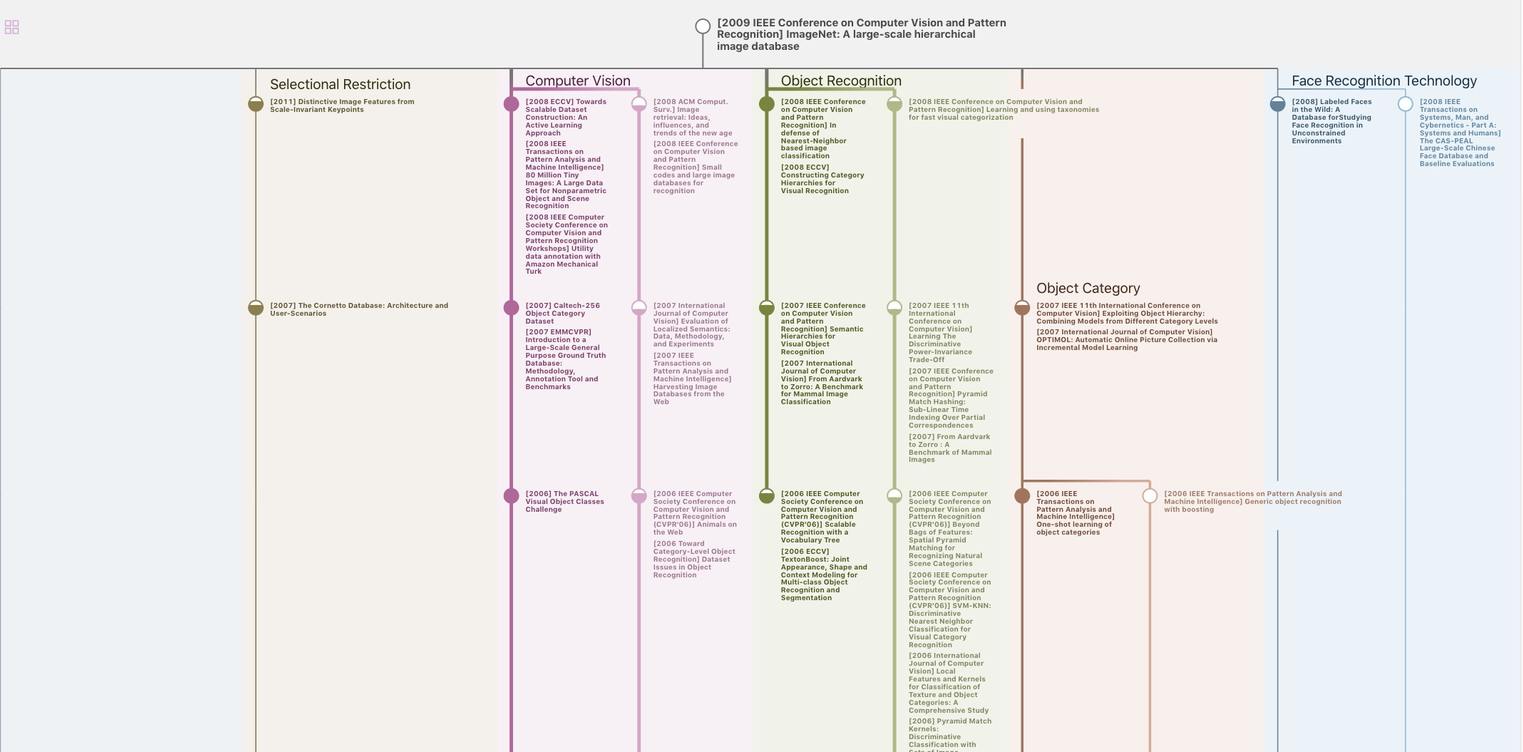
生成溯源树,研究论文发展脉络
Chat Paper
正在生成论文摘要