PIONEER: Highly Efficient and Accurate Hyperdimensional Computing using Learned Projection
2024 29th Asia and South Pacific Design Automation Conference (ASP-DAC)(2024)
摘要
Hyperdimensional Computing (HDC) has emerged as a lightweight learning paradigm garnering considerable attention in the IoT domain. Despite its appeal, HDC has lagged behind more intricate Machine Learning (ML) algorithms in accuracy, prompting prior research to propose sophisticated encoding and training techniques at the expense of efficiency. In this study, we present a novel approach for selecting projection vectors, used to encode input data into high-dimensional spaces, to enable HDC to attain high accuracy with significantly reduced vector sizes. We adopt a neural network-based mechanism to learn the projection vectors, and demonstrate their efficacy when integrated into a conventional HDC system. Furthermore, we introduce a novel sparsity technique to enhance hardware efficiency by compressing projection vectors and reducing computational operations with minimal impact on accuracy. Our experimental results reveal that at larger vector dimensions (e.g., 10k), our method (PIONEER), leveraging INT4 or binary vectors, outperforms the state-of-the-art high-precision nonlinear encoding in terms of accuracy, while preserving noteworthy accuracy even at extremely lower dimensions of 50–100. Additionally, by applying our proposed sparsification technique, PIONEER achieves significant performance and energy efficiency compared to previous work.
更多查看译文
关键词
Projection Learning,Hyperdimensional Computing,Learning Algorithms,Energy Efficiency,Internet Of Things,Dimensional Vector,Vector Of Size,Artificial Neural Network,Hidden Layer,Row Vector,Matrix Multiplication,Sparse Matrix,Random Vector,Element Of Vector,Projection Matrix,Binary Matrix,Nonzero Elements,Sign Function,Class Weights,Constant Vector,Random Projection,Encoding Vector,Learning Vector,Class Vector,Encoding Processes,Large Vectors,Small Vectors,Neural Network,Column Index,Datapath
AI 理解论文
溯源树
样例
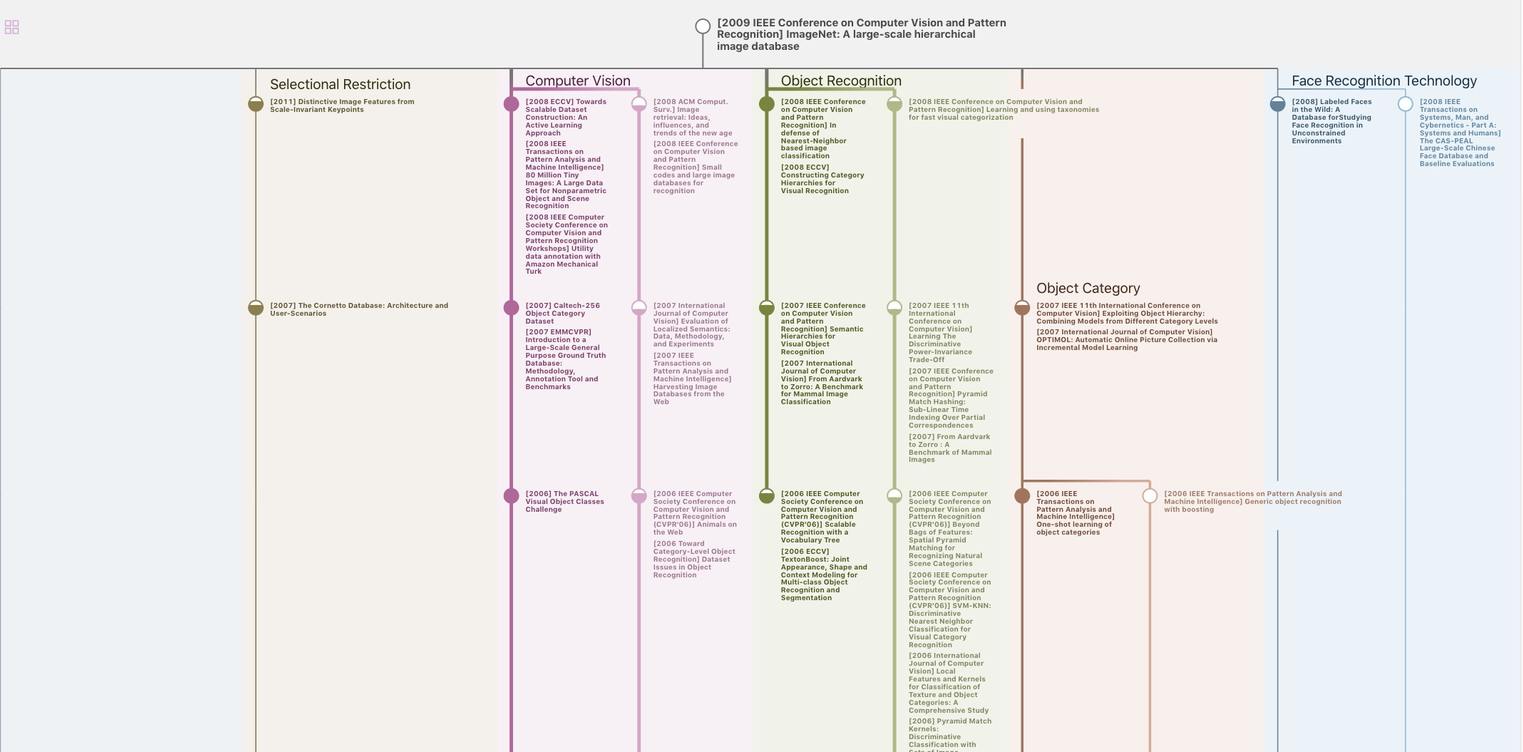
生成溯源树,研究论文发展脉络
Chat Paper
正在生成论文摘要