ZEBRA: A Zero-Bit Robust-Accumulation Compute-In-Memory Approach for Neural Network Acceleration Utilizing Different Bitwise Patterns
2024 29th Asia and South Pacific Design Automation Conference (ASP-DAC)(2024)
摘要
Deploying a lightweight quantized model in compute-in-memory (CIM) might result in significant accuracy degradation due to reduced signal-noise rate (SNR). To address this issue, this paper presents ZEBRA, a zero-bit robust-accumulation CIM approach, which utilizes bitwise zero patterns to compress computation with ultra-high resilience against noise due to circuit non-idealities, etc. First, ZEBRA provides a cross-level design that successfully exploits value-adaptive zero-bit patterns to improve the performance in robust 8-bit quantization dramatically. Second, ZEBRA presents a multi-level local computing unit circuit design to implement the bitwise sparsity pattern, which boosts the area/energy efficiency by 2x-4x compared with existing CIM works. Experiments demonstrate that ZEBRA can achieve <1.0% accuracy loss in CIFAR10/100 with typical noise, while conventional CIM works suffer from > 10% accuracy loss. Such robustness leads to much more stable accuracy for high-parallelism inference on large models in practice.
更多查看译文
关键词
Neural Network,Compute-in-Memory,Robustness Computing
AI 理解论文
溯源树
样例
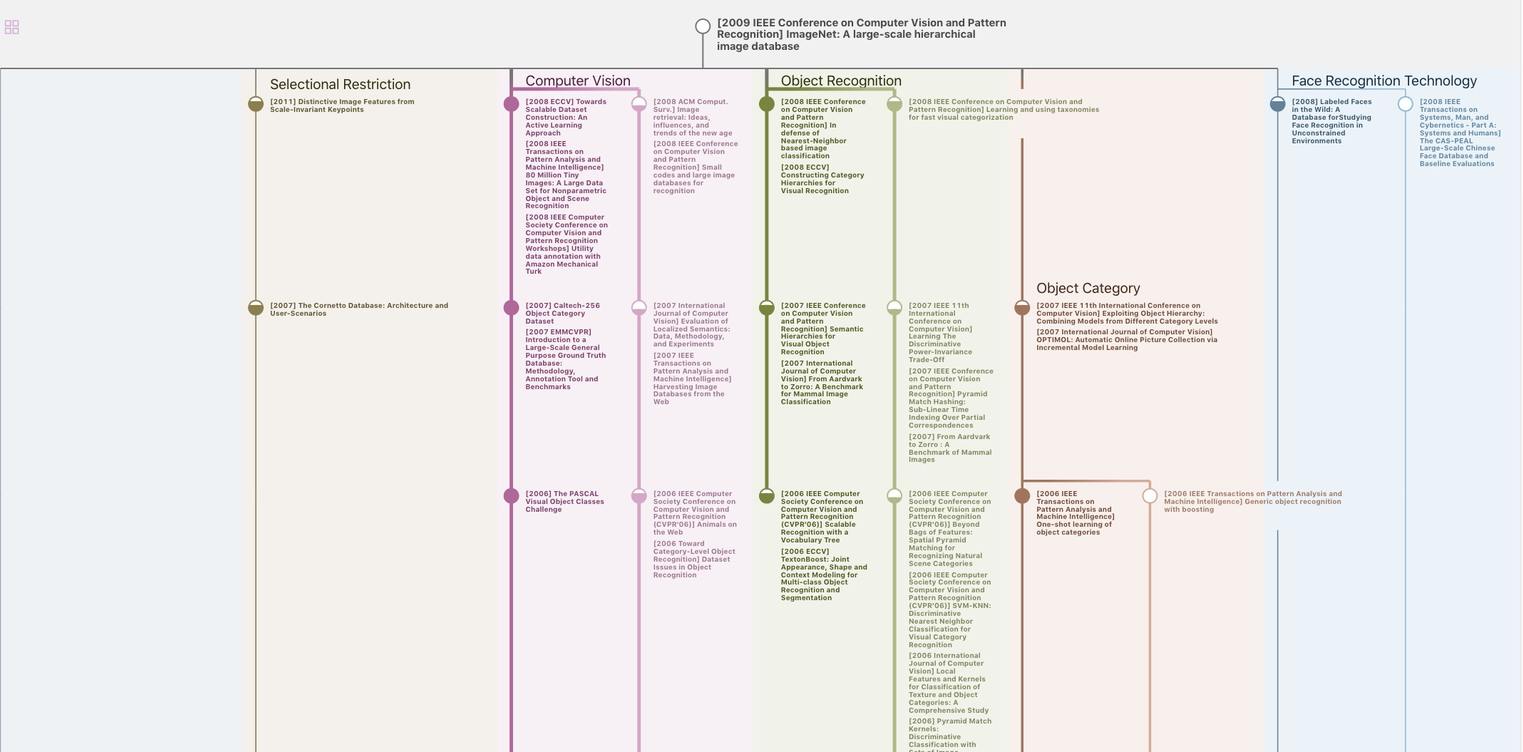
生成溯源树,研究论文发展脉络
Chat Paper
正在生成论文摘要