Detecting rotated ships in SAR images using a streamlined ship detection network and gliding phases
REMOTE SENSING LETTERS(2024)
摘要
Deep learning methods have greatly promoted the ship detection performance in synthetic aperture radar (SAR) images. However, due to the different imaging mechanisms and target characteristics, detecting ships in SAR imagery precisely and efficiently is still challenging. To address this issue, a streamlined rotational ship detection network (SRSD-Net) is proposed in this letter. First, we construct a lightweight network using one-level feature and a receptive field block (RFB) to extract ship features with rich context. Second, a simple and effective representation for rotated ship bounding boxes using the minimum external horizontal box and two phase factors is proposed. Finally, to reduce the missed detections of small ships, k-nearest label assignment (KNLA) is designed to make all targets equally contribute to the network training. Extensive experiments are conducted on high-resolution SAR images dataset (HRSID) and the results show that the proposed method can achieve high detection accuracy with low computational cost.
更多查看译文
关键词
Deep learning,lightweight model,rotated ship detection,synthetic aperture radar (SAR)
AI 理解论文
溯源树
样例
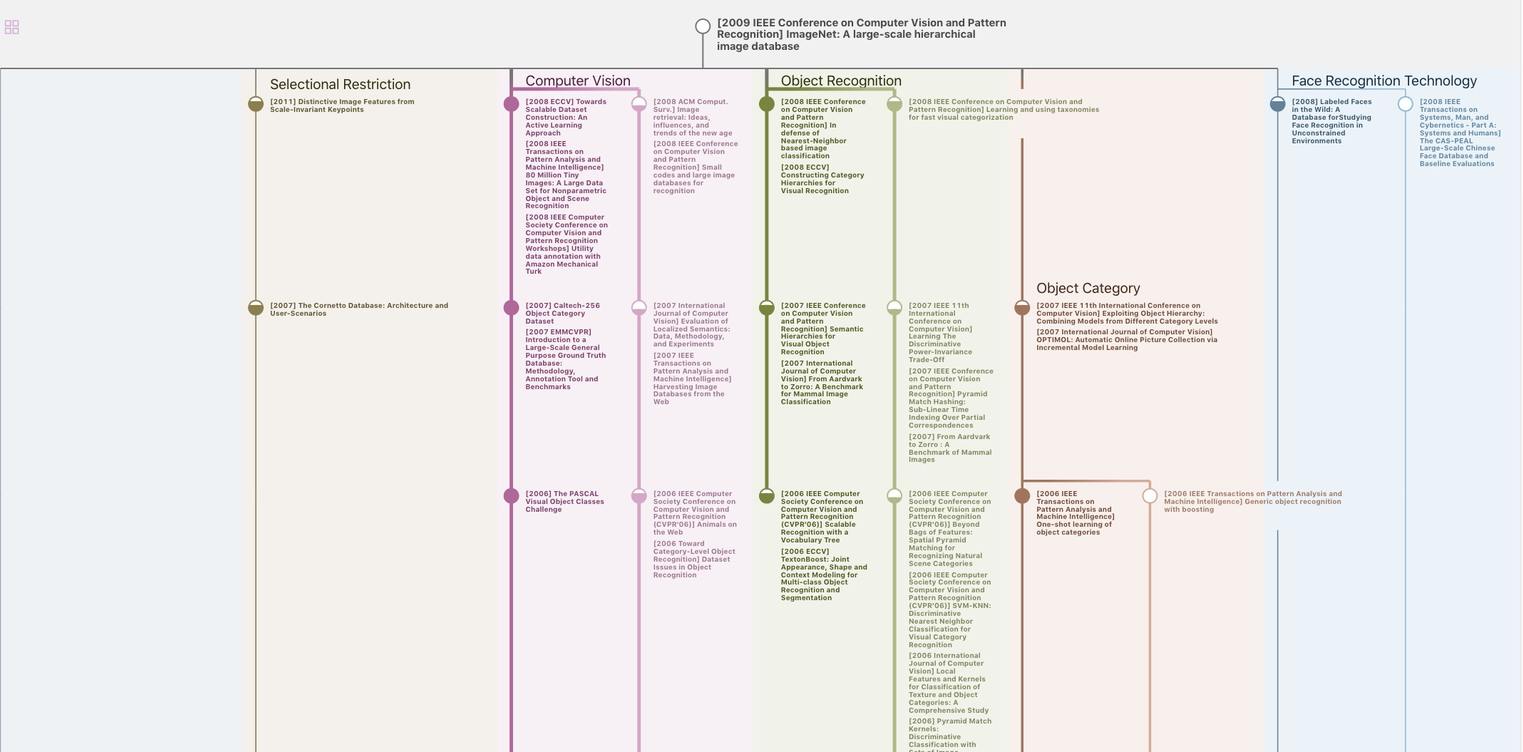
生成溯源树,研究论文发展脉络
Chat Paper
正在生成论文摘要