SkipDiff: Adaptive Skip Diffusion Model for High-Fidelity Perceptual Image Super-resolution
AAAI 2024(2024)
摘要
It is well-known that image quality assessment usually meets with the problem of perception-distortion (p-d) tradeoff. The existing deep image super-resolution (SR) methods either focus on high fidelity with pixel-level objectives or high perception with generative models. The emergence of diffusion model paves a fresh way for image restoration, which has the potential to offer a brand-new solution for p-d trade-off. We experimentally observed that the perceptual quality and distortion change in an opposite direction with the increase of sampling steps. In light of this property, we propose an adaptive skip diffusion model (SkipDiff), which aims to achieve
high-fidelity perceptual image SR with fewer sampling steps. Specifically, it decouples the sampling procedure into coarse skip approximation and fine skip refinement stages. A coarse-grained skip diffusion is first performed as a high-fidelity prior to obtaining a latent approximation of the full diffusion. Then, a fine-grained skip diffusion is followed to further refine the latent sample for promoting perception, where the fine time steps are adaptively learned by deep reinforcement learning. Meanwhile, this approach also enables faster sampling of diffusion model through skipping the intermediate denoising process to shorten the effective steps of the computation. Extensive experimental results show that our SkipDiff achieves superior perceptual quality with plausible reconstruction accuracy and a faster sampling speed.
更多查看译文
关键词
CV: Low Level & Physics-based Vision,ML: Deep Generative Models & Autoencoders
AI 理解论文
溯源树
样例
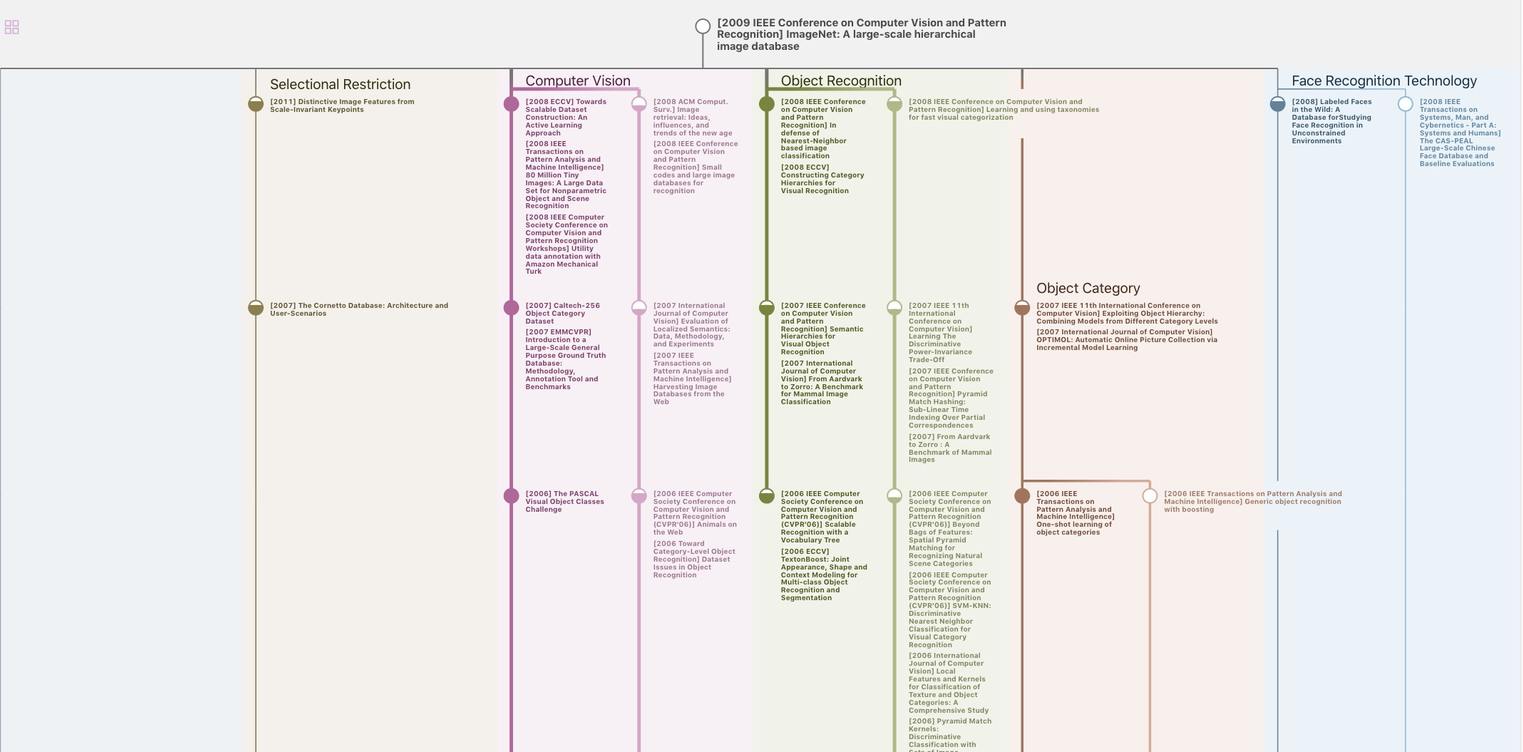
生成溯源树,研究论文发展脉络
Chat Paper
正在生成论文摘要