Inspecting Prediction Confidence for Detecting Black-Box Backdoor Attacks
AAAI 2024(2024)
摘要
Backdoor attacks have been shown to be a serious security threat against deep learning models, and various defenses have been proposed to detect whether a model is backdoored or not. However, as indicated by a recent black-box attack, existing defenses can be easily bypassed by implanting the backdoor in the frequency domain.
To this end, we propose a new defense DTInspector against black-box backdoor attacks, based on a new observation related to the prediction confidence of learning models. That is, to achieve a high attack success rate with a small amount of poisoned data, backdoor attacks usually render a model exhibiting statistically higher prediction confidences on the poisoned samples. We provide both theoretical and empirical evidence for the generality of this observation. DTInspector then carefully examines the prediction confidences of data samples, and decides the existence of backdoor using the shortcut nature of backdoor triggers. Extensive evaluations on six backdoor attacks, four datasets, and three advanced attacking types demonstrate the effectiveness of the proposed defense.
更多查看译文
关键词
APP: Security
AI 理解论文
溯源树
样例
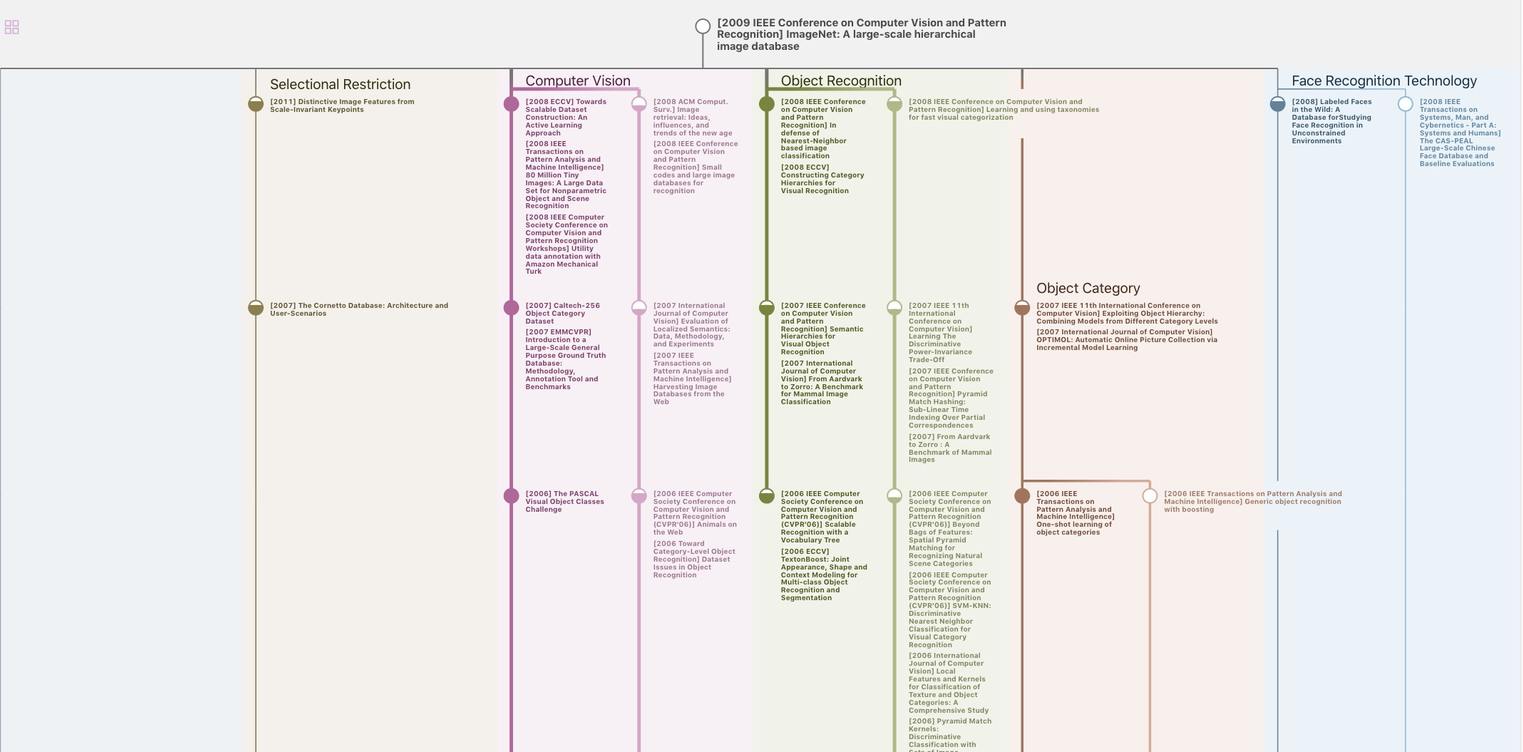
生成溯源树,研究论文发展脉络
Chat Paper
正在生成论文摘要