A General Theoretical Framework for Learning Smallest Interpretable Models
AAAI 2024(2024)
摘要
We develop a general algorithmic framework that allows us to obtain fixed-parameter tractability for computing smallest symbolic models that represent given data. Our framework applies to all ML model types that admit a certain extension property. By showing this extension property for decision trees, decision sets, decision lists, and binary decision diagrams, we obtain that minimizing these fundamental model types is fixed-parameter tractable. Our framework even applies to ensembles, which combine individual models by majority decision.
更多查看译文
关键词
KRR: Computational Complexity of Reasoning,ML: Transparent, Interpretable, Explainable ML
AI 理解论文
溯源树
样例
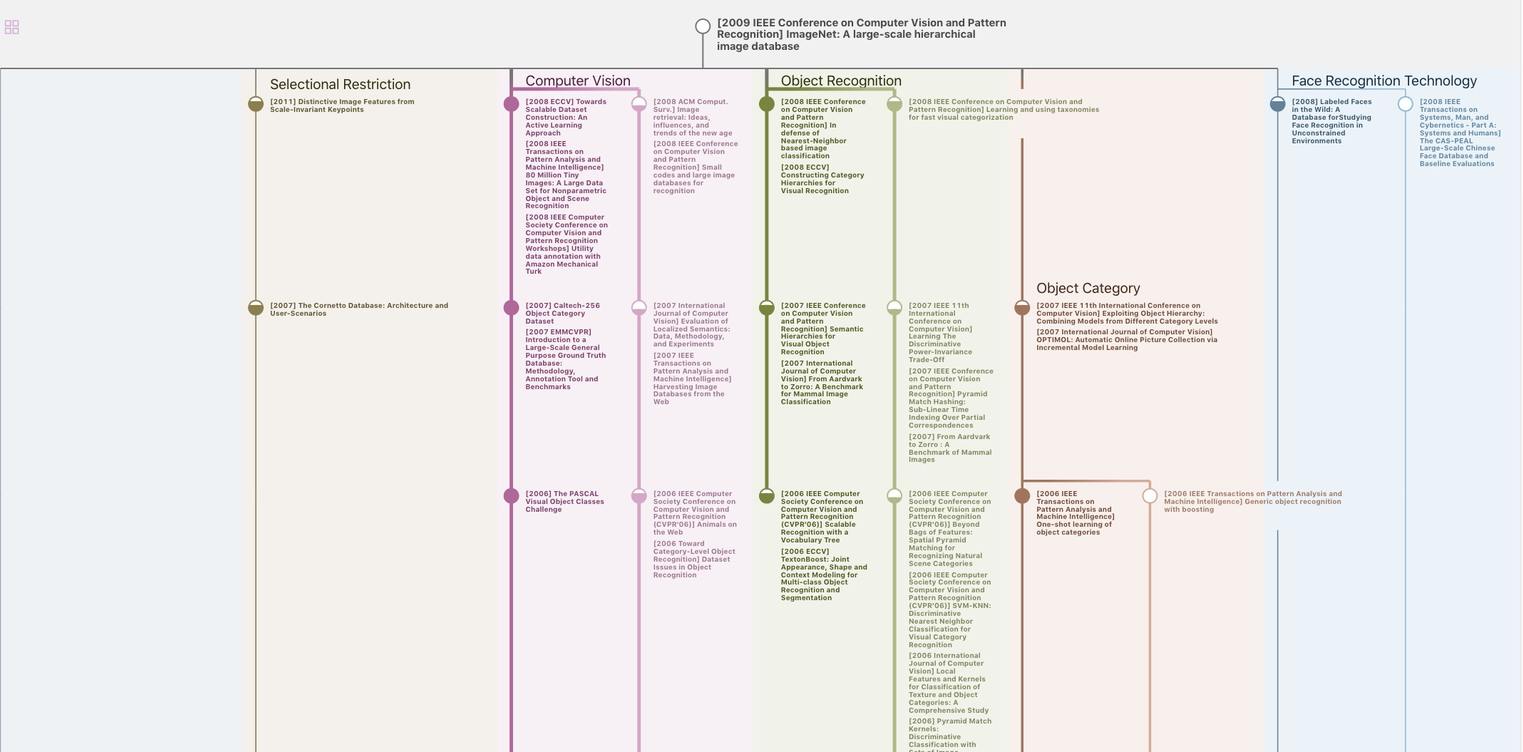
生成溯源树,研究论文发展脉络
Chat Paper
正在生成论文摘要