Review and Comparative Analysis of Deep Learning Techniques for Smart Grid Load Forecasting
2024 20th CSI International Symposium on Artificial Intelligence and Signal Processing (AISP)(2024)
摘要
In the last decade, the water and electricity industry has experienced significant investments in smart grid technologies. Within a smart grid framework, information and energy engage in bidirectional transmission, opening up diverse applications for artificial intelligence, including artificial neural networks, machine learning, and deep learning. This comprehensive review investigates the dynamic landscape of deep learning methodologies applied to load forecasting within smart grids, spanning short-term (STLF), medium-term (MILF), and long-term (LTLF) Forecasting horizons. We scrutinize a range of techniques, encompassing Auto-Encoder Method, Recurrent Neural Network (RNN), Long Short-Term Memory (LSTM), Convolutional Neural Network (CNN), Restricted Boltzmann Machine (RBM), Deep Belief Network (DBN), Deep Boltzmann Machine (DBM), Graph Neural Networks (GNNs), Attention Mechanisms, and Hybrid Models. This article introduces and reviews common deep-learning algorithms used in load forecasting for smart grids and power systems. It also offers a comparative assessment based on the reduction percentage in four indicators: accuracy, speed, mean absolute error (MAPE), and root mean square error (RMSE). The research aims to provide valuable insights into the strengths and weaknesses of each deep learning method, guiding researchers and practitioners in making informed decisions when selecting the most suitable approach for diverse load forecasting scenarios in smart grid environments.
更多查看译文
关键词
Load forecasting,Deep Learning,Machine learning,Smart grids,Short-term load forecasting (STLF),Medium-term load forecasting (MILF),Long-term load forecasting (LTLF),Artificial intelligence,Neural networks
AI 理解论文
溯源树
样例
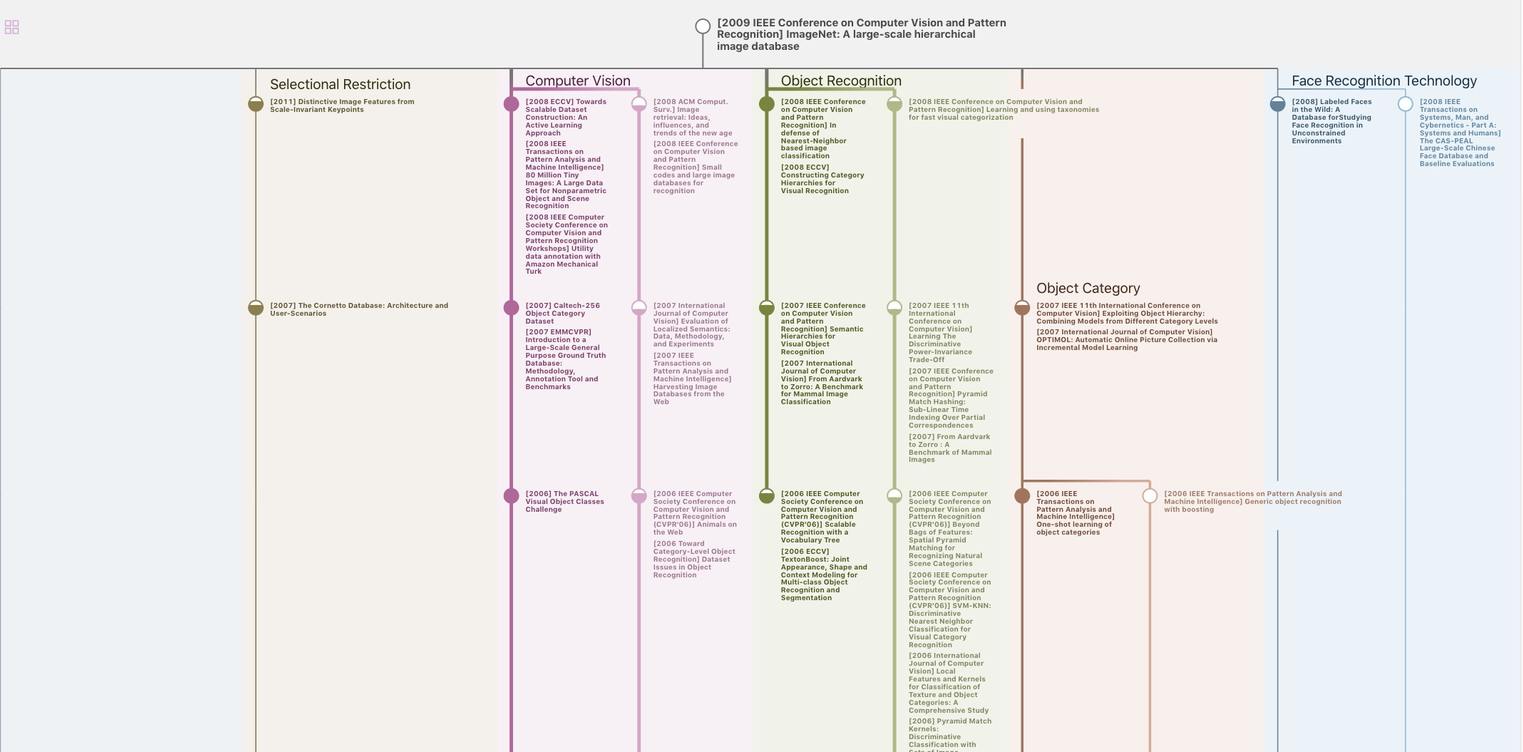
生成溯源树,研究论文发展脉络
Chat Paper
正在生成论文摘要