Symmetric Self-Paced Learning for Domain Generalization
AAAI 2024(2024)
摘要
Deep learning methods often suffer performance degradation due to domain shift, where discrepancies exist between training and testing data distributions.
Domain generalization mitigates this problem by leveraging information from multiple source domains to enhance model generalization capabilities for unseen domains.
However, existing domain generalization methods typically present examples to the model in a random manner, overlooking the potential benefits of structured data presentation.
To bridge this gap, we propose a novel learning strategy, Symmetric Self-Paced Learning (SSPL), for domain generalization.
SSPL consists of a Symmetric Self-Paced training scheduler and a Gradient-based Difficulty Measure (GDM).
Specifically, the proposed training scheduler initially focuses on easy examples, gradually shifting emphasis to harder examples as training progresses.
GDM dynamically evaluates example difficulty through the gradient magnitude with respect to the example itself.
Experiments across five popular benchmark datasets demonstrate the effectiveness of the proposed learning strategy.
更多查看译文
关键词
ML: Transfer, Domain Adaptation, Multi-Task Learning,ML: Deep Learning Algorithms
AI 理解论文
溯源树
样例
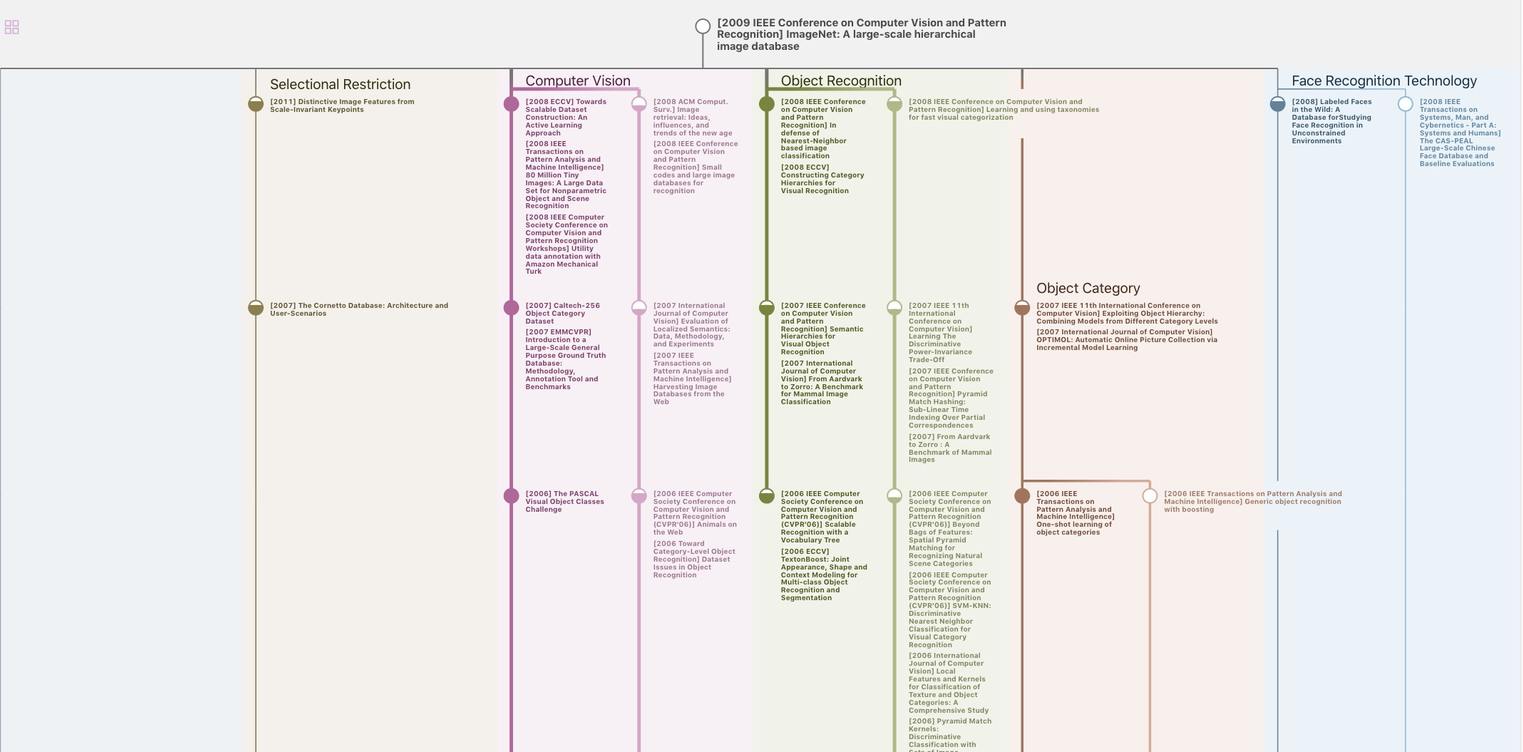
生成溯源树,研究论文发展脉络
Chat Paper
正在生成论文摘要