Scaling Offline Evaluation of Reinforcement Learning Agents through Abstraction
AAAI 2024(2024)
摘要
A critical challenge for the widescale adoption of reinforcement learning (RL) is the need to give domain experts assurance that learned policies will improve decision-making -- and not lead to unacceptable behavior. To meet this challenge, my work aims to develop new methods for offline policy evaluation in real world RL domains. There has been much recent interest in offline evaluation and many advances. However, recent benchmarking efforts have also shown that there remains a substantial gap between current state-of-the-art methods and real world domains such as robotics. Towards scalable offline evaluation, my group is investigating the use of methods for abstraction and representation learning. In this new faculty highlight, I will present our recent results that show the promise of this direction for scaling offline evaluation in RL domains. I will then describe future directions in this line of that work which will further realize the promise of offline policy evaluation for increasing confidence in deployed RL.
更多查看译文
关键词
Reinforcement Learning,Off-policy Evaluation,Abstraction
AI 理解论文
溯源树
样例
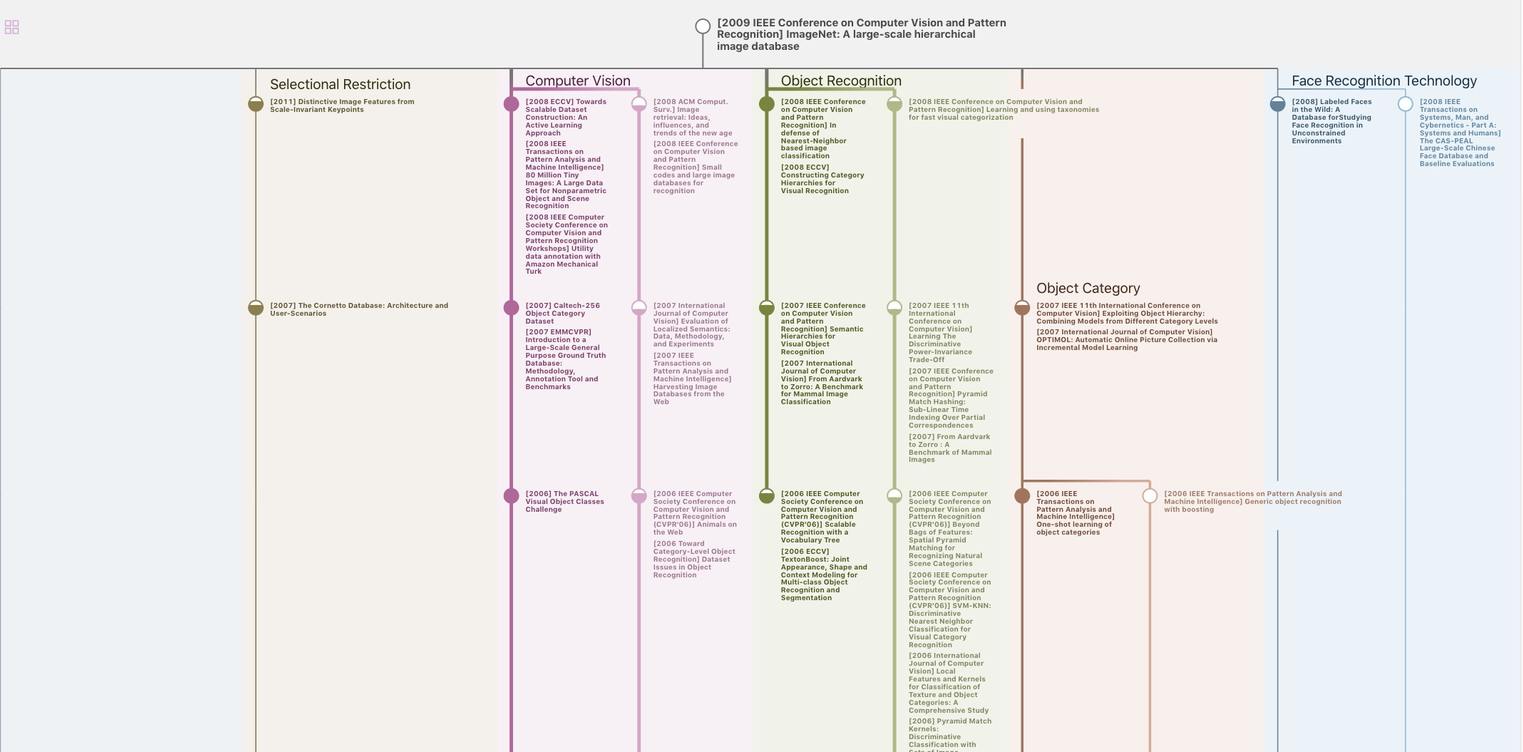
生成溯源树,研究论文发展脉络
Chat Paper
正在生成论文摘要