Non-exemplar Domain Incremental Object Detection via Learning Domain Bias
AAAI 2024(2024)
摘要
Domain incremental object detection (DIOD) aims to gradually learn a unified object detection model from a dataset stream composed of different domains, achieving good performance in all encountered domains. The most critical obstacle to this goal is the catastrophic forgetting problem, where the performance of the model improves rapidly in new domains but deteriorates sharply in old ones after a few sessions. To address this problem, we propose a non-exemplar DIOD method named learning domain bias (LDB), which learns domain bias independently at each new session, avoiding saving examples from old domains. Concretely, a base model is first obtained through training during session 1. Then, LDB freezes the weights of the base model and trains individual domain bias for each new incoming domain, adapting the base model to the distribution of new domains. At test time, since the domain ID is unknown, we propose a domain selector based on nearest mean classifier (NMC), which selects the most appropriate domain bias for a test image. Extensive experimental evaluations on two series of datasets demonstrate the effectiveness of the proposed LDB method in achieving high accuracy on new and old domain datasets. The code is available at https://github.com/SONGX1997/LDB.
更多查看译文
关键词
ML: Life-Long and Continual Learning,CV: Object Detection & Categorization
AI 理解论文
溯源树
样例
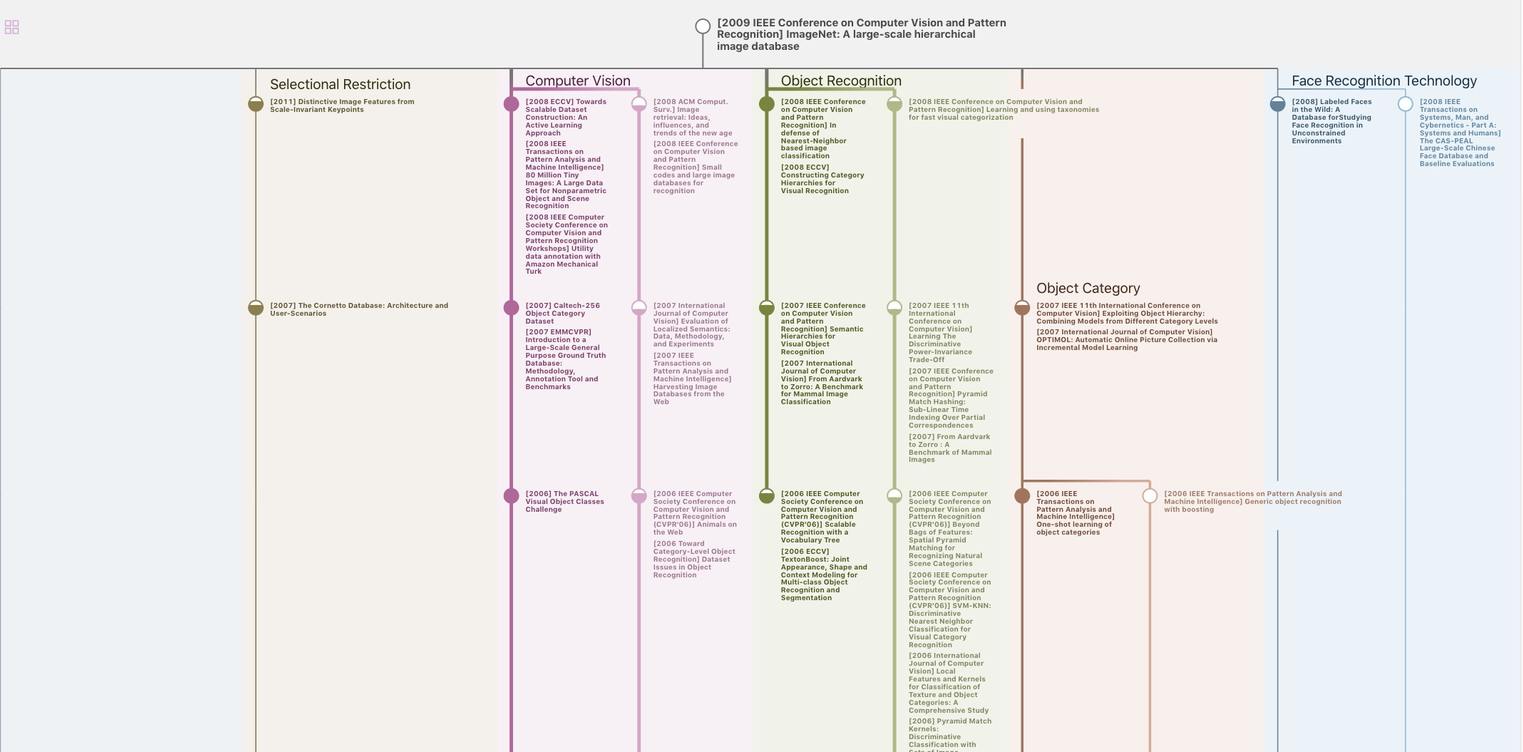
生成溯源树,研究论文发展脉络
Chat Paper
正在生成论文摘要