Discovering Agents (Abstract Reprint)
AAAI 2024(2024)
摘要
Causal models of agents have been used to analyse the safety aspects of machine learning systems. But identifying agents is non-trivial – often the causal model is just assumed by the modeller without much justification – and modelling failures can lead to mistakes in the safety analysis. This paper proposes the first formal causal definition of agents – roughly that agents are systems that would adapt their policy if their actions influenced the world in a different way. From this we derive the first causal discovery algorithm for discovering the presence of agents from empirical data, given a set of variables and under certain assumptions. We also provide algorithms for translating between causal models and game-theoretic influence diagrams. We demonstrate our approach by resolving some previous confusions caused by incorrect causal modelling of agents.
更多查看译文
关键词
Journal Track
AI 理解论文
溯源树
样例
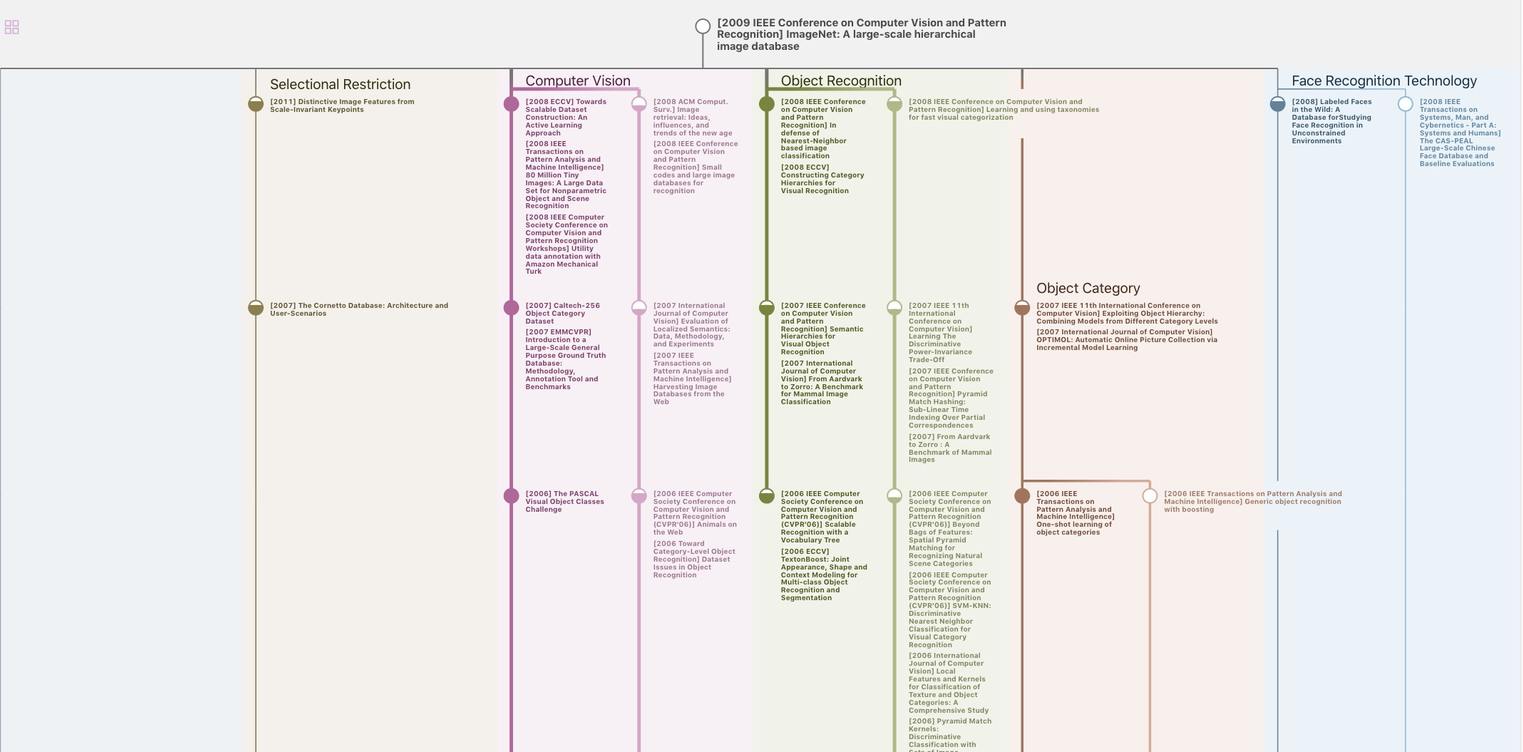
生成溯源树,研究论文发展脉络
Chat Paper
正在生成论文摘要