High-efficiency recovery of valuable metals from spent lithium-ion batteries: Optimization of SO2 pressure leaching and selective extraction of trace impurities
Journal of Environmental Management(2024)
摘要
The recovery of valuable metals from spent lithium-ion batteries (LIBs) is crucial for environmental protection and resource optimization. In the traditional recovery process of spent LIBs, the leaching of high-valence metals has the problems of high cost and limited reagent utilization, and some valuable metals are lost in the subsequent purification process of the leaching solution. To reduce the cost of reagents, this study proposes the use of low-cost SO2 as a reagent combined with pressure leaching to efficiently recover high-valence metals from delithiated materials of spent LIBs, while selective solvent extraction is used to remove trace impurities in the leaching solution to avoid the loss of valuable metals. Experimental results demonstrated that by optimizing the conditions to 0.25 MPa SO2 partial pressure and 60 min reaction time at 70 °C, the leaching efficiencies for Ni, Co, and Mn reached 99.6%, 99.3%, and 99.6%, respectively. The kinetic study indicated that the leaching process was diffusion-controlled. Furthermore, the delithiated materials were used to completely utilize the residual SO2 in the solution to obtain a high concentration Ni–Co–Mn rich solution. Subsequently, Fe and Al impurities were deeply removed through a synergistic extraction of Di-2-ethylhexyl phosphoric acid (D2EHPA) and tributyl phosphate (TBP) without loss of valuable metals, achieving a high-purity Ni–Co–Mn solution. The process developed based on this work has the characteristics of environmental friendliness, high valuable metal recovery, and high product purity, providing a reference technical method for the synergistic treatment of waste SO2 flue gas with spent LIBs and the deep purification of impurities in spent LIBs.
更多查看译文
关键词
Spent lithium-ion batteries,Sulfur dioxide,Pressure leaching,Solvent extraction
AI 理解论文
溯源树
样例
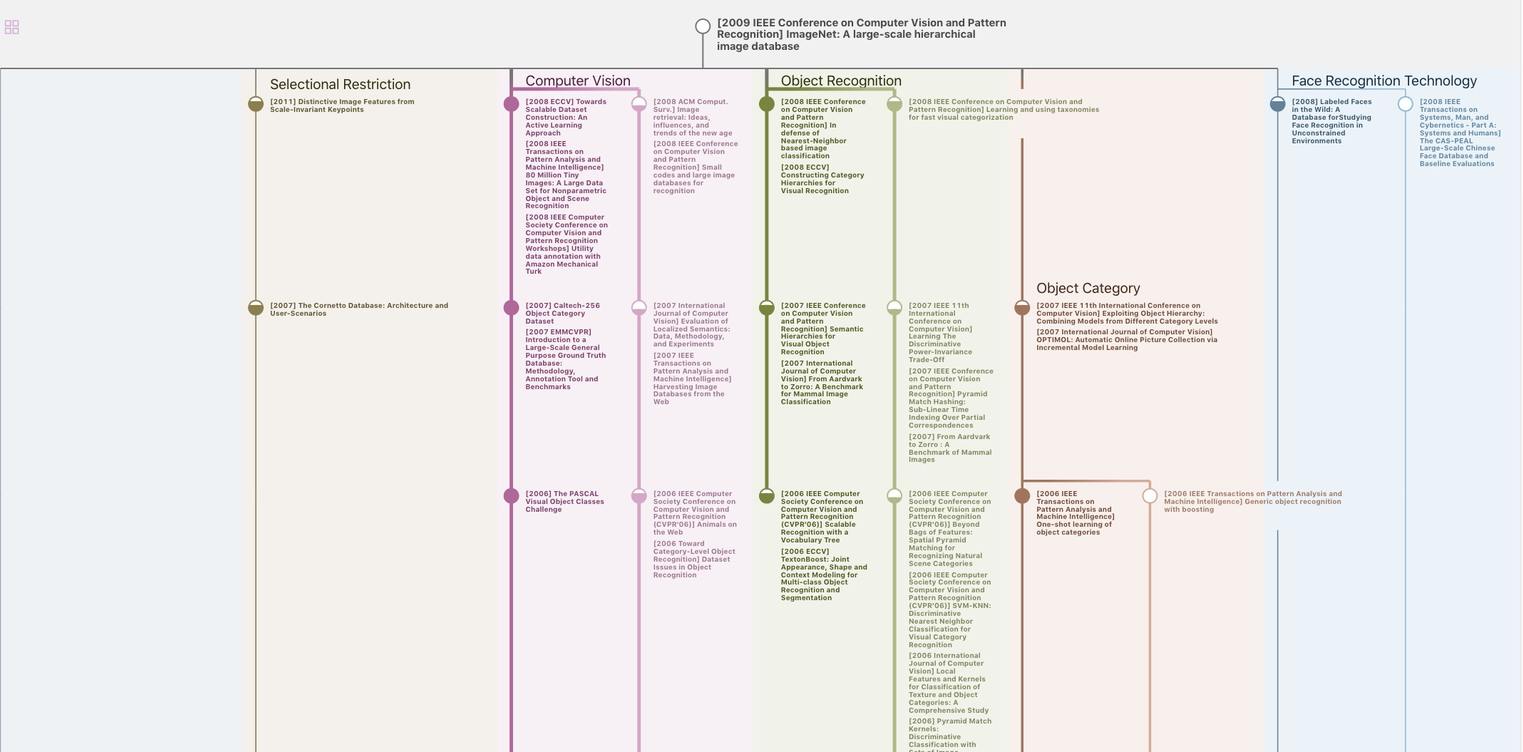
生成溯源树,研究论文发展脉络
Chat Paper
正在生成论文摘要