MACRO: Multi-agent Reinforcement Learning-based Cross-layer Optimization of Operational Amplifier
2024 29th Asia and South Pacific Design Automation Conference (ASP-DAC)(2024)
摘要
The optimization of operational amplifiers, including topology design and parameter tuning, is significantly challenging, given the high-dimensional and heterogeneous characteristics of the design space. This paper presents MACRO, a novel approach to operational amplifier design that employs multi-agent reinforcement learning for cross-layer optimization. We model the sequentially executed topology design and parameter tuning tasks as a Markov decision process, where the high-dimensional design space is effectively transformed into a series of manageable action spaces at each step. Two agents are meticulously tailored to specialize in these two distinct tasks respectively. The co-evolution of the agents is ensured by sharing design information and customizing the policy-gradient training method. Experimental results show that, compared with state-of-the-art methods, MACRO can produce superior-performing circuits while maintaining competitive design efficiency.
更多查看译文
关键词
operational amplifier,topology design,parameter tuning,multi-agent reinforcement learning
AI 理解论文
溯源树
样例
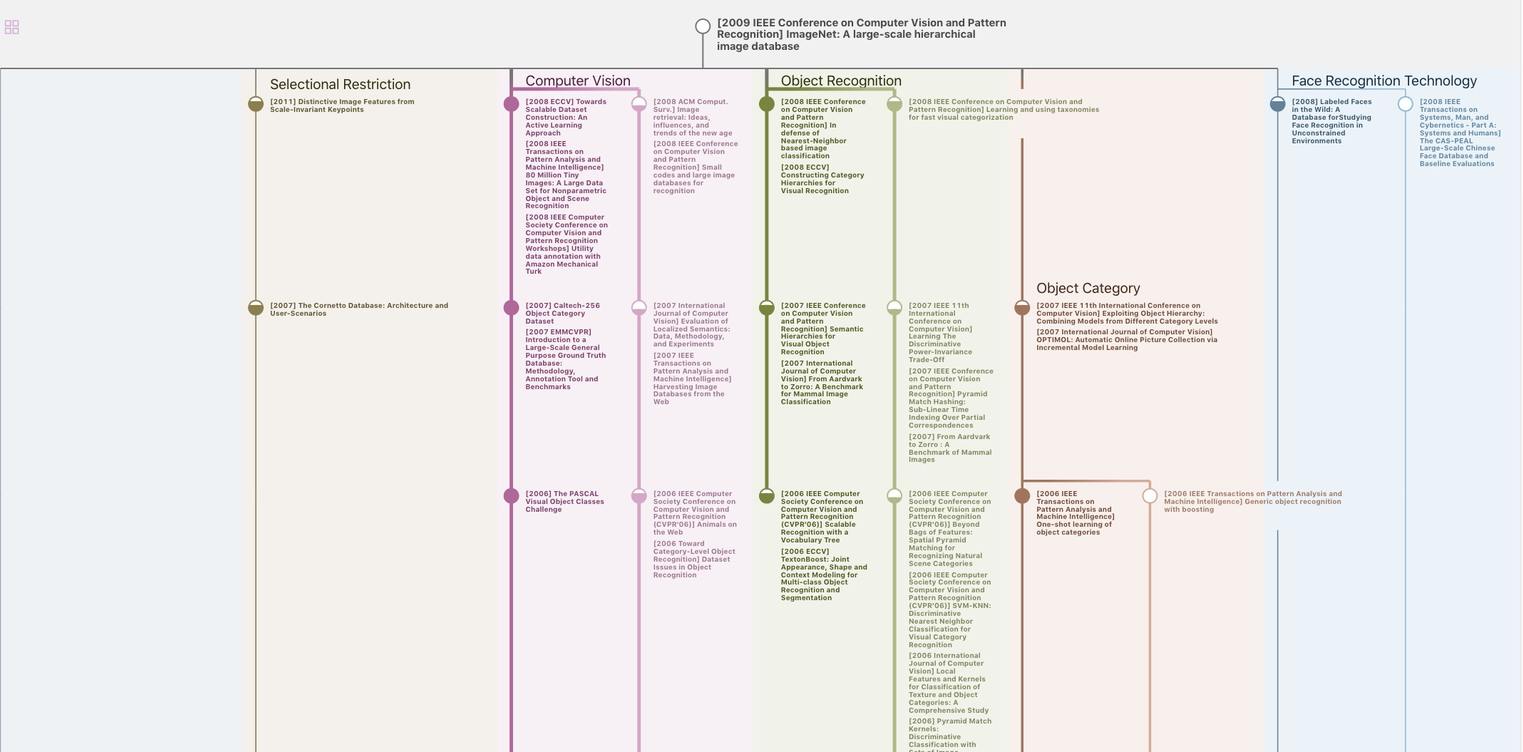
生成溯源树,研究论文发展脉络
Chat Paper
正在生成论文摘要