Automated synthesis of mixed-signal ML inference hardware under accuracy constraints
2024 29th Asia and South Pacific Design Automation Conference (ASP-DAC)(2024)
摘要
Due to the inherent error-tolerance of machine learning (ML) algorithms, many parts of the inference computation can be performed with adequate accuracy and low power under relatively low precision. Early approaches have used digital approximate computing methods to explore this space. Recent approaches using analog-based operations achieve power-efficient computation at moderate precision. This work proposes a mixed-signal optimization (MiSO) approach that optimally blends analog and digital computation for ML inference. Based on accuracy and power models, an integer linear programming formulation is used to optimize design metrics of analog/digital implementations. The efficacy of the method is demonstrated on multiple ML architectures.
更多查看译文
关键词
Machine Learning Inference,Inference Hardware,Low Precision,Approximate Computation,Digital Computer,Analog Computing,Integer Linear Programming Formulation,Machine Learning Architectures,Digital Technologies,Machine Learning Models,Thermal Noise,Energy Model,Set Of Operations,Noise Model,Data Conversion,Least Significant Bit,Digital Circuits,Noise Threshold,Quantization Noise,Digital Domain,Uniform Quantization,Digital Operations,Accuracy Trade-off,Analog Circuits,Digital Block,Analog Domain,Bitwise Operations,ResNet-18 Model,Intuitive Concept,Switching Energy
AI 理解论文
溯源树
样例
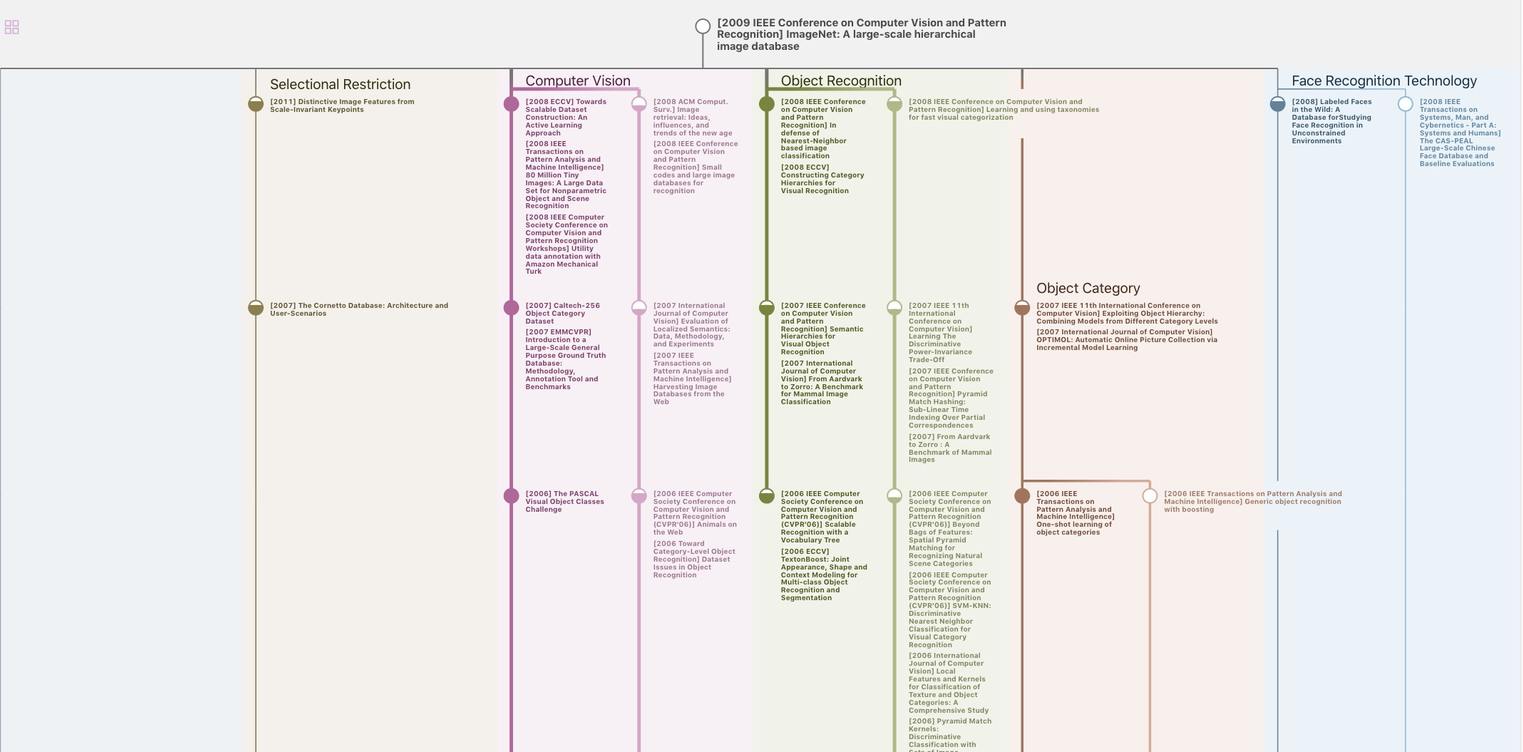
生成溯源树,研究论文发展脉络
Chat Paper
正在生成论文摘要