Guiding a Harsh-Environments Robust Detector via RAW Data Characteristic Mining
AAAI 2024(2024)
摘要
Consumer-grade cameras capture the RAW physical description of a scene and then process the image signals to obtain high-quality RGB images that are faithful to human visual perception. Conventionally, dense prediction scenes require high-precision recognition of objects in RGB images. However, predicting RGB data to exhibit the expected adaptability and robustness in harsh environments can be challenging. By capitalizing on the broader color gamut and higher bit depth offered by RAW data, in this paper, we demonstrate that RAW data can significantly improve the accuracy and robustness of object detectors in harsh environments. Firstly, we propose a general Pipeline for RAW Detection (PRD), along with a preprocessing strategy tailored to RAW data. Secondly, we design the RAW Corruption Benchmark (RCB) to address the dearth of benchmarks that reflect realistic scenarios in harsh environments. Thirdly, we demonstrate the significant improvement of RAW images in object detection for low-light and corrupt scenes. Specifically, our experiments indicate that PRD (using FCOS) outperforms RGB detection by 13.9mAP on LOD-Snow without generating restored images. Finally, we introduce a new nonlinear method called Functional Regularization (FR), which can effectively mine the unique characteristics of RAW data. The code is available at https://github.com/DreamerCCC/RawMining.
更多查看译文
关键词
CV: Representation Learning for Vision,CV: Other Foundations of Computer Vision,ML: Representation Learning
AI 理解论文
溯源树
样例
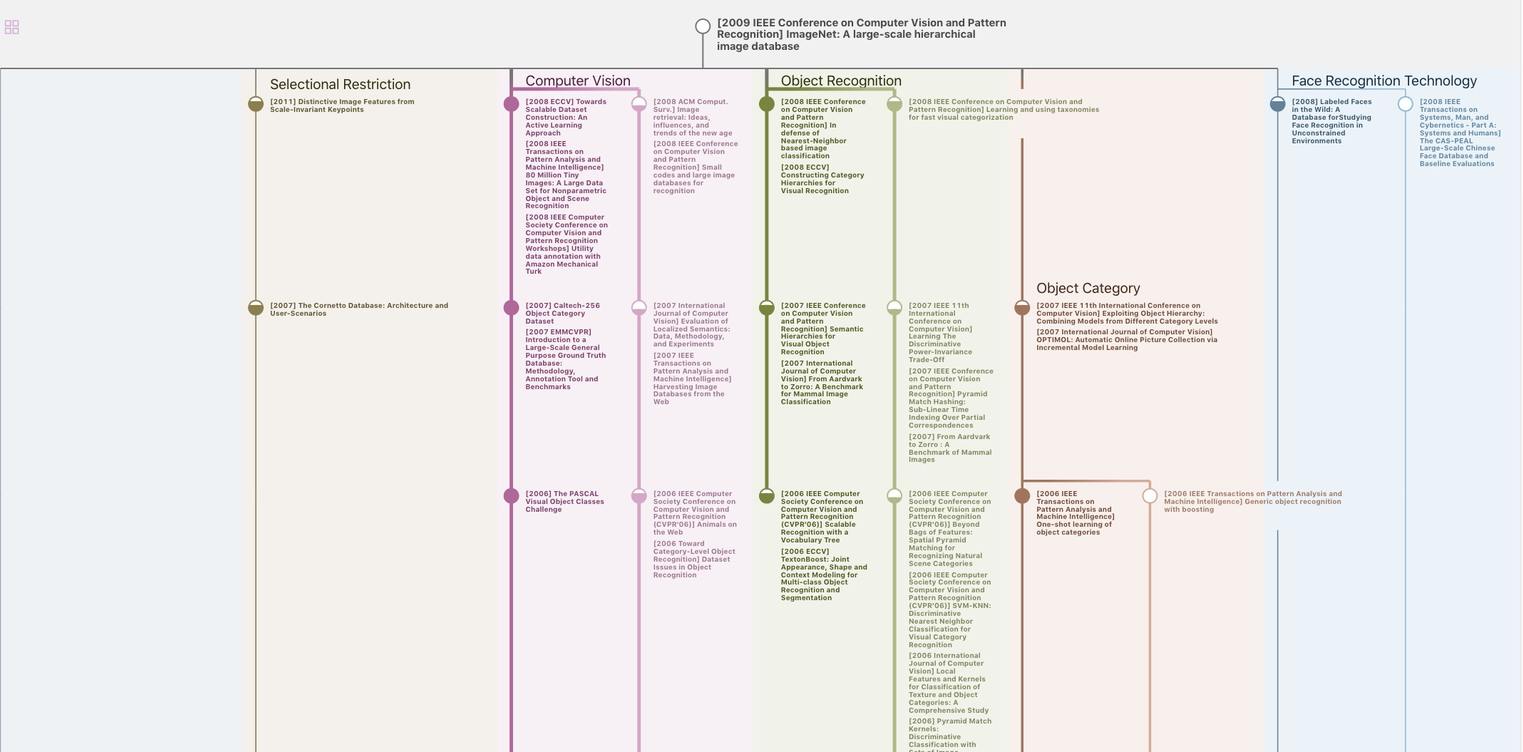
生成溯源树,研究论文发展脉络
Chat Paper
正在生成论文摘要