On Unsupervised Domain Adaptation: Pseudo Label Guided Mixup for Adversarial Prompt Tuning
AAAI 2024(2024)
摘要
To date, a backbone of methods for unsupervised domain adaptation (UDA) involves learning label-discriminative features via a label classifier and domain-invariant features through a domain discriminator in an adversarial scheme. However, these methods lack explicit control for aligning the source data and target data within the same label class, degrading the classifier's performance in the target domain. In this paper, we propose PL-Mix, a pseudo label guided Mixup method based on adversarial prompt tuning. Specifically, our PL-Mix facilitates class-dependent alignment and can alleviate the impact of noisy pseudo-labels. We then theoretically justify that PL-Mix can improve the generalization for UDA. Extensive experiments of the comparison with existing models also demonstrate the effectiveness of PL-Mix.
更多查看译文
关键词
NLP: Text Classification,ML: Transfer, Domain Adaptation, Multi-Task Learning
AI 理解论文
溯源树
样例
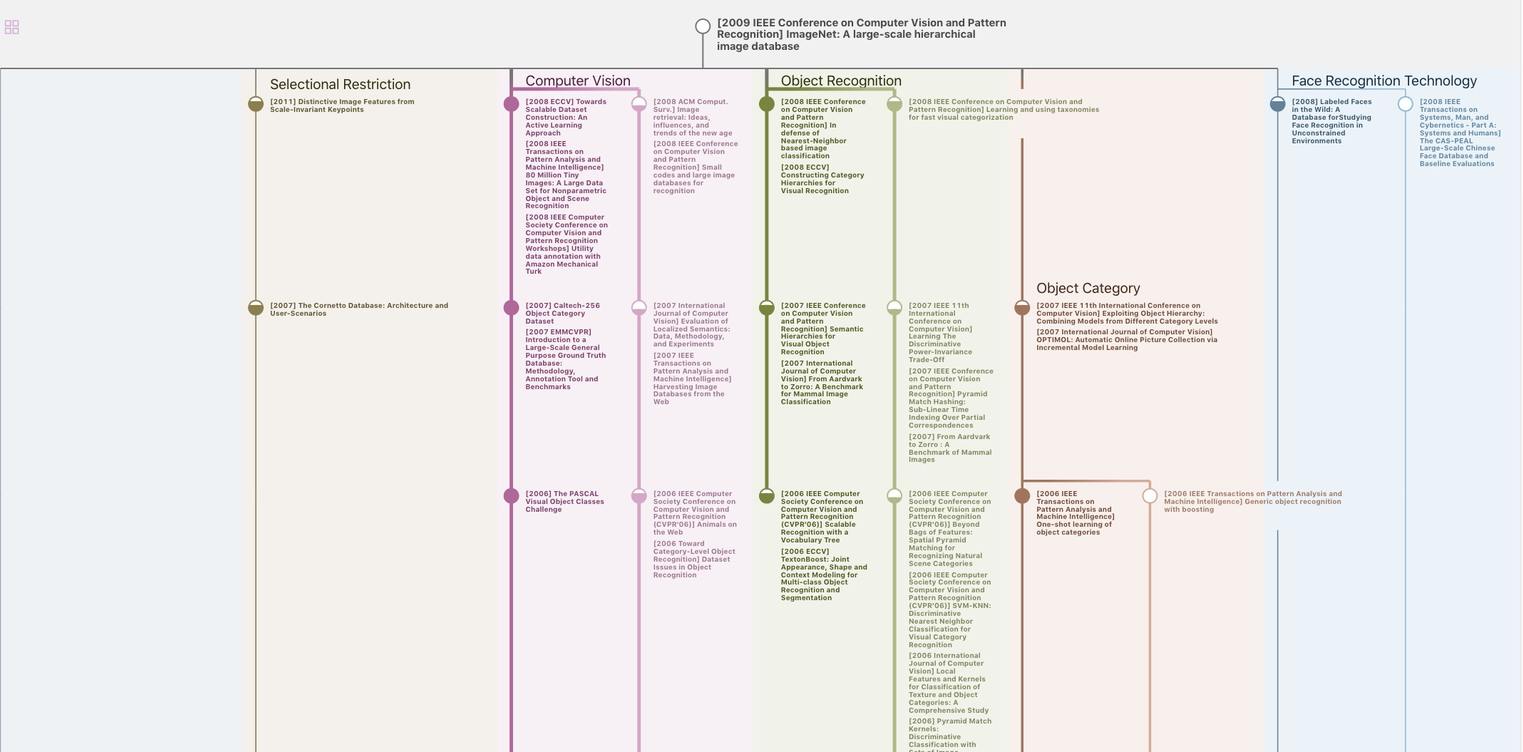
生成溯源树,研究论文发展脉络
Chat Paper
正在生成论文摘要